New algorithm forms atlas of histomorphological phenotypes
Posted: 13 June 2024 | Ellen Capon (Drug Target Review) | No comments yet
The algorithm can accurately diagnose cases of lung adenocarcinoma, determining structural features that are statistically most significant for assessing disease severity and likelihood of tumour recurrence.
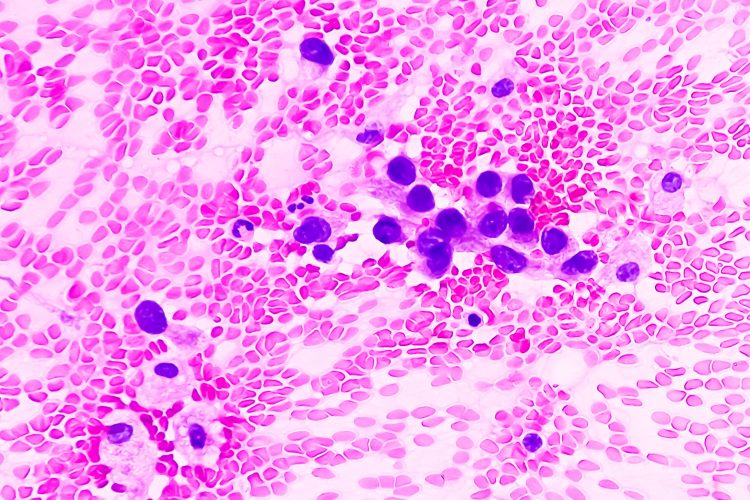
Researchers at NYU Langone Health’s Perlmutter Cancer Center and the University of Glasgow have developed an algorithm based on data from almost 500,000 tissue images. Driven by artificial intelligence (AI), this programme can accurately diagnose cases of adenocarcinoma, the most common form of lung cancer.
The algorithm provides an unbiased, detailed and dependable second opinion for patients and oncologists about the presence of adenocarcinoma, and the likelihood and timing of its return, by incorporating the structural features of tumours from 452 adenocarcinoma patients. These patients are part of the 11,000 patients in the United States National Cancer Institute’s Cancer Genome Atlas. As well as this, the team highlight that the algorithm is independent and self-taught, determining which structural features are statistically most significant for assessing the severity of disease and had the largest impact on tumour recurrence.
The algorithm, specifically named histomorphological phenotype learning (HPL), accurately distinguished between two similar lung cancers, adenocarcinoma and squamous cell cancers, 99 percent of the time. Also, the HPL programme was 72 percent accurate at predicting the likelihood and timing of cancer’s return after therapy, compared to the 64 percent accuracy in the predictions made by pathologists.
Dr Nicolas Coudray, study lead investigator and bioinformatics programmer at NYU Grossman School of Medicine and Perlmutter Cancer Center, explained: “Our new histomorphological phenotype learning programme has the potential to offer cancer specialists and their patients a quick and unbiased diagnostic tool for lung adenocarcinoma that, once further testing is complete, can also be used to help validate and even guide their treatment decisions.”
He added: “Patients, physicians, and researchers know they can rely on this predictive modelling because it is self-taught, provides explainable decisions, and is based only on the knowledge drawn specifically from each patient’s tissue, including such features as its proportion of dying cells, tumour-fighting immune cells, and how densely packed the tumour cells are, among other features.”
Study co-senior investigator Dr Aristotelis Tsirigos is a professor in the Departments of Pathology and Medicine at NYU Grossman School of Medicine and Perlmutter Cancer Center, where he also is co-director of precision medicine and director of its Applied Bioinformatics Laboratories. He stated that, owing to such tools, pathologists will be studying tissue scans on their computer screens, and less on microscopes, and then using AI programmes to analyse the image and produce its own image of the scan. The new image will provide a detailed breakdown of the tissue’s content. Potentially, it may note the percentage of necrosis and tumour infiltration, and what this means for survival.
Developing the algorithm
The researchers first analysed lung adenocarcinoma tissue slides from the Cancer Genome Atlas to develop the HPL algorithm. Lung adenocarcinoma is histologically heterogeneous, with five distinct histologic growth patterns: bronchioloalveolar (lepidic), acinar, papillary, micropapillary, and solid.1 Having these characteristic features was the reason why this disease was chosen to develop the algorithm.
There were 46 key histomorphological phenotype clusters in both normal and diseased tissue, found from the images from the slides that had been digitally scanned and broken into 432,231 small quadrants. Their results were confirmed by further and separate testing on tissue images from 276 men and women treated for adenocarcinoma at NYU Langone from 2006 to 2021.
The team aim to use the HPL algorithm to assign to each patient a score between zero and one that indicates their statistical chance of survival and tumour recurrence for up to five years. HPL will become increasingly more accurate as more data is added over time, because the algorithm is self-learning. The team have put their programming code online and have aim to make the novel HPL tool freely available upon completion of further testing.
Tumour recurrence was associated with characteristics including high tile percentages of dead cancer cells and lymphocytes, as well as tumour cells in the lungs’ outer linings. An increased likelihood of survival was associated with high percentages of unchanged or preserved lung sac tissue, and lack of or small presence of inflammatory cells.
Dr Tsirigos concluded that they are planning to develop HPL-like programmes for other prevalent cancers, like breast and ovarian cancers which are also based on distinctive morphological features. Epithelial ovarian cancer has five clinically and genetically distinct histotypes, which have several reproductive and hormonal risk factors, although differences also exist.2
Furthermore, to improve the accuracy of the current adenocarcinoma HPL programme, the team will include other data from hospital electronic health records about other illnesses and diseases, or even income and home ZIP codes.
This study was published Nature Communications.
References
1 Behrens C, Galindo H, Kadara H, et al. Histologic patterns and molecular characteristics of lung adenocarcinoma associated with clinical outcome. Cancer [Internet]. 2011 October 21 [cited 2024 June 13];118(11):2889-2899. Available from: https://doi.org/10.1002/cncr.26584
2 Jordan S and Webb P. Global epidemiology of epithelial ovarian cancer. Nature Reviews Clinical Oncology [Internet]. 2024 March 24 [cited 2024 June 13];21:389-400. Available from: https://doi.org/10.1038/s41571-024-00881-3
Related topics
Analysis, Artificial Intelligence, Oncology
Related conditions
Cancer Research, Lung adenocarcinoma
Related organisations
NYU Langone's Perlmutter Cancer Center, University of Glasgow