How AI and LLMs are transforming drug discovery: part 2
Posted: 26 May 2025 | Dr Raminderpal Singh (Hitchhikers AI and 20/15 Visioneers) | No comments yet
As AI reshapes scientific work, two founders debate how best to build tools scientists can trust — should we embed expertise into the model or the team? From agent-powered labs to hypothesis-generating machines, the future of drug discovery is being reimagined right now.
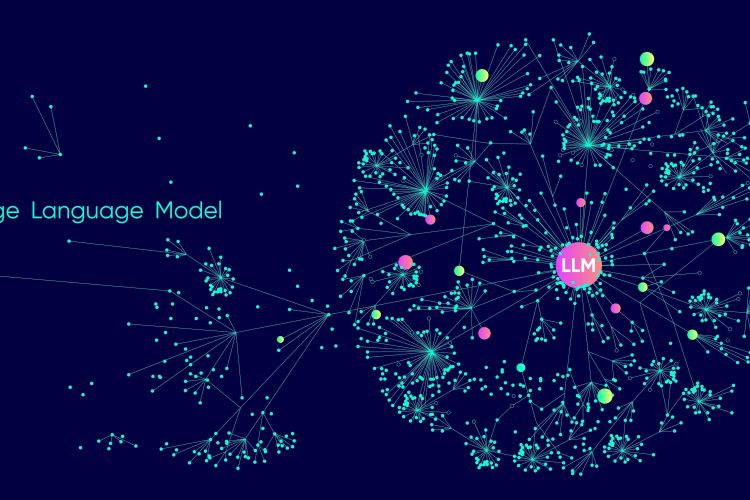
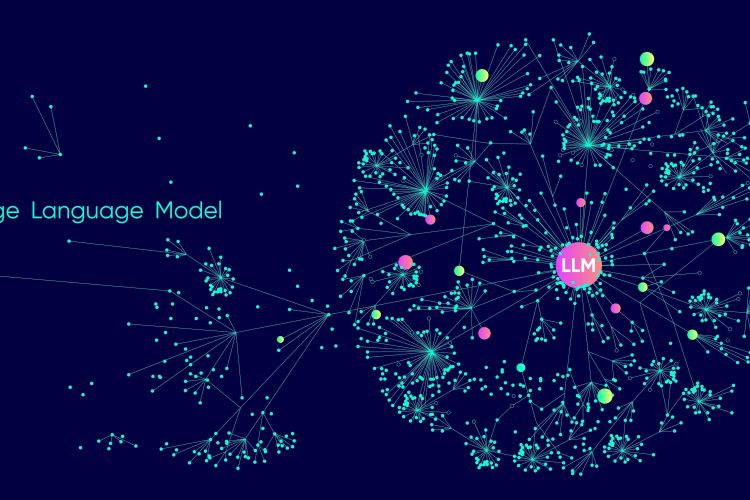
The integration of artificial intelligence (AI) into scientific workflows represents both tremendous opportunity and significant challenges. I recently discussed the subject with two founders at the cutting edge of this transformation: André França of Ergodic and Eli Pollock of Ontologic. We considered the barriers scientists face when adopting AI tools, contrasting approaches to building AI systems for specialised domains, and their visions for how these technologies will reshape scientific work. In this second article of the two-part series, we explore contrasting approaches and look ahead to the future.
Contrasting approaches to AI systems
Singh: You represent two distinct approaches: André advocates for configurable systems where expertise can be integrated to create pseudo-expert systems, while Eli proposes embedding experts directly within the development team. These approaches aren’t mutually exclusive but offer different approaches.
França: There is indeed a spectrum. At one end, you have general tasks like email correction – generic, configurable, low-stakes, but still improvable with more structure. At the opposite end, protein structure prediction requires an expert system built specifically for that purpose with high-quality, problem-specific data.
Reduce preclinical failures with smarter off-target profiling
24 September 2025 | 15:00PM BST | FREE Webinar
Join this webinar to hear from Dr Emilie Desfosses as she shares insights into how in vitro and in silico methods can support more informed, human-relevant safety decisions -especially as ethical and regulatory changes continue to reshape preclinical research.
What you’ll learn:
- Approaches for prioritizing follow-up studies and refining risk mitigation strategies
- How to interpret hit profiles from binding and functional assays
- Strategies for identifying organ systems at risk based on target activity modulation
- How to use visualization tools to assess safety margins and compare compound profiles
Register Now – It’s Free!
Language models can’t inherently make sense of protein data, but they can generate queries, write code to extract useful information, or build dashboards to visualise structures.
The boundary we’re establishing is this: language models can’t inherently make sense of protein data, but they can generate queries, write code to extract useful information, or build dashboards to visualise structures. However, for the next step, you need specialised algorithms. These could be trained with help from configurable systems that manage data and setup, but when dealing with specialised structured data, you need to transition from ‘language model plus extras’ to truly specialised models.
Pollock: The middle case is the billion-dollar question – not just determining protein structure but understanding what it means for a therapeutics business. This is where the greatest demand exists, but it’s also the most challenging problem because it requires both reasoning capabilities and domain-specific scientific knowledge. Whoever cracks this code will define the field’s future.
Singh: It’s like the semiconductor industry’s battle between cheaper MOSFET transistors and expensive high-speed bipolar transistors. Companies invested heavily to make cheaper technologies work at higher frequencies, succeeding until requirements became more complex, bringing specialised hardware back into play.
There’s a continuous trade-off: Do we use generalised systems that keep improving, or specialised models that maintain the leading edge while that edge keeps advancing? As science progresses, specialised tools remain necessary, but general software handles more and more. The 80/20 rule applies, but the pie keeps growing.
Agents: the next frontier
Singh: Let’s talk about this other trajectory that agents unlock.
França: Language models have limitations – they don’t handle mathematics well. Instead of teaching them maths, we let them call on calculators. This is the promise of agents: if you have specialised models for tasks like protein structure prediction, you can teach language models to delegate to these specialised tools.
The result is a generalist system functioning as an architect that understands requirements, maps them to plans, and executes by delegating specialised tasks to purpose-built tools. You get the best of both worlds.
Some companies are raising funds to build fully automated, agent-powered drug discovery labs, which will eventually materialise for certain applications.
Pollock: That’s an elegant description of agents. It’s exciting – if one system can’t do everything, use multiple systems with an orchestrator. However, trust remains an issue. As responsibilities shift to agents, people need confidence in their decision making. We’ll likely see agentic workflows with humans in the loop verifying results.
Some companies are raising funds to build fully automated, agent-powered drug discovery labs, which will eventually materialise for certain applications.
Singh: Business decisions still require business leaders – data-driven science is one thing, but strategic business decisions are another matter entirely.
Visions for the future
Singh: Looking five years ahead, what is your ideal scenario for how scientists will use AI?
França: My vision is for scientists to focus more on science and less on everything else. In the 19th century, entire rooms of people calculated mathematical constants by hand. By the time I was doing my PhD, we had Mathematica to solve differential equations at the click of a button, freeing us to think about science.
AI pushes this concept further – what if scientists could focus entirely on hypothesis generation?
AI pushes this concept further – what if scientists could focus entirely on hypothesis generation? Human ingenuity lies in our ability to connect disparate data points, create mental models, form hypotheses and deduce their consequences. My nirvana is a massive hypothesis-generating machine that exponentially advances science.
Pollock: We’ve used the slogan “focus on the science, not the setup.” It’s exciting to envision scientists simply stating hypotheses and easily understanding their data, without friction at every step – ie, setting up labs, ensuring functionality, cleaning messy data, implementing cutting-edge analysis models.
I’m excited about making tools more accessible and intuitive for scientists. Less about automating critical decisions, more about keeping scientists in the loop with better tools to test hypotheses and progress their work.
The future of human-computer interaction
Singh: Do you believe in seamless voice interfaces replacing keyboards for scientific work?
Pollock: That’s a user interface/user experience question. OpenAI succeeded by creating a simple text-box interface rather than requiring complex setup. The best interface depends on information transfer quality. In science’s precise, concise world, typing allows specificity that conversational interfaces might lose.
Multimodal AI, allowing document and image uploads, offers advantages beyond voice. The optimal interaction method depends on the task.
França: AI interfaces will likely become more amorphous. Different scientific disciplines already have different communication interfaces. Physics happens at blackboards – ideally, my AI system would be there too. I draw a Feynman diagram and it produces results without requiring explicit explanation. The interfaces will vary by field and purpose.
The future of AI in drug discovery is full of promise, with AI and LLMs offering transformative potential, but also creating significant challenges.
The future of AI in drug discovery is full of promise, with AI and LLMs offering transformative potential, but also creating significant challenges. As André and Eli discussed, combining generalist systems with specialised tools could empower scientists to focus more on hypothesis generation and decision making, while delegating data-heavy tasks to AI.
In the next five years, the goal is for scientists to seamlessly interact with AI, allowing them to focus purely on science, not on setup or data management. With the right tools and interfaces, AI could revolutionise how drug discovery is done, making it faster, more accurate and more accessible.
About the authors
Dr Raminderpal Singh is a recognised visionary in the implementation of AI across technology and science-focused industries. He has over 30 years of global experience leading and advising teams, helping early to mid-stage companies achieve breakthroughs through the effective use of computational modelling.
Raminderpal is currently Global Head of AI and GenAI Practice at 20/15 Visioneers and leads the HitchhikersAI.org open-source community. He is also a co-founder of Incubate Bio – a techbio providing a service to life sciences companies who are looking to accelerate their research and lower their wet lab costs through in silico modelling.
Raminderpal has extensive experience building businesses in both Europe and the US. As a business executive at IBM Research in New York, Dr Singh led the go-to-market for IBM Watson Genomics Analytics. He was also Vice President and Head of the Microbiome Division at Eagle Genomics Ltd, in Cambridge. Raminderpal earned his PhD in semiconductor modelling in 1997. He has published several papers and two books and has twelve issued patents. In 2003, he was selected by EE Times as one of the top 13 most influential people in the semiconductor industry.
For more: http://raminderpalsingh.com; http://20visioneers15.com; http://hitchhikersAI.org