Capturing the computational abilities of real neurons
Posted: 1 July 2024 | Drug Target Review | No comments yet
A new neural network computational model has been developed, which more closely reflects the abilities of real neurons and could advance AI progress.
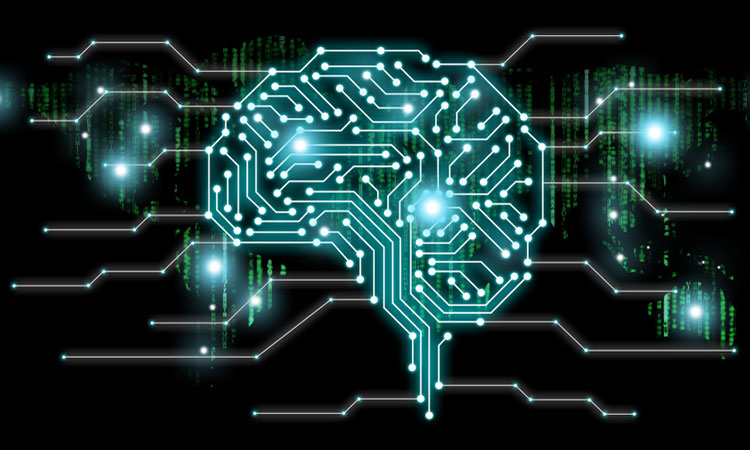
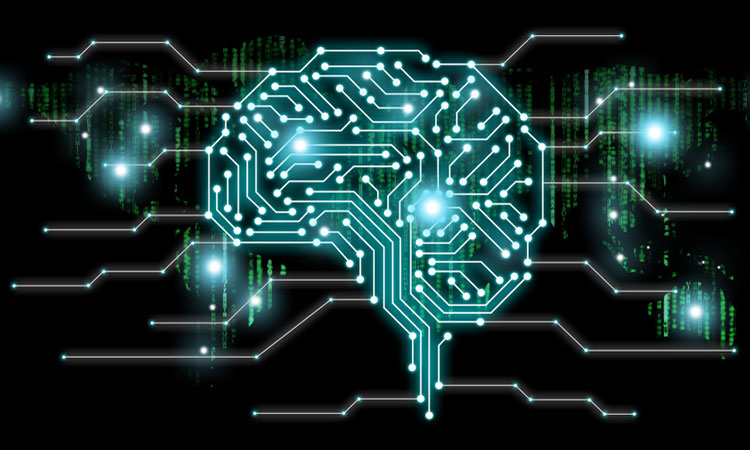
Researchers at the Flatiron Institute’s Center for Computational Neuroscience (CNN) have developed a new neural network computational model that more closely reflects the abilities of real neurons in the human brain, which could greatly advance artificial intelligence (AI) progress.
Almost all neural networks powering AI tools are centred on a 1960s-era computational model of a living neuron. However, the CNN team believe that this does not capture all the computational abilities that real neurons have, as individual neurons have more control over their surroundings than previously considered. Dr Dmitri Chklovskii, group leader at the CNN and senior author of the paper, stated: “Neuroscience has advanced quite a bit in these past 60 years, and we now recognise that previous models of neurons are rather rudimentary…A neuron is a much more complex device — and much smarter — than this overly simplified model.”
Artificial neural networks are built of ordered layers of ‘nodes’, beginning with an input layer of nodes that receives information. Then, there are middle layers of nodes that process the information, and a final output layer of nodes that broadcasts the results. Normally, a node will only pass information to the following layer if the total input it gets from the previous layer’s nodes exceeds a particular threshold. However, when existing artificial neural networks are trained, information travels through a node in only one direction. Furthermore, there is no way for nodes to influence the information they receive from prior nodes.
Contrastingly, the new model regards neurons as small ‘controllers,’ devices that can effect their surroundings based on information gained about those surroundings. Our brain cells could work to control the state of their fellow neurons. Dr Chklovskii thought that this model of a neuron-as-controller could be a substantial move toward enhancing the performance and efficiency of machine learning (ML) applications. He explained: “The current applications can give you wrong answers, or hallucinate, and training them requires a lot of energy; they’re very expensive. There are all these problems that the human brain seems to avoid. If we were to understand how the brain actually this does, we could build better AI.”
As brain circuits are thought to be organised into feedback loops, Dr Chklovskii commented that his kind of feedback control could also be realised by an individual brain cell. The team discovered that a new form of control, called direct data-driven control, is straightforward and efficient enough to be biologically credible as happening in individual cells. “People thought of the brain as a whole or even parts of the brain as being a controller, but no one suggested that a single neuron could do that,” Dr Chklovskii added. “Control is a computationally intensive task. It’s hard to think of a neuron as having enough computational capacity.”
Furthermore, thinking of neurons as mini-controllers explains numerous previously unexplained biological phenomena. Notably, at synapses, there are frequent occasions where a neuron transmits an electrical signal, yet the downstream coupled neuron does receive it. It seems to be directed by chance whether and when the downstream neuron receives the synaptic signal. Although some researchers have attributed this randomness to the nature of small biological systems and not significant to neuron behaviour, the study team discovered that adding noise to their model neuron enabled it to alter to a changing environment. They suggest that this randomness is important in replicating how real neurons function.
Moving forwards, the team aims to analyse types of neurons that do not fit their new model, such as neurons in the retina, which receive direct inputs from the visual environment. Although these neurons may not be able to control their inputs like neurons deeper in the brain can, they could predict their inputs, even if they cannot influence them.
This study was published in Proceedings of the National Academy of Sciences.
Related topics
Artificial Intelligence, Neurons, Technology
Related organisations
Flatiron Institute’s Center for Computational Neuroscience (CNN)
Related people
Dr Dmitri Chklovskii (CNN)