How AI and LLMs are transforming drug discovery: part 1
Posted: 14 May 2025 | Dr Raminderpal Singh (Hitchhikers AI and 20/15 Visioneers) | No comments yet
AI is reshaping drug discovery – but not without resistance. In this two-part conversation, André França and Eli Pollock share honest insights about the real barriers to AI adoption in life sciences and how embedding domain expertise into AI workflows might be the key to unlocking its full potential.
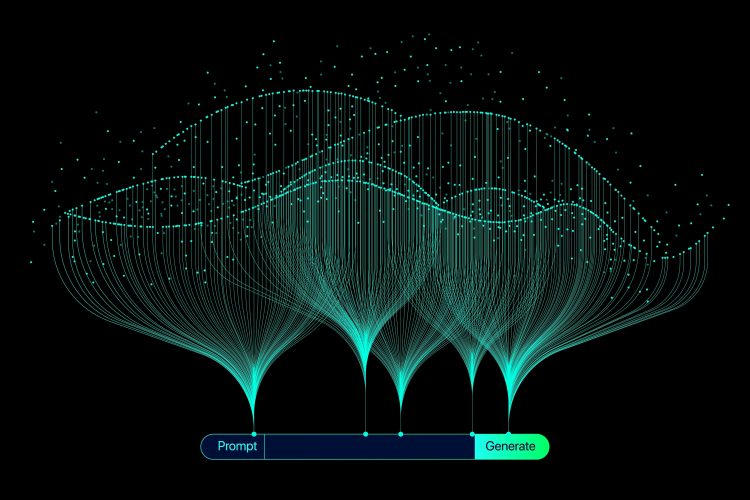
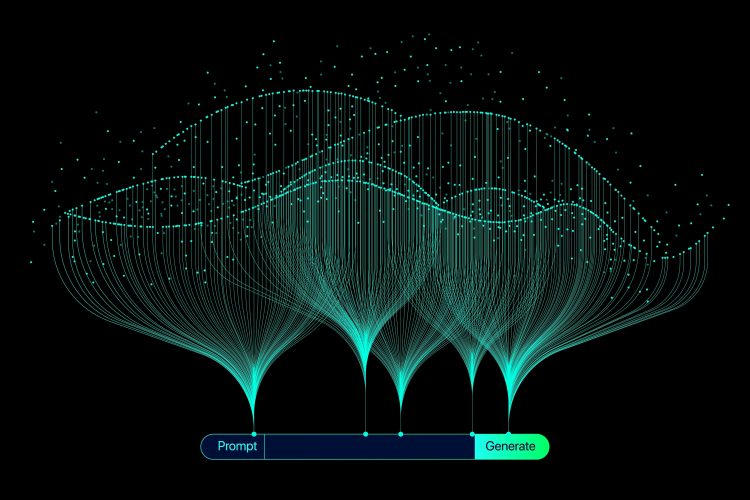
The integration of artificial intelligence (AI) into scientific workflows represents both tremendous opportunity and significant challenges. I recently discussed the subject with two founders at the cutting-edge of this transformation: André França of Ergodic and Eli Pollock of Ontologic. We explored the barriers scientists face when adopting AI tools, contrasting approaches to building AI systems for specialised domains, and their visions for how these technologies will reshape scientific work. In this first article of the two-part series, we cover the challenges of AI adoption in life sciences – drawing lessons from industries that have embraced these tools more rapidly.
Introduction and background
Singh: How do we bring scientists working in drug discovery into the world of AI? What barriers are holding them back? This isn’t about hype or grand promises – it’s about identifying low-hanging fruit and achievable productivity gains.
França: I’m André. I have a background in physics and earned my PhD some time ago. Having worked in finance and AI, I founded Ergodic to help people make better decisions using data and AI.
Pollock: I’m Eli, Co-founder of Ontologic. My background is in computational neuroscience from MIT. At Ontologic, we were sceptical of AI tools for a while and tried not to lean too much into the hype. Recently, we’ve been pursuing AI product initiatives that have made me consider why people might be resistant to AI tools, what AI tools are capable of in drug discovery, and their limitations. It was an interesting time for my studies – AlphaGo happened at the start of my PhD, and I graduated right before ChatGPT blew up the scene. It was a period where no one was quite sure what the next big advancement would be.
AI adoption: inside vs outside life sciences
Singh: André, when you compare the life sciences industry to others, what kinds of excitement or momentum around AI do you see outside the sector that you wish were happening inside life sciences?
França: Outside of life sciences, language models have been employed for about three years, sparking both funding and enthusiasm for experimentation. Just check LinkedIn today and you’ll see 50 percent AI creating a lot of FOMO that drives advances. Therefore, if others are adopting it, I need to get on board to avoid falling behind.
The challenges lie in regulated industries where governance frameworks for standard quantitative models don’t necessarily apply to LLMs.
This is especially true in industries like financial services – particularly hedge funds, which must be first adopters of everything. These organisations not only embraced AI right away for processing information, but they were using many technologies we’re just discovering now, 5-10 years ago. The challenges lie in regulated industries where governance frameworks for standard quantitative models don’t necessarily apply to LLMs. It’s harder to quantify uncertainty and limitations, and harder to constrain usage.
For example, using a language model to review CEO call transcripts is relatively low risk. However, when applied to customer interactions that directly impact financial gain or loss, especially with fiduciary responsibilities involved, trust becomes far more complicated.
The opportunity lies in packaging AI into controlled environments where we understand inputs, possible outputs, and have monitoring systems. One of the biggest blockers of adoption is understanding downstream ramifications – including both the benefits and risks.
Singh: Eli, do you relate to these issues or see things differently in your interactions with scientists in Boston?
Pollock: In drug discovery and biotech, it comes down to different user personas. Much of the hype and FOMO exists on the business side. People use generative AI for productivity tasks like writing emails and formatting presentations. They believe you can throw AI at all data and get cool insights.
The business side wants to incorporate these tools, the scientists who would use them are more sceptical.
However, it’s much harder than that. Working with structured data is complex, and scientists recognise this. While the business side wants to incorporate these tools, the scientists who would use them are more sceptical because they realise that specialised expertise is needed to make sense of their data. They need certainty that generative AI won’t hallucinate. Overcoming that trust barrier is challenging, though opportunities exist to address these problems.
The challenge of specialised expertise
Singh: How do we bring specialised expertise into these deep, research-intensive industries in a way that solves real problems?
Pollock: One exciting breakthrough area is foundation models for domain-specific biological predictions. AlphaFold for protein folding is the most famous example – trained on specific data with impressive performance. I meet startups monthly working on foundation models for whole cells or molecular interactions. These are complex problems requiring more data, but they’re keeping it domain specific.
You need well-structured data, plenty of it, and must incorporate biochemistry and physics assumptions for the systems you’re modelling. Drawing insights without that structure is much harder. Using language models to generally analyse data and generate insights remains challenging.
Singh: So there’s a wave of scientists with deep domain knowledge creating or tuning technologies, embedding scientific context directly into the models. This contrasts with the earlier approach where computer scientists created models that biologists struggled to use effectively. Your point is that embedding expertise in tool creation is a promising path forward?
Pollock: Right, and keeping tools scoped to specific domains with well-structured data. A more general tool that generates scientific insights from varied data would be valuable but is far more challenging to achieve.
Singh: André, your approach seems different – more about casting a wide net and iterating to find use cases?
França: I agree with Eli’s perspective. We’re trying to provide the ingredients necessary to incorporate domain expertise into whatever quantitative models or workflows require AI.
For example, in supply chain, when launching a new product to market with limited sales data, domain expertise becomes critical. Understanding that a red dress will have a different growth profile than a blue suit, and mapping those connections to available data, is important. This helps create a causal chain we can trace for valuable insights.
In drug discovery, identifying targets and compounds depends on your business ontology.
Similarly, in drug discovery, identifying targets and compounds depends on your business ontology. We plan to give users both power and responsibility to make domain knowledge central to their AI workflow.
We’re at an exciting development point for domain-specific models. What we’ve learned from reinforcement learning is that with well-defined quality metrics and a means of scoring language model outputs, we can quickly create systems that transform generic AI tools into domain experts through iteration and training on data with verifier systems you’ve created. With well-structured data and verification methods, you can breed your own specialist model that excels in your domain.
Pollock: I’ve seen companies innovating on the model-building side, but there’s another crucial aspect: high-throughput prediction testing. Automated lab processes are needed to quickly validate predictions. When predictions concern biology – which is inherently complex – it takes time to cultivate cells and can be expensive to generate sufficient data.
What makes this an exciting time is that laboratory automation technology has matured significantly in recent years. These two halves of the problem are coming together.
About the author
Dr Raminderpal Singh is a recognised visionary in the implementation of AI across technology and science-focused industries. He has over 30 years of global experience leading and advising teams, helping early to mid-stage companies achieve breakthroughs through the effective use of computational modelling.
Raminderpal is currently Global Head of AI and GenAI Practice at 20/15 Visioneers and leads the HitchhikersAI.org open-source community. He is also a co-founder of Incubate Bio – a techbio providing a service to life sciences companies who are looking to accelerate their research and lower their wet lab costs through in silico modelling.
Raminderpal has extensive experience building businesses in both Europe and the US. As a business executive at IBM Research in New York, Dr Singh led the go-to-market for IBM Watson Genomics Analytics. He was also Vice President and Head of the Microbiome Division at Eagle Genomics Ltd, in Cambridge. Raminderpal earned his PhD in semiconductor modelling in 1997. He has published several papers and two books and has twelve issued patents. In 2003, he was selected by EE Times as one of the top 13 most influential people in the semiconductor industry.
For more: http://raminderpalsingh.com; http://20visioneers15.com; http://hitchhikersAI.org