New algorithm could make CRISPR gene editing more precise
Posted: 11 June 2021 | Victoria Rees (Drug Target Review) | No comments yet
Researchers have created an algorithm that can predict the efficiencies of guide RNAs for CRISPR, potentially making it more precise.
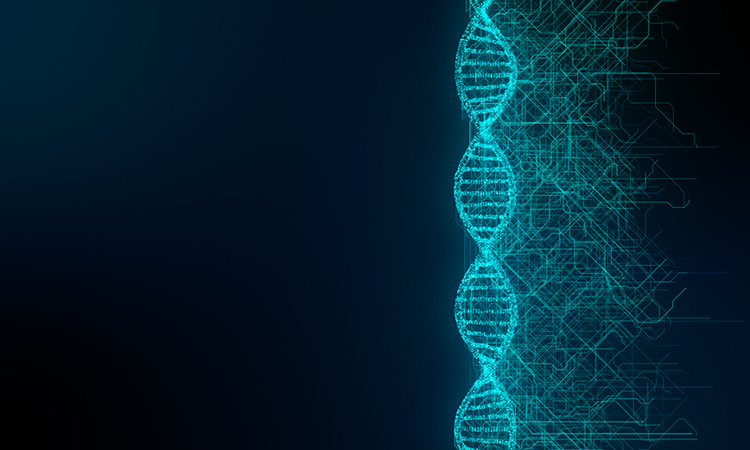
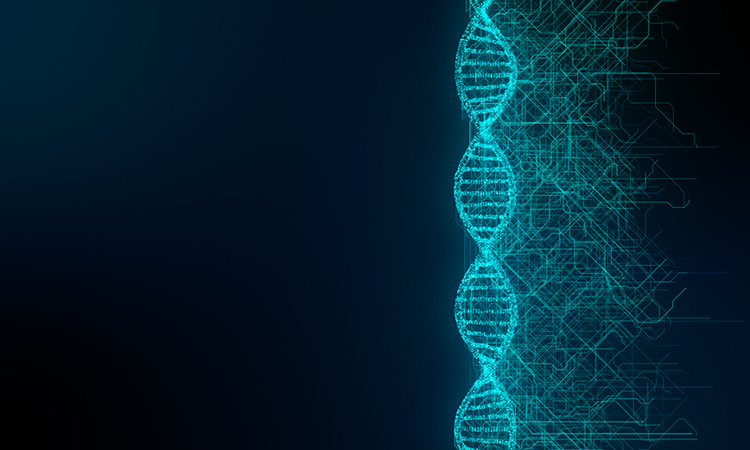
Researchers from the University of Copenhagen, Denmark, have developed an algorithm that could make CRISPR gene editing more precise.
“Typically, there are multiple possible gRNAs and they are not all equally efficient. Therefore, the challenge is to select the few that work with high efficiency and that is precisely what our new method does,” said Associate Professor Yonglun Luo, one of the lead researchers.
The new method is developed from the researchers’ new data and implementation of an algorithm, which gives a prediction on what gRNAs that work most efficiently.
“By combining our own data with publicly available data and including knowledge on the molecular interactions between gRNA, DNA and the CRISPR-Cas9 protein, we have succeeded in developing a better method,” said Professor Jan Gorodkin, another of the lead researchers.
The researchers took their starting point concerning data generation in the concept of having a virus express gRNA and a synthetic target site in one cell at a time. The synthetic target sites have exactly the same DNA sequences as the corresponding target sites in the genome. Thus, these synthetic target sites are used as so-call surrogate target sites to capture the CRISPR-Cas9 editing efficiency. The team generated high quality CRISPR-Cas9 activity for over 10,000 gRNAs.
“In our study, we have quantified the efficiency of gRNA molecules for more than 10,000 different sites. The work was achieved using a massive, high throughput library-based method, which would not be possible with traditional methods,” said Luo.
With this dataset of gRNAs with known efficiencies from low to high, the researchers were able to construct a model that could predict efficiencies of gRNAs which has not been seen before.
“In order to train an algorithm to become precise, one has to have a large dataset. With our library of viruses, we have obtained data that constitutes the perfect starting point for training our deep learning algorithm to predict the efficiency of gRNAs for gene editing. Our new method is more precise than other methods currently available,” said Gorodkin.
The study was published in Nature Communications.
Related topics
Bioinformatics, CRISPR, Genome Editing, Informatics, RNAs
Related organisations
Copenhagen University
Related people
Associate Professor Yonglun Luo, Professor Jan Gorodkin