Using knowledge graphs in drug discovery (Part 2): how they’re shaping scientific progress
Posted: 30 April 2025 | Andreas Kollegger (Head of GenAI innovation at Neo4), Dr Raminderpal Singh (Hitchhikers AI and 20/15 Visioneers) | No comments yet
In this second interview of the series, Andreas Kolleger, Head of GenAI Innovation at Neo4j, discusses how knowledge graphs and AI are transforming scientific discovery and improving life sciences workflows.
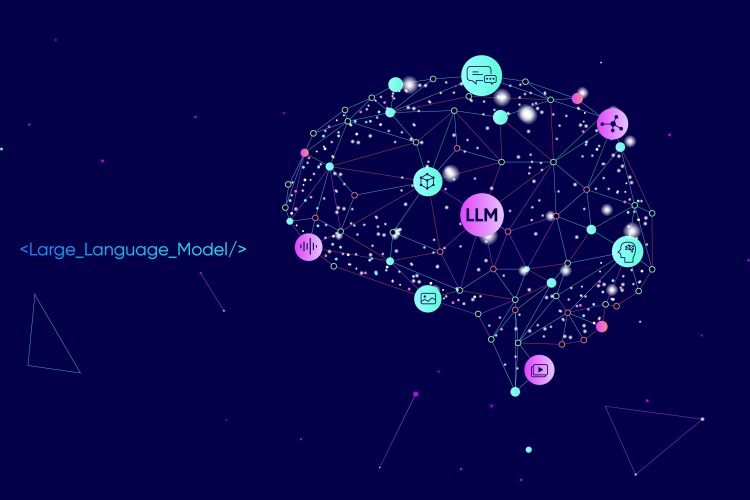
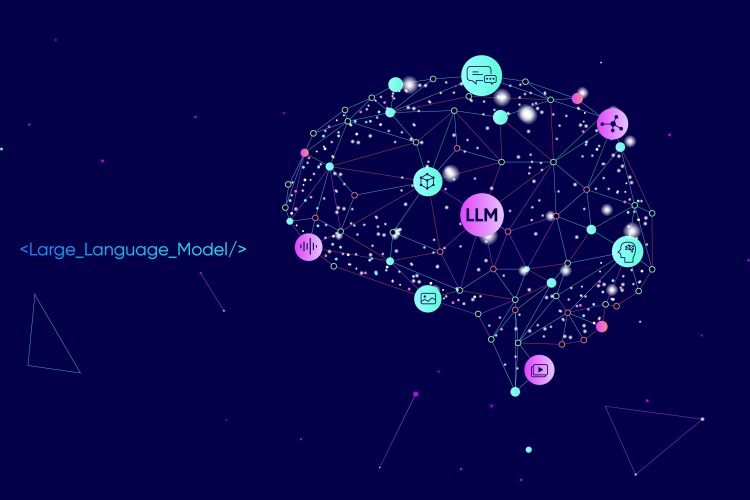
Looking towards the next five years, knowledge graphs are poised to play an increasingly central role in scientific discovery, particularly as reasoning capabilities in AI systems continue to mature. As Kollegger observes, the industry is moving beyond simply making the machinery work to focusing on setting frameworks and motivations for scientific discovery. This evolution promises to accelerate the drug discovery process significantly, though with important caveats about the nature and limitations of AI advancement.
The rise of multi-modal knowledge graphs
A major upcoming development is the shift towards multimodal knowledge graphs. While current knowledge graphs are primarily text-based, future systems will likely incorporate diverse data types – such as molecular structures, laboratory measurements and imaging data. This integration of multiple data modalities will be particularly valuable in drug discovery, where insights often emerge from the combination of computational and experimental data. The challenge lies not just in storing this diverse data, but in creating meaningful relationships between different types of scientific information.
While current knowledge graphs are primarily text-based, future systems will likely incorporate diverse data types – such as molecular structures, laboratory measurements and imaging data.
The future landscape will likely see the emergence of what Kollegger terms a “multi-graph space,” where different types of graphs (geospatial, vector-based, textual) coexist and interact. This architectural approach reflects the reality of how scientific knowledge is organised – different aspects of research require different types of representation. For instance, a drug discovery project might simultaneously need to work with molecular structure graphs, protein interaction networks and clinical trial outcome data. Each of these domains has its own natural graph structure, and the future challenge lies in creating meaningful connections between these different graph spaces.
This multigraph approach will be supported by what Kollegger describes as a “mixture of experts” model in AI systems. Rather than relying on a single generalist AI model, future systems will likely employ specialised models for different types of data and analysis, coordinated by a generalist AI that can integrate insights across domains. This approach mirrors the way human experts collaborate in drug discovery, where specialists in different areas work together to develop new therapeutic approaches.
Knowledge graphs as a trust layer
As AI systems become more sophisticated, the role of knowledge graphs as a ‘trust layer’ between AI and human researchers will become increasingly important. While LLMs can generate insights rapidly, knowledge graphs provide a verifiable foundation of curated relationships that researchers can audit and validate. This aspect will prove particularly crucial as AI systems become more sophisticated in their reasoning capabilities. As Kollegger notes, researchers will want to not only see the conclusions drawn by AI systems but also examine the knowledge graphs that were used to derive those conclusions.
As AI systems become more sophisticated, the role of knowledge graphs as a ‘trust layer’ between AI and human researchers will become increasingly important.
The integration of knowledge graphs with reasoning models represents another frontier of development. Current AI models excel at pattern matching and information retrieval, but the next generation of systems will need to perform more sophisticated reasoning tasks. Knowledge graphs will play a crucial role in this evolution by providing structured, validated information that reasoning models can work with. This combination could enable more complex forms of scientific inference while maintaining traceability and reliability.
Lower barriers to adoption
For organisations looking to adopt knowledge graph technology, the entry point is now more accessible than ever. Tools like Neo4j’s Knowledge Graph Builder and frameworks like LangChain offer user-friendly starting points for building and experimenting with knowledge graphs. The key is to start small, allowing researchers to experience what Kollegger calls the “graph epiphany” – the moment when the value of graph-based representation becomes clear in their specific domain. This approach allows organisations to gradually build expertise while delivering immediate value.
The need for better tools
The tooling landscape for knowledge graphs is also expected to evolve significantly. While current tools excel at creating and managing individual graphs, there is a growing need for better tools to handle multi-graph environments and to support the integration of different data modalities. As Kollegger points out, the industry requires improved means of helping users navigate between different levels of abstraction and different types of graph representations.
The goal is not to replace human expertise but to augment it by providing better tools for managing and reasoning about complex scientific knowledge.
Looking beyond pure technology, the future of knowledge graphs in drug discovery will likely be shaped by their ability to support collaboration between human researchers and AI systems. The goal is not to replace human expertise but to augment it by providing better tools for managing and reasoning about complex scientific knowledge. Knowledge graphs offer a unique advantage here because they represent information in a way that is both machine-actionable and human-interpretable.
As reasoning capabilities in AI systems continue to advance, knowledge graphs are expected to become even more valuable as a structured foundation for scientific discovery. Their ability to represent complex relationships in a way that bridges traditional scientific knowledge and emerging AI capabilities positions them as a crucial technology for the future of drug discovery. However, realising this potential will require continued development of both the underlying technology and the tools that make it accessible to researchers.
To understand the foundations of this topic, see part one of this series.
About the authors
Dr Raminderpal Singh is a recognised visionary in the implementation of AI across technology and science-focused industries. He has over 30 years of global experience leading and advising teams, helping early to mid-stage companies achieve breakthroughs through the effective use of computational modelling.
Raminderpal is currently Global Head of AI and GenAI Practice at 20/15 Visioneers and leads the HitchhikersAI.org open-source community. He is also a co-founder of Incubate Bio – a techbio providing a service to life sciences companies who are looking to accelerate their research and lower their wet lab costs through in silico modelling.
Raminderpal has extensive experience building businesses in both Europe and the US. As a business executive at IBM Research in New York, Dr Singh led the go-to-market for IBM Watson Genomics Analytics. He was also Vice President and Head of the Microbiome Division at Eagle Genomics Ltd, in Cambridge. Raminderpal earned his PhD in semiconductor modelling in 1997. He has published several papers and two books and has twelve issued patents. In 2003, he was selected by EE Times as one of the top 13 most influential people in the semiconductor industry.
For more: http://raminderpalsingh.com; http://20visioneers15.com; http://hitchhikersAI.org
Andreas is a technological humanist. He began at NASA, designing systems from scratch to support scientific missions. In Zambia, he developed medical informatics systems to apply technology for social good. Now at Neo4j, he’s focused on democratising graph databases to validate and extend our understanding of how the world works.
Related topics
Artificial Intelligence, Big Data, Computational techniques, Drug Discovery, Drug Discovery Processes, Informatics
Related organisations
Hitchhikers AI and 20/15 Visioneers, Neo4j