Kickstarting the use of AI for biotechs: part two
Posted: 24 May 2024 | Dr Raminderpal Singh (Hitchhikers AI and 20/15 Visioneers) | No comments yet
Traditional wet lab scientists working on target discovery, drug identification and drug optimisation have an opportunity to catch up with their AI-enabled peers – but why should they, and how? In this article – the second of a three-part series – Dr Raminderpal Singh touches on methods that are being implemented in early drug discovery. They include LLMs, protein modelling, traditional prediction algorithms and data curation.
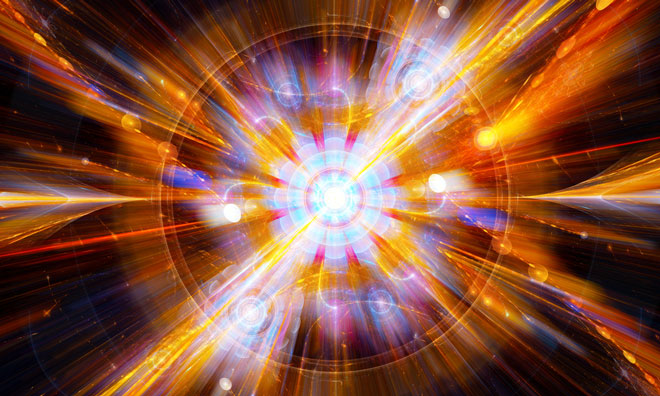
In the last article, I noted select application areas where artificial intelligence (AI) & data can or are already being used:
- Generating and analysing existing data
- Designing compound structures
- Designing in vitro experiments
- Understanding and modelling biological mechanisms
- Extracting deep insights from across literature and study reports
- Designing proteins.
Below are example technology areas for AI and data that are driving significant advancements in the above application areas. More explanation and practical tips for using these technologies will be explored in future articles.
- The elephant in the room is large language models – commonly called LLMs. This is the ChatGPT-like1 environment that we have become rapidly familiar with over the last couple of years. In principle, LLMs can change the world of drug discovery (as they are often claiming to do), but biology and chemistry are full of nuances and the expectations for how LLMs will actually impact drug discovery need to be kept reasonably low for the moment.
- Another big field of advancement is protein modelling and simulation. There are several technologies and specific tools being rolled out in this area. The latest exciting advancement is AlphaFold 3, which was just released.2 AlphaFold 3 has been built to model DNA, RNA and smaller molecules (ligands).3
- The third area is predictive modelling (or statistical inference). This is a traditional application for AI (including machine learning). These methods are extensions of classical statistical techniques that we learnt at school, such as linear regression (fitting a straight line to data points). The big advancement over the last two to three decades has been the power of the new algorithms, which leverage cheap compute processing and storage (think cloud) and cheap data generation (eg, $100 whole genome sequencing).
- AI technologies cannot work their magic without sufficient quality curated data, and generating such data is challenging – especially with in vitro lab tests. According to a prominent article ten years back,4 data scientists spend up to 80 percent of their time mired in the mundane labour of collecting and preparing unruly digital data, before it can be explored for useful nuggets. There are academics and companies actively working on solving this challenge, but biology and chemistry is hard and lab data is often messy and irreproducible. For those looking to learn the basics of data quality, a good start is the 7 C’s framework.5
Several major technology areas are not included above, such as cheminformatics and bioinformatics. These are foundational to drug discovery and will be discussed in future articles.
In the next article, going live on Thursday 20 June, I will discuss key decisions that biotech CEOs and CSOs need to make and their associated risks (including costs) in adopting AI and data technologies.
References
1 Wikipedia. ChatGPT [Internet] 2024 [updated 2024 May 12; cited 2024 May] Available from: https://en.wikipedia.org/wiki/ChatGPT
2 Howe NP, Thompson B. Alphafold 3.0: the AI protein predictor gets an upgrade. Nature [Internet] 2024 [updated 2024 May 8; cited 2024 May]. Available from: https://www.nature.com/articles/d41586-024-01385-x
3 Emilia David. Google DeepMind’s new AI can model DNA, RNA, and ‘all life’s molecules’. The Verge [Internet] 2024 [updated 2024 May 8; cited 2024 May] Available from: https://www.theverge.com/2024/5/8/24152088/google-deepmind-ai-model-predict-molecular-structure-alphafold
4 Lohr S. For Big-Data Scientists, ‘Janitor Work’ Is Key Hurdle to Insights. The New York Times [Internet] 2014 [updated 2024 ; cited 2024 May] Available from: https://www.nytimes.com/2014/08/18/technology/for-big-data-scientists-hurdle-to-insights-is-janitor-work.html
5 Agre JR, Gordon KD, Vassiliou MS. The Seven C’s of Data Curation for the Two C’s – Command and Control. Institute for Defense Analyses [Internet] 2015 [updated 2015 February; cited 2024 May] Available from: https://www.ida.org/research-and-publications/publications/all/t/th/the-seven-cs-of-data-curation-for-the-two-cscommand-and-control
About the author
Dr Raminderpal Singh
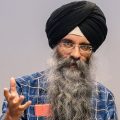
Dr Raminderpal Singh is a recognised key opinion leader in the techbio industry. He has over 30 years of global experience leading and advising teams on building computational modelling systems that are both cost-efficient and have significant IP value. His passion is to help early to mid-stage life sciences companies achieve novel biological breakthroughs through the effective use of computational modelling.
Raminderpal is currently leading the HitchhikersAI.org open-source community, accelerating the adoption of AI technologies in early drug discovery. He is also CEO and co-Founder of Incubate Bio – a techbio providing a service to life sciences companies who are looking to accelerate their research and lower their wet lab costs through in silico modelling.
Raminderpal has extensive experience building businesses in both Europe and the US. As a business executive at IBM Research in New York, Dr Singh led the go-to-market for IBM Watson Genomics Analytics. He was also Vice President and Head of the Microbiome Division at Eagle Genomics Ltd, in Cambridge. Raminderpal earned his PhD in semiconductor modelling in 1997. He has published several papers and two books and has twelve issued patents. In 2003, he was selected by EE Times as one of the top 13 most influential people in the semiconductor industry.
For more: http://raminderpalsingh.com ; http://hitchhikersAI.org ; http://incubate.bio
Related topics
Artificial Intelligence, Informatics
Related organisations
HitchhikersAI