Turning rare diseases into efficient targets with AI
Posted: 14 March 2017 | Dr Patrick Keohane (Chief Medical Officer: BenevolentBio) | No comments yet
BenevolentBio’s CMO, Dr Patrick Keohane, says the industry is on the cusp of an artificial intelligence (AI) revolution…
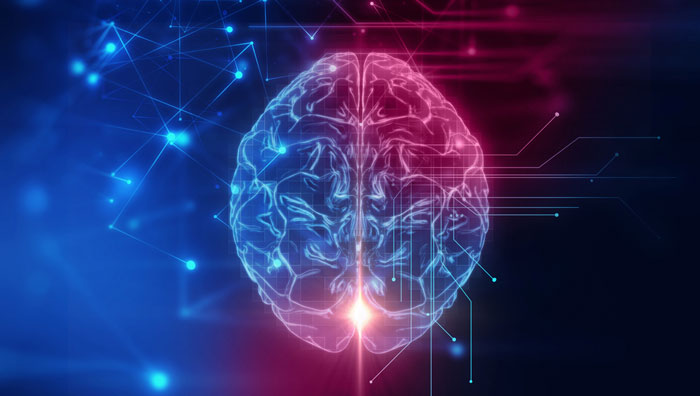
Medical research is entering a period of rapid transformation, driven by the explosion of scientific data, rapid advances in artificial intelligence (AI), and the development of extremely powerful computers.
This convergence enables researchers to access enormous and diverse datasets to rapidly form and test scientific hypotheses. It is disrupting the way we identify, validate, and transform scientific concepts into potential Healthcare solutions – ultimately revolutionising the scientific process.
Better medicines at a reasonable cost
AI enables access and the more effective use of existing information for the discovery and development of better drugs at a speed previously unimagined.
This is helping to meet the high demand from society to provide better medicines at a reasonable cost, but also influencing how we are looking to treat rarer forms of disease. Because the legacy drug discovery processes are data and time intensive, with high attrition and cost, traditional pharma companies are unable to identify all the opportunities in their research and development (R+D) portfolios or pursue every drug candidate.
AI provides a uniquely 21st Century opportunity to change this.
Where is the industry failing rarer diseases and where can AI help?
Despite the deployment of many advanced technologies such as high-through screening, and enabling biomarkers in the last two decades, the overall success rate to develop a new medicine remains in the 5% range with costs spiraling to around $3 billion per product launched.
Only one in ten drugs make it to FDA approval stage. Rarer disease drug discovery can suffer most, as every development needs to cover its financial cost and offer stakeholders some return.
Only one in ten drugs make it to FDA approval stage
It is estimated that the average academic researcher reads 250-270 articles per year, but, there are now over 50 million scientific articles worldwide. This means that it is becoming harder and harder to access all of these insights to confirm or disprove a given hypothesis, to act quickly with confidence, or recognise a novel and unsuspected potential medical use of a drug candidate.
Combined data sources
Using greater access and evaluation of data to reduce early attrition is attractive to make candidates put forward for clinical trials more successful. Use of all data sources available combined with rapid powerful analysis is key. But the use of AI to collapse the time required to sort and summarise key associations has the potential to transform attrition as well as identification of previously unknown potential uses for a drug candidate.
AI is proving a valuable tool to solve this problem. The technology can access all of the data, with the support of supercomputers, analyse and interrogate it, and make the connections where researchers simple don’t have the capacity to do so.
It can help to unearth information and join the dots where we hadn’t thought to before. This is starting to speed up drug discovery, has enabled us to form novel insights more effectively from existing data, and is showing the potential to dramatically accelerate R+D pipeline decisions and delivery.
One example of this is BenevolentAI’s use of our own proprietary AI to research suitable drug compounds for a form of Motor Neurone Disease, Amyotrophic Lateral Sclerosis (ALS). Our technology was able to review billions of sentences and paragraphs from millions of scientific research papers and abstracts. The AI platform we’ve developed then interrogated this information to form direct relationships between the data and organised it into ‘known facts’. These ‘known facts’ then helped our team of scientists to establish hypotheses to explore. The AI augments the researcher’s existing expertise to form a prioritised list of hypotheses to explore, which, with further interrogation, have now been whittled down to just five. These are now all undergoing testing in the lab.
New mechanisms for disease modification
This process has allowed us to explore the potential for new mechanisms for disease modification in a timescale pharma companies previously could only dream of. It’s allowing us to also put forward highly informed hypotheses.
This has made the method of making the drug discovery process quicker and more effective, enabling us as a company to explore rarer forms of disease.
When can we be looking to see change?
The true benefits of AI may not be realised and fully applied by the life science and Pharma industry for another three to five years. In addition to public scientific data sources, there is an enormous amount of untapped pre-clinical and clinical data located in Pharma research and development organisations. There are also other assets parked within Commercial R+D organisations without any plans to develop or monetise them. These are just waiting to be transformed by AI.
Change also depends on the speed of registry and large database controllers, and regulators (like the Medical and Healthcare products Regulatory Agency (MHRA) here in the UK and the Food and Drug Agency (FDA) in the US) to gain interest in, support and enable the application of these new technologies and techniques.
Only then can AI fully leverage interrogation of the diverse datasets of general and disease specific populations, creating new opportunities and accelerating all the stages of new medicines development from drug discovery all the way to point of care.
AI tools and techniques
We’ve already seen interest in these tools and techniques accelerate over the last couple of years. However, both ends of the drug life span are starting to build momentum in terms of emerging capabilities with AI technology. The development we’re focusing on is scientific, very much focused on drug discovery and development, and the beginning of the chain of new and better medicines development.
Other companies like IBM Watson and Google DeepMind have been identifying point of care uses. They are focused on using AI to improve how healthcare systems can identify opportunities to manage patient needs better, while BenevolentAI is focused on accelerating identification and development of better medicines for patients.
Benefit to clinical pipelines
AI has the potential to also benefit the pipeline at the clinical trial stage, much of what my work focuses on. AI can augment a researcher’s understanding of the data points involved. It provides greater depth in terms of how certain patients will respond to treatments, helping to better recruit patients for trials. This can also improve safety, more readily identifying the potential side effects and how certain drugs might affect people with other conditions too.
It can also help clinicians to identify patterns in any placebos used, more effectively. Unlocking this data can take the insight for these researchers to a new level.
It is hard to pin point an exact time as to when the AI revolution will be fully felt across the whole drug discovery and development pipeline, but the revolution has unquestionably started. The fundamental tipping point will come when we link all of this currently untapped information together.
Related topics
Artificial Intelligence, Biomarkers, Research & Development
Related conditions
Amyotrophic Lateral Sclerosis (ALS), Rare diseases
Related organisations
BenevolentAI, BenevolentBio, IBM Watson, Medicines and Healthcare Products Regulatory Agency (MHRA), U.S. Food and Drug Administration (FDA)
Related people
Dr Patrick Keohane