How the AI revolution can accelerate early drug discovery
Posted: 20 September 2023 | Dr Robert Scoffin (Cresset), Matthew Habgood (Cresset) | No comments yet
Rob Scoffin and Matthew Habgood from solutions provider Cresset look to the future of drug discovery and the roles that artificial intelligence and machine learning could play.
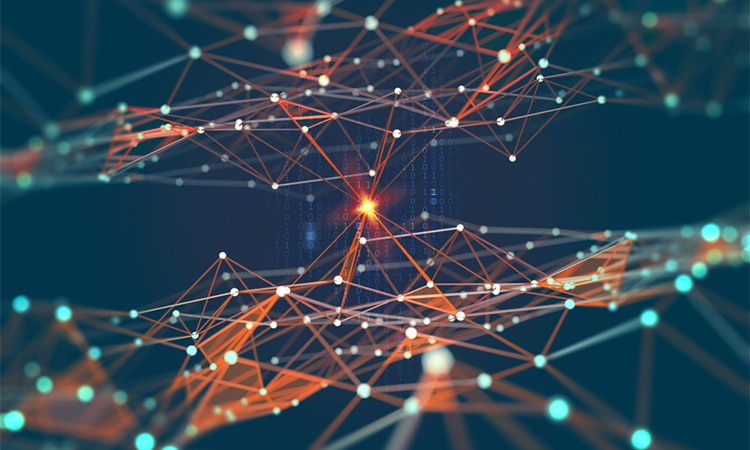
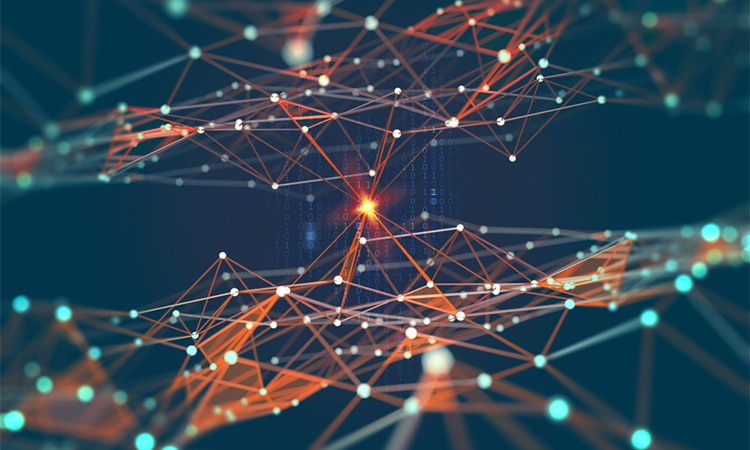
“AI will not replace drug discovery scientists, but drug discovery scientists who use AI will replace those who don’t” – comment during EFMC meeting 2018
Progressing a drug molecule from concept to commercialisation typically takes 10-15 years and has high associated costs of up to $2 billion per launched drug, if all failures are factored in.1 While many of these costs (most failures) occur within the development and clinical phases, the early discovery phase has high associated costs too.
This has led to a demand for the application of novel technologies to speed up and de-risk the discovery pipeline. Of current interest are artificial intelligence (AI) and machine learning (ML) approaches, which offer the ability to expand the chemical ‘search space’ for novel compounds, enable accelerated calculations of complex properties and provide insights into inherently noisy and incomplete information.
“In the future ALL DRUGS will be designed with AI” – AIDD company website
Here, we discuss whether and how AI and ML can accelerate delivery of a final drug candidate, while also examining why the above statement isn’t necessarily true.
AI and ML enhance efficiency in drug discovery
Selecting the best candidate molecule to progress from discovery to new chemical entity (NCE) is complex and time consuming. As a result, drug discovery teams are increasingly looking to improve the quality of their initial hit molecules to get a head start on this process. Drivers for this include that it is significantly harder to find novel molecules to bind to many drug targets of current interest, especially compared to twenty or thirty years ago. This has led to increased interest in evaluating many more molecules than previously, without significantly increasing wet chemistry and biology budgets.
AI and ML methods running on latest generation hardware can triage molecules at a scale that was unthinkable several years ago. Current practice contemplates ‘virtual library’ sizes of tens of billions of molecules; either in a ‘bottom up’ scenario where the library consists of molecules defined by a set of synthetic reactions and appropriate starting materials to form an ultra-large virtual library (ULVL), and/or a ‘top down’ scenario where the chemical space is defined by the assembly using a ‘generative AI’ of molecules from a ‘molecular soup’ of small chemical fragments. Each approach has strengths and weaknesses – generative AI, for instance, tends to suggest many molecules that are synthetically inaccessible or chemically unstable. Conversely, the ULVL contains predominantly synthetically accessible molecules, but has biased (and constrained) diversity, so does not explore as widely. In both cases, there is a large-scale computing challenge inherent in considering billions of options and reducing that down to a few tens to hundreds of molecules, which will then be made or acquired and tested against the biological target of interest.
“What I don’t understand is why my medicinal chemists don’t make all of the molecules I design” – AI scientist reflecting on why they hadn’t yet developed the ‘perfect’ molecule for their target
Although the filtering process, at least for early drug discovery, can be (or must be) highly automated, in the later stages, when nearing candidate selection, the process naturally switches to human decision making, albeit in the context of the multi-factorial data generated throughout the discovery project to-date. The nature of the AI ‘support’ to the human scientist must therefore alter throughout the discovery process.
How AI/ML technology has accelerated drug discovery
Although AI for drug discovery (AIDD) can be viewed as being in the initial phases of development and acceptance, compounds discovered using AIDD platforms are already entering clinical trials. The overall impact of AI and ML approaches is potentially very profound and can be seen at multiple levels. Aside from suggesting novel molecules in the front-end of a project, it can also accelerate the optimisation of molecules through the rapid calculation of complex properties and analysis of large-scale and inter-dependent data to enable candidate selection on a more robust and reproducible basis; for example:
Use of AI/ML in predicting results of complex calculations
Many complex calculations are completed throughout drug discovery to determine how a drug candidate behaves and identify if the properties of a molecule align with the desired profile for a drug to treat the disease being targeted. Training AI/ML tools to predict results of otherwise complex and time-consuming calculations is gaining traction in pharmaceutical R&D. These approaches require extensive datasets, with continuous input and output, to train the ML model for accurate predictions before use. ML techniques have been applied to:
- approximate the quantum mechanics (QM) of compound libraries. Accelerated and scaled analysis of the electronic contributions to the physical and chemical properties of a molecule – a single calculation wherein can take several hours using traditional QM codes – can be delivered with comparable results with a trained AI model in milliseconds.
- predict binding energies by free energy perturbation (FEP) calculations. FEP calculations are resource-intensive, but using AI methods to screen a library with FEP calculations accurately predicts the binding affinity and leads to results comparable to experimental measurements.
- protein folding for structural enablement. AI models can generate structures for targets that have not been determined experimentally. The path to using these in drug discovery has not been smooth, but as the sophistication of the tools and understanding of their output grows, AI-generated protein structures look likely to be increasingly adopted.
AI/ML has slashed screening times for ultra-large libraries
Using ML methods, an AI model can be built to screen molecules against a chosen drug target. Filtering through billions of potential drug candidates in quick succession, the AI/ML system can be trained to process ultra-large libraries of molecules to predict properties such as binding affinity. Operating at a scale that would not be feasible using traditional methods, this cost-effective approach significantly streamlines candidate analysis, removing molecules that lack the desired properties from the drug discovery study. This process can consider more compounds than would ever be possible in the lab and allows scientists to focus on the most promising contenders identified through virtual screening methods.
Generation of synthetic routes using AI
AI methods are extremely adept at pattern recognition in complex and noisy datasets. An early example of the use of AI in chemistry and drug discovery was the generation and optimisation of synthetic routes to molecules of interest.2 AI is trained using literature examples of synthetic reactions and is then able to rapidly search for viable synthesis routes to a set of compounds, as well as further optimise routes to give better yields or lower costs. In published studies the AI performed similarly to very experienced synthetic organic chemists when challenged to develop a route to a given set of test compounds, but was able to consistently find better (fewer steps/higher yields/lower feedstock costs, etc) routes in a far more reasonable time.3
Overcoming limitations to embrace the future of AI
AI/ML techniques have already made a significant impact on drug discovery, offering advanced solutions to funnel potential candidates through the pipeline at speed. Although AI has the potential to solve other limiting steps in drug discovery, application of AI/ML systems is restricted by the data available, as significant amounts of information is required to train an AI model. The nature of the drug discovery process necessarily requires the implementation of strict data protection and privacy, which does unfortunately limit data sharing.
To really benefit from AI, the pharmaceutical industry must be more open to data sharing. Larger amounts of available data would significantly increase the range of problems to which AI/ML tools can be applied. Complex molecular molecular properties in the areas of absorption, distribution, metabolism or excretion (ADME) could be better predicted, accurately filtering out poor candidates and streamlining discovery.
Another limitation to date has been the relative scarcity of experts who are proficient in both drug discovery and AI, whom are necessary to ensure that the tools will be utilised efficiently to deliver accurate insights and to inform and accelerate drug discovery.
The future of AI in drug discovery
While we don’t subscribe to the “one day all drug discovery will be done solely using AI” philosophy, it is clear that AI can positively impact challenges encountered throughout the drug discovery process. Current limitations to adoption hinge on training ML models, with restricted access to data and strict confidentiality in the pharmaceutical industry forming a barrier. Interest in utilising AI is on the rise, however, for applications including approximation of quantum mechanics, generation of optimal synthetic routes for compounds, predicting protein structures, accurate prediction of FEP calculations, and scaling of docking. As more data is generated, ML tools could be better positioned to address these complex calculations.
Integration of AI/ML into drug discovery platforms has already led to considerable improvements, with collective efforts accelerating drug discovery rates, enhancing efficiency and reducing costs. AI/ML systems will continue to evolve and shape the pharmaceutical industry, significantly enhancing scale and accelerating candidate selection.
Author bio:
Matthew Habgood
Principal Computational Chemist, Cresset
Matthew graduated from Imperial College London in 2004 with an MSci in Chemistry and was co-awardee of the Neil Arnott prize for best chemistry graduate at the University of London. He subsequently obtained an MSc in Mathematical Modelling and Scientific Computing from the University of Oxford.
From 2005 to 2008 he carried out a DPhil (PhD) at Oxford on nanomaterials for quantum computation, followed by postdoctoral work on the prediction of crystal structures at University College London.
Matthew subsequently put his computational chemistry skills to use in the defence sector. He then joined the pharmaceutical industry as a senior scientist at Evotec in 2016, working to develop drug candidates for a wide variety of internal and external clients. Following a second stint in defence, he joined Cresset in 2022, working to develop, source and evaluate new computational techniques for Cresset’s software.
Matthew is a chartered chemist. He has published 22 scientific articles and has a h-index of 13.
CEO and Chairman, Cresset
Robert is an expert in the fields of molecular modelling and cheminformatics. His DPhil is in chemistry from the University of Oxford. Rob is passionate about applying computational methods to help meet medical challenges. He believes drug discovery and design can be streamlined and improved through the use of computational methods, resulting in better drugs being brought to market sooner. Rob is a Fellow of the Royal Society of Chemistry.
Rob joined Cresset as CEO in 2010 and now also serves as Chairman of Cresset. During the time he has been leading the team, Cresset has further developed its software and contract research divisions from a very solid customer base, into a high-growth and profitable business. Rob also serves as Co-Chairman for Torx Software, a collaboration between Cresset and Elixir Software. Previous roles include CEO of Amedis and VP, Europe at CambridgeSoft.
References
- Austin D, Hayford T. Research and Development in the Pharmaceutical Industry | Congressional Budget Office [Internet]. Congressional Budget Office. 2021. Available from: https://www.cbo.gov/publication/57126
- Schneider G. Automating drug discovery. Nat Rev Drug Discov 17, 97–113 (2018). https://doi.org/10.1038/nrd.2017.232
- Paul D, Sanap G, Shenoy S, et al. Artificial intelligence in drug discovery and development. Drug discovery today, 26(1), 80–93 (2021). https://doi.org/10.1016/j.drudis.2020.10.010
Related topics
Artificial Intelligence, Drug Discovery, Machine learning
Related organisations
Cresset Discovery Services
Related people
Dr Robert Scoffin (Cresset), Matthew Habgood (Cresset)