Overcoming the complexity of free energy perturbation calculations
Posted: 23 April 2024 | Dr Robert Scoffin (Cresset) | No comments yet
In this article, Dr Robert Scoffin, Chairman and CEO at Cresset, explores FEP and its current challenges, and outlines how continuous advancements and strategic approaches are helping to drive the adoption of FEP methods in drug discovery.
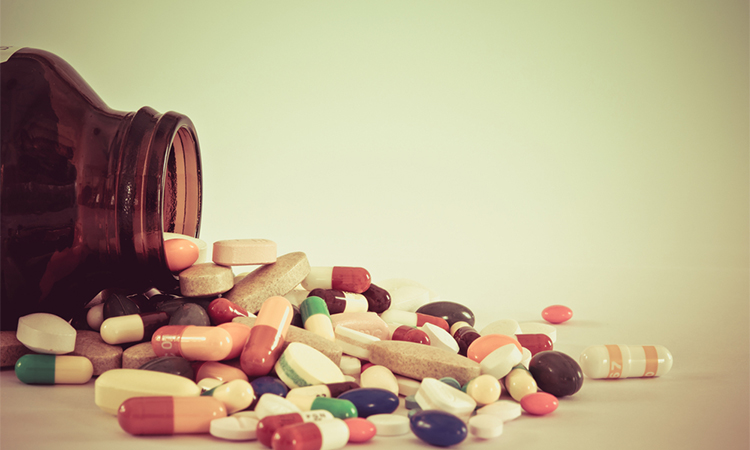
Drug discovery is a complex process, where millions of compounds are investigated throughout the initial hit identification, hit-to-lead, and lead optimisation stages.1 Throughout the discovery process, compounds are synthesised, screened, and eliminated based on their developability. The process of drug discovery is lengthy, and it can take 2-5 years on average to reach the preclinical phase.1
To overcome the bottleneck of synthesising compounds of interest and forming derivatives for lead optimisation, in silico methods are being increasingly used in drug discovery workflows to drive efficiency. The greater use of in silico methods is reflected in the growth of the computer-aided drug design (CADD) market, valued at $3.62 billion in 2023, and forecast to rise at a compound annual growth rate (CAGR) of 11.7 percent, to reach $8.77 billion by 2031.3
One fast-evolving technique to accelerate drug discovery is the use of relative free energy perturbation (FEP) calculations. This method calculates the relative binding affinity of a target library compared to a structurally similar reference, providing binding affinities with accuracy comparable to that of experimental methods. FEP can therefore eliminate poor candidates before ‘wet lab’ synthesis, accelerating the hit-to-lead and lead optimisation stages of the process so that a project can get to the NCE stage more quickly, and with fewer resources. However, the high computing power, associated costs, and complexity of FEP mean it is not as widely adopted within the drug development industry as it should be.
The advantages of utilising FEP in drug discovery
When integrated into later drug discovery stages, such as for enhancing lead optimisation, FEP is an effective tool to help eliminate molecules where changes to the structure of the target negatively impact binding affinity. Having this insight before ‘wet lab’ testing can help introduce significant time and cost savings. As a result, drug developers can expedite the path to clinical and commercial stages, meaning patients will receive essential treatments at accelerated rates. However, to fully recognise the value of FEP in drug discovery, effectively navigating the complexity of FEP calculations is key.
The current limitations of FEP calculations
One obstacle which researchers face when deciding to implement relative FEP approaches is that it can only be used for similar ligands, limiting its applicability especially in early stage hit-to-lead. However, in most cases this is not so limiting, as once a single example of a new chemical series has been synthesised and assayed, it can be used as a template for rapid computational exploration of that new series. The implementation of FEP is also hindered by several other factors, including:
- Knowledge of scripting: FEP interfaces are often command-line run and an understanding of coding is needed in order to successfully implement FEP into a workflow.
- The need for significant computing power: The complexity of FEP calculations, coupled with the need for accuracy and precision, means running these simulations demands significant computational resources.
- Domain expertise: FEP calculations generally require significant expertise from the user, as minor errors in the preparation of the protein or ligands, or in the system setup can cause the calculation to give incorrect or misleading results.
- High cost: The cost of running FEP is high due to the computational resources required and the need for expert users.
To overcome these obstacles, numerous strategies have been developed to ease the use of FEP and enhance approaches to drug discovery.
Strategies to optimise FEP
Several strategies can be leveraged to navigate these difficulties. The first is to introduce a user-friendly interface. Removing the reliance on scripting knowledge broadens the application of FEP to individuals without expertise on using the command line. Therefore, both synthetic and medicinal chemists as well as a wider subset of computational chemists can use this platform to assess potential drug candidates and test binding in silico before ‘wet lab’ methods, thus accelerating development. A further benefit of an advanced user interface is that it can be designed to warn the user of any issues with the setup of the calculation, preventing mistakes that might otherwise mean that the costly simulations need to be re-run.
Another challenge to consider is the computational expense associated with FEP calculations. Achieving accurate free energy estimates requires extensive sampling of the system’s relevant configurations. This typically involves running many simulations, each of which needs to be sufficiently long. Such computations demand access to graphical processing unit (GPU) clusters, which may not be readily available to all researchers. A solution would be to provide the users with access to a cloud-based platform to provide the necessary GPU horsepower in a scalable manner. The up-front costs of running FEP are thus reduced as investment into a high-processing computing system is no longer needed. Licensing costs also need to be factored into the cost/benefit analysis, as the licensing terms of some commercial providers can severely limit the number of GPUs that can be used and hence the overall throughput.
A significant amount of research is being done on other strategies to reduce the computational cost of the calculations. One approach is to use bespoke scheduling strategies such as Adaptive Lambda Scheduling. This algorithm identifies the optimum number of molecular dynamics simulations required for the transformation from reference to target, making sure that the calculation of free energy is accurate. Another approach is to look at options for reducing the size of the system being simulated, trimming off parts of the protein a long way from the binding site. This can reduce the computational costs for large and difficult proteins by a factor of five or more. These and other improvements allow a large number of compounds to be assessed by FEP for a given number of GPUs, giving chemists the ability to test more ideas before committing the best ones to the synthesis pipeline.
The future of FEP
Despite these technological advances, FEP calculations are still very computationally intensive. The move towards a more user-friendly interface and access to cloud computing are increasing FEP accessibility, but further optimisation of FEP is still required to make it more widely used in drug discovery.
Advances are leading to the evolution of absolute FEP, where the binding affinity of non-similar compounds can be accurately calculated. Researchers will therefore have the opportunity to apply free energy calculations to much larger and more diverse compound libraries. In addition, the continued improvements of relative FEP calculations will widen its domain of applicability and further reduce computation times and costs, increasing its applicability to more drug discovery projects and further optimising cost and time savings.
About the author
Dr Robert Scoffin
Chairman and CEO at Cresset
Rob is an expert in the fields of molecular modelling and cheminformatics. His DPhil is in Chemistry from the University of Oxford. Rob is passionate about applying computational methods to help meet medical challenges. He believes drug discovery and design can be streamlined and improved through the use of computational methods, resulting in better drugs being brought to market sooner.
Rob is a Fellow of the Royal Society of Chemistry. Rob joined Cresset as CEO in 2010 and now also serves as Chairman of Cresset. During the time he has been leading the team, Cresset has further developed its software and contract research divisions from a very solid customer base, into a high-growth and profitable business. Rob also serves as co-Chairman for Torx Software, a collaboration between Cresset and Elixir Software. His previous roles include CEO of Amedis and VP, Europe at CambridgeSoft.
References
- FutureWiseResearch: Global Market Research Reports, USA, UK, accessed 23 February 2024, from: https://www.futurewiseresearch.com/healthcare-market-research/Computer-Aided-Drug/14791
- How long does it take to develop a new drug? – MatchTrial, accessed 26 February 2024, from: https://matchtrial.health/en/how-long-does-it-take-to-develop-a-new-drug/
- Hughes, J. P., Rees, S., Kalindjian, S. B., & Philpott, K. L. (2011). Principles of early drug discovery. British journal of pharmacology, 162(6), 1239–1249. https://doi.org/10.1111/j.1476-5381.2010.01127.x
Related topics
Drug Delivery, Drug Development, Drug Discovery
Related organisations
Cresset
Related people
Dr Robert Scoffin (Cresset)