Clinically-oriented phenotypic screening: understanding and exploiting the dynamic nature of the cancer cell phenotype
Posted: 6 December 2017 | David Bailey, Fredrik Svensson | No comments yet
Understanding the cancer cell phenotype is central to both cancer diagnosis and treatment. Discrete molecular signatures among cancer phenotypes underpin patient stratification, while rapid methods for detailed phenotyping in the clinic (via liquid biopsies and tumour imaging) enable the delivery of personalised, effective precision medicines to the cancer patient…
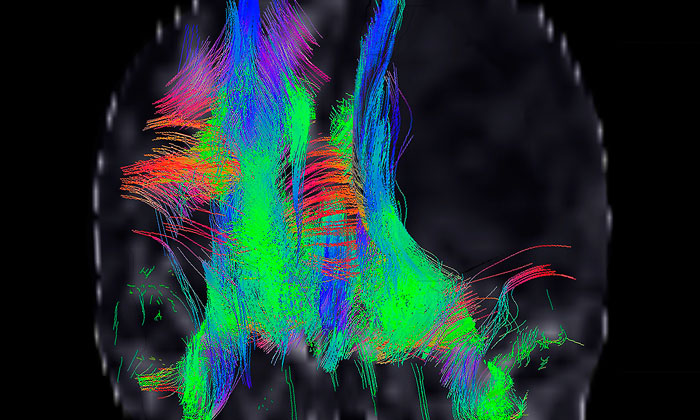
Here, briefly, we describe how emerging data are defining molecular phenotypes among specific cancer cell lineages, and how these can be used in phenotypic screening to discover and profile drugs which modulate them. We focus on recent advances in molecular phenotyping for human glioma and its high-grade counterpart, glioblastoma multiforme (GBM), and review how laboratory advances for glioma are translating to the clinic.
Phenotypic screening systems underpin much of cancer drug discovery, with assays measuring proliferation, differentiation and other phenotypes forming a basis for reliably delivering new first-in-class drugs to the patient.1 In the literature, discussions have focused on the relative success of phenotypic drug discovery (PDD) compared to target-based drug discovery (TDD).2,3 However, the debate is now shifting to translating PDD screens from the lab to the clinic.4,5 In the lab, discussion centres on the authenticity of the cell and tissue systems being used for drug screening, but in the clinic, the key issues are the definition, validation and therapeutic utility of the phenotypic endpoints themselves.
Cancer-derived cell and animal models, and the human cancer phenotypes they recapitulate have long been the workhorse for studies of cancer drugs’ mode of action, efficacy and selectivity. Established cell lines provide robust and readily accessible cellular reagents for technology assessment, tool development and pathway analysis, and they continue to be used as the first port of call for initial translation of TDD ‘lead’ compounds into well-characterised cellular contexts.
Where established cancer cell lines can fail, however, is in clinically-relevant, disease-related hypothesis testing. The use of established cancer cell lines in this area, especially those that have been cultured for decades in artificial media containing high levels of growth factors and other serum components, comes with many caveats.6 Many established cell lines in routine use as cancer models have become ‘addicted’ to their ex vivo growth conditions, having acquired mutations that favour their growth and propagation under these unnatural laboratory conditions, making them in practice a poor surrogate for disease in situ.7
To mitigate these concerns, several new cell-based platforms have been established for use in phenotypic screening campaigns for glioma.8 Some of these, such as the Human Glioblastoma Cell Culture (HGCC) resource, comprise recently derived, well-characterised and clinically-annotated glioma cell lines representing all the tumour cell lineages present in glioma and glioblastoma patients, and are publicly available.9 Crucially, the basic process for producing authentic patient-derived cell systems for phenotypic screening at point-of-care is now well established.
Much of the current interest in PDD as a drug discovery approach can be attributed to innovations in imaging and cell separation technology, especially single cell separation and analysis by fluorescence-activated cell sorting (FACS), facilitated by high-content cell imaging and immunophenotyping.10 Hand-in-hand with exciting new functional genomics technologies such as CRISPR-Cas 9 gene editing, these approaches provide powerful, high-throughput data-gathering platforms for phenotypic screening.11
Heterogeneity and plasticity of glioma cell lineages
Glioblastoma cell populations display a high degree of phenotypic and genetic heterogeneity, as do many other cancers. Nevertheless, within this heterogeneity histopathological markers characteristic of glioma progression can be identified.12 A clinical analysis network for glioma staging, known as GLASS (the Glioma Longitudinal Analysis Consortium), has recently been built around the need to integrate histopathological and molecular data across glioma, to support diagnosis and prognostic grading in the clinic.13,14
Many of the observed molecular changes characteristic of gliomagenesis recapitulate normal neurogenesis, with processes such as the reversible phenotypic change ‘epithelial-mesenchymal transition’ (EMT), mirroring facets of early embryonic development.15 Cancer cells also retain many elements of their normal adult phenotypic programmes, although they actually originate from discrete cancer-initiating stem cell populations. Studies of glioma stem cell lineages show characteristic patterns of quiescence, proliferation and differentiation, driven by particular transcription factors.11
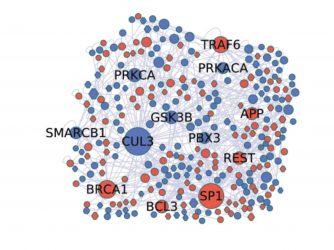
Figure 1: Molecular phenotypes in the laboratory: proteomic reconstruction of a GBM stem cell.17
Recent results using human induced pluripotent stem cells (iPSCs) as a model system for gliomagenesis show that dysregulation of receptor tyrosine kinase and p53 signalling in vitro recapitulates that seen in glioma tumour initiating cells in vivo. Transplantation of engineered iPSC-derived neural progenitor cells leads to highly aggressive tumours containing undifferentiated stem cells and their differentiated derivatives, capturing many elements of the cellular heterogeneity seen in glioma and glioblastoma.16 A detailed understanding of the molecular components of these stages of glioma evolution, and how they are related to developmental plasticity, is vitally important in the search for new GBM biomarkers and therapeutic targets (Figure 1).17
Despite prodigious preclinical efforts in the laboratory, the first generation of TDD-led approaches to glioma have not translated to efficacy in the clinic. When glioma was found to express high levels of the mutant epidermal growth factor receptor EGFRvIII, it was hoped that drugs specifically targeting this mutant EGFR might halt, or at least slow, glioma progression. This has turned out not to be the case.18 Equally, mono-drug therapies targeting other kinase activities – for example, the multi-kinase inhibitor sorafenib – although showing efficacy in xenograft models, have failed in the clinic.19
Combination therapies: from GLASS to WINDOW
Acknowledging the pronounced functional heterogeneity seen in the glioma phenotype, research has swung towards combination therapy, in which molecularly targeted therapies are aggregated. In other diseases such as AIDS, combination therapy, although not without issues, has shown impressive results.20 In glioma, combinations of up to six drugs with proven efficacy in animal models,21 including combinations of drugs with different modalities,22 are now being tested in the clinic. We will soon know whether any of these regimens are effective in patients.
To expedite combination testing, The Brain Tumour Charity (UK) has recently funded a new combination therapy platform, WINDOW, to provide drug prototypes and further resources for systematically evaluating combination therapies in glioblastoma drug discovery, enhanced by CRISPR-enabled genome editing approaches to study any associated drug-resistance mechanisms.23 In our view, targeting glioma in phenotypic drug screening campaigns by combining panels of drugs with defined molecular mechanisms, together with measurement of differentially expressed phenotypic markers, holds considerable promise as a drug discovery approach.
Bringing phenotypic imaging into the clinic
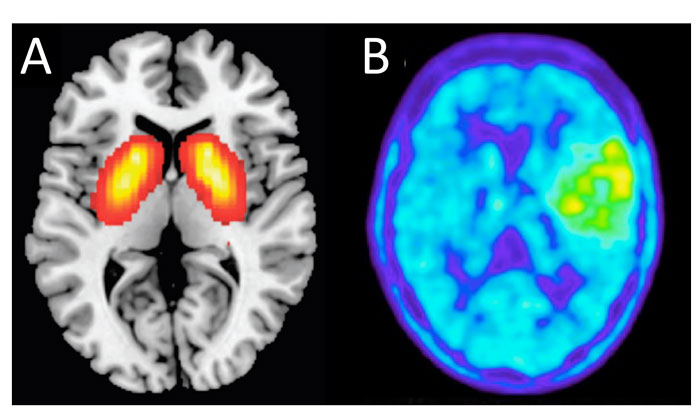
Figure 2: Phenotypic screening in the clinic. PANEL A: noninvasive PET imaging of a schizophrenia drug target. PANEL B: FET PET imaging of a metabolically active low-grade oligoastrocytoma brain tumour.
Magnetic resonance imaging (MRI) in conjunction with histopathology at first surgery is the current benchmark for brain tumour prognosis.24 Imaging science for the brain and its neoplasms is advancing rapidly. In particular, information from drug-target-directed positron emission tomography (PET) scanning is building an increasing understanding of differential drug target expression in the brain, opening completely new avenues for targeted imaging (Figure 2A).25,26
In parallel, generic metabolic imaging, in which the metabolic status of the patient’s tumour is measured as a surrogate marker of treatment response,27 offers possibilities for monitoring drug efficacy directly in the patient (Figure 2B). Used in combination, these techniques should provide prognostic insights into the functional phenotypes of glioma and glioblastoma in situ.
Furthermore, the use of imaging in combination with liquid biopsies to confirm the mutational status of individual tumours,28 promises to rapidly and non-invasively guide therapy in support of precision medicine, on a patient-by-patient basis.
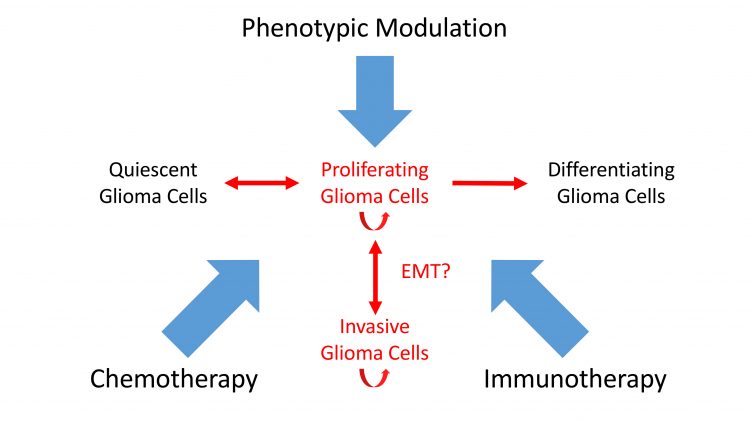
Figure 3: A new view of combination therapy. Immunotherapy, phenotypic modulation and chemotherapy combine to target intransigent cancers such as GBM.
Glioblastoma – the endgame
A detailed understanding of the molecular basis of the glioma cell phenotype is emerging as a central requisite for the design and application of new glioma treatments. Defining the patient’s tumour cell biology and personal tumour phenotypic response will underpin radically new approaches to glioblastoma, including dendritic cell-based vaccination,29 CAR T-cell immunotherapy,30 and drug-based phenotypic modulation2, all of which will have a role to play in eradicating this devastating disease.
Encouragingly, the pace of phenotype discovery in difficult-to-treat cancers such as glioblastoma is markedly quickening. A new echelon of integrated, phenotypically-informed cancer drug treatments are poised to enter the clinic and deliver personalised precision medicines for glioma patients.
Acknowledgements
The authors thank the Brain Tumour Charity (UK) for funding their work as part of the WINDOW consortium, and thank other members of the WINDOW consortium, especially Mr Colin Watts, Dr Bart Westerman, Dr Emmanouil Metzakopian and Dr Tom Wurdinger, together with Professor Steven Pollard (CRUK, Edinburgh) and Dr Ed Zanders (PharmaGuide Ltd, Cambridge) for inspiring scientific discussions. The illustrations in Figures 1 & 2 are provided courtesy of Prof Steven Pollard and Jeroen Krijsveld (Edinburgh Cancer Research UK Centre and MRC Centre for Regenerative Medicine, University of Edinburgh), Prof Gunnar Antoni (Uppsala University Hospital, Uppsala, Sweden) and Prof Karl-Josef Langen (Forschungszentrum Julich GmbH, Julich, Germany).
Biographies
FREDRIK SVENSSON leads IOTA’s computational chemistry and informatics work. He was awarded his PhD in 2015 from Uppsala University, Sweden, followed by postdoctoral work at the University of Cambridge. Fredrik’s current research focus is on the role of FBDD and lead optimisation in drug discovery and development.
DAVID BAILEY is a founding director of the drug discovery company IOTA Pharmaceuticals, which is based in Cambridge, UK. Previously, he worked in the Department of Tumor and Cell Biology at SmithKline & French in Philadelphia and was head of the Molecular Sciences Department at Pfizer Central Research in Sandwich, UK. During his work at Pfizer he was involved in the discovery and development of the PDE inhibitor sildenafil (Viagra) and the antifungal agent fluconazole (Diflucan).
References
- Swinney & Anthony. How were new medicines discovered? Nat Rev Drug Discov. 2011;10:507-19.
- Moffat et al. Phenotypic screening in cancer drug discovery – past, present and future. Nat Rev Drug Discov. 2014;13:588–602.
- Eder et al. The discovery of first-in-class drugs: origins and evolution. Nat Rev Drug Discov. 2014;13:577-87.
- Horvath et al. Screening out irrelevant cell-based models of disease. Nat Rev Drug Discov. 2016;15:751-769.
- Moffat et al. Opportunities and challenges in phenotypic drug discovery: an industry perspective. Nat Rev Drug Discov. 2017;16:531–543.
- Villa & Mischel. Shared intelligence: a patient-derived deeply characterized glioblastoma cell line resource. EBioMedicine 2015;2:1274-5.
- Miller et al. Transcription elongation factors represent in vivo cancer dependencies in glioblastoma. Nature 2017;547:355-359.
- Pollard et al. Glioma stem cell lines expanded in adherent culture have tumor-specific phenotypes and are suitable for chemical and genetic screens. Cell Stem Cell 2009;4:568-80.
- Yuan et al. The human glioblastoma cell culture resource: validated cell models representing all molecular subtypes. Ebiomedicine 2015;2:1351–1363.
- Meyer et al. Single cell-derived clonal analysis of human glioblastoma links functional and genomic heterogeneity. Proc Natl Acad Sci USA. 2015;112:851-6.
- O’Duibhir et al. Accelerating glioblastoma drug discovery: convergence of patient-derived models, genome editing and phenotypic screening. Mol Cell Neurosci. 2017;80:198-207.
- Huse et al. Molecular subclassification of diffuse gliomas: seeing order in the chaos. Glia 2011;59:1190-9.
- Louis et al. The 2016 World Health Organization classification of tumors of the central nervous system: a summary. Acta Neuropathol. 2016;131:803-820.
- The Glioma Longitudinal Analysis Consortium. Glioma through the Looking GLASS: the molecular evolution of diffuse gliomas. 2017 www.biorxiv.org/content/early/2017/10/02/196139
- Ye & Weinberg. Epithelial-Mesenchymal Plasticity: a central regulator of cancer progression. Trends Cell Biol. 2015;25:675-686.
- Sancho-Martinez et al. Establishment of human iPSC-based models for the study and targeting of glioma initiating cells. Nat Commun. 2016;7:10743.
- Okawa et al. Proteome and secretome characterization of glioblastoma-derived neural stem cells. Stem Cells 2017;35:967-980.
- Westphal et al. EGFR as a target for glioblastoma treatment: an unfulfilled promise. CNS Drugs 2017. doi: 10.1007/s40263-017-0456-6.
- Carra et al. Sorafenib selectively depletes human glioblastoma tumor-initiating cells from primary cultures. Cell Cycle 2013;12:491-500.
- Garbelli et al. How to win the HIV-1 drug resistance hurdle race: running faster or jumping higher? Biochem J. 2017;474:1559-1577.
- Kast et al. Blocking epithelial-to-mesenchymal transition in glioblastoma with a sextet of repurposed drugs: the EIS regimen. Oncotarget 2017;8:60727-60749.
- Gravina et al. The brain-penetrating CXCR4 antagonist, PRX177561, increases the antitumor effects of bevacizumab and sunitinib in preclinical models of human glioblastoma. J Hematol Oncol. 2017;10:5.
- WINDOW: www.thebraintumourcharity.org/media-centre/news/latest-news/awarding-window-consortium-15-million-new-research/
- Villanueva-Meyer et al. Current Clinical Brain Tumor Imaging. Neurosurgery 2017;81:397–415.
- Hostetler et al (2016) Preclinical characterization of the Phosphodiesterase 10A PET tracer [(11)C]MK-8193. Mol Imaging Biol. 2017;18:579-87.
- Boden et al. Striatal phosphodiesterase 10A and medial prefrontal cortical thickness in patients with schizophrenia: a PET and MRI study. Transl Psychiatry 2017;7:e1050.
- Langen et al. Imaging of amino acid transport in brain tumours: Positron emission tomography with O-(2-[18F]fluoroethyl)-L-tyrosine (FET). Methods 2017;130:124-134.
- Best et al. Liquid biopsies in patients with diffuse glioma. Acta Neuropathol. 2015;129:849-65.
- Desai et al. Emerging immunotherapies for glioblastoma. Expert Opin Emerg Drugs 2016;21:133-45.
- O’Rourke et al. A single dose of peripherally infused EGFRvIII-directed CAR T cells mediates antigen loss and induces adaptive resistance in patients with recurrent glioblastoma. Sci Transl Med. 2017;9:pii: eaaa0984.
Related topics
Screening