New AI model can evaluate invasive breast cancer outcomes
Posted: 28 November 2023 | Drug Target Review | No comments yet
Combining cancerous and non-cancerous cell patterns, the AI model evaluates breast cancer outcomes better than expert pathologists.
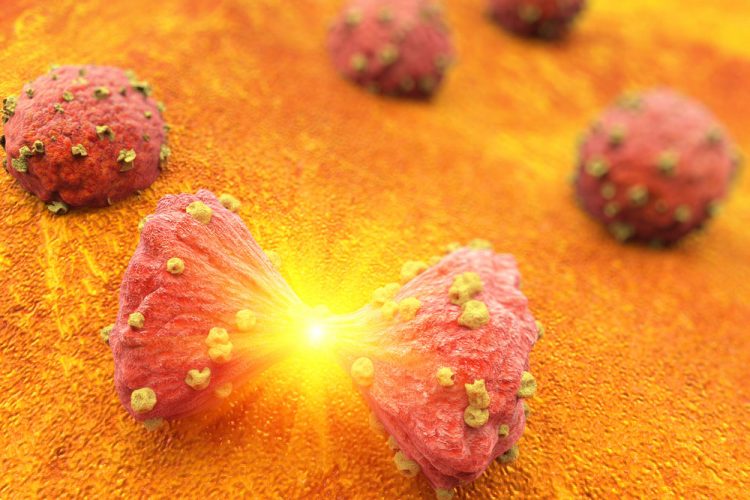
Researchers from Northwestern Medicine have developed a new Artificial intelligence (AI) tool to spare breast cancer patients’ unnecessary chemotherapy treatments by using a more accurate method of predicting their outcomes, which was better than evaluations made by expert pathologists. This is the first study to use AI for comprehensive evaluation of both the cancerous and non-cancerous elements of invasive breast cancer.
The AI tool was able to identify breast cancer patients who are currently classified as high or intermediate risk but who become long-term survivors.
Pathologist diagnosis
About one in eight US women will receive a breast cancer diagnosis in their lifetime. During diagnosis, a pathologist studies the cancerous tissue to establish how abnormal the tissue appears. This process, called grading, concentrates on cancer cells’ appearance, and has stayed mostly unchanged for decades. The grade is used to determine what treatment the patient will receive.
Many studies of breast cancer biology have demonstrated that the non-cancerous cells, including cells from the immune system and cells that provide form and structure for the tissue, are significant for sustaining or inhibiting cancer growth.
“Our study demonstrates the importance of non-cancer components in determining a patient’s outcome”
Corresponding author Dr Lee Cooper, Associate Professor of Pathology at Northwestern University Feinberg School of Medicine, said: “Our study demonstrates the importance of non-cancer components in determining a patient’s outcome… The importance of these elements was known from biological studies, but this knowledge has not been effectively translated to clinical use.”
The AI model evaluates breast cancer tissue from digital images that measures the appearance of cancerous cells, non-cancerous cells, and interactions between them.
“These patterns are challenging for a pathologist to evaluate as they can be difficult for the human eye to categorise reliably,” said Cooper, also a member of the Robert H. Lurie Comprehensive Cancer Center of Northwestern University. “The AI model measures these patterns and presents information to the pathologist in a way that makes the AI decision-making process clear to the pathologist.”
Therapeutic response
The AI model analyses 26 different properties of a patient’s breast tissue to create an overall prognostic score, and generates individual scores for the cancer, immune and stromal cells to explain the overall score to the pathologist. A good prognosis score could be because of properties of the patients’ immune cells, but for others it may be due to properties of their cancer cells. Individualised treatment plans could be formed using this information.
Also, this model could assess therapeutic response, allowing treatment to be escalated or de-escalated depending on how the microscopic appearance of the tissue changes over time. This could be useful to reduce the duration or intensity of chemotherapy, as chemotherapy is linked to unpleasant and harmful side effects like nausea, or more rarely, heart damage.
“We also hope that this model could reduce disparities for patients who are diagnosed in community settings,”
“We also hope that this model could reduce disparities for patients who are diagnosed in community settings,” Cooper stated. “These patients may not have access to a pathologist who specialises in breast cancer, and our AI model could help a generalist pathologist when evaluating breast cancers.”
Training the model
While Northwestern developed the AI software, researchers at the American Cancer Society (ACS) and National Cancer Institute offered expertise of breast cancer epidemiology. The ACS made a unique dataset of breast cancer patients through their Cancer Prevention Studies, which represents patients from over 423 US counties, many who received a diagnosis or care at community medical centres. Most studies only use data from large academic medical centres.
The scientists needed hundreds of thousands of human-generated annotations of cells and tissue structures within digital images of patient tissues to train the AI model. Therefore, they made an international network of medical students and pathologists across several continents, who provided this data through a website over the course of several years to enable the AI model to reliably interpret images of breast cancer tissue.
The scientists are now required to validate the model for clinical use, which coincides with the transition to using digital images for diagnosis at Northwestern Medicine, which will occur over the following three years. They are also working towards developing models for specific types of breast cancer, like HER2-positive. Invasive breast cancer has several varying categories, and the tissue patterns could differ across these categories.
The study was published in Nature Medicine.
Related topics
Artificial Intelligence, Cancer research, Chemotherapy
Related conditions
Cancer Research, HER2-driven breast cancer, invasive breast cancer
Related organisations
American Cancer Society (ACS), National Cancer Institute, Northwestern Medicine, Northwestern University