The role of drug transporters in phenotypic screening
Posted: 27 November 2018 | Douglas B. Kell (School of Chemistry - The Manchester Institute of Biotechnology), Marina Wright Muelas (School of Chemistry - The Manchester Institute of Biotechnology), Philip J. Day (The Manchester Institute of Biotechnology & Faculty of Biology - Medicine and Health - The University of Manchester), Steve O’Hagan (School of Chemistry - The Manchester Institute of Biotechnology) | No comments yet
The relative failure of molecular target-based drug discovery has led to a return to phenotypic screening. Targets that are intracellular necessitate their drug ligands to pass through plasma membranes, where the protein:lipid ratio is often 3:1 by mass and at least 1:1 by area. The widespread view that most of such transmembrane drug transport occurs through the phospholipid bilayer portion is incorrect: drugs must and do exploit transmembrane protein transporters that have been selected or evolved for the uptake of intermediary metabolites and natural products.
In some cases, the potency of extracellular drugs depends more or less exclusively on the activity of the transporters necessary to get them to their targets. Such considerations can explain the heterogeneous nature of drug efficacy and toxicity, and hence their roles in attrition, which highlights the need to consider drug transporters as a core component of the pipeline in both target-based and phenotypic drug discovery.
Following the basic recognition in chemical pharmacology that drugs must, in fact, have receptors,1 there arose two fundamental approaches to drug discovery. These can be referred to as phenotypic (function-first) and hypothesis-driven (gene- or target-based) (Figure 1). They are equivalent to the data-driven versus hypothesis-dependent approaches to science2 (Figure 1A), or to what are equivalently termed forward and reverse (chemical) genetics/genomics3,4 (Figure 1B). They can also be seen to differ in terms of the intellectual requirements of pre- and post-genomic research.5 Such diagrams (Figure 1) at once recognise two aspects: (i) that in a standard experimental programme there is (or should be) an iterative interplay between the gathering of data, their interpretation, and the design of follow-up experiments; and (ii) that different programmes can and do give dramatically different emphases to the two arcs represented in Figure 1B.
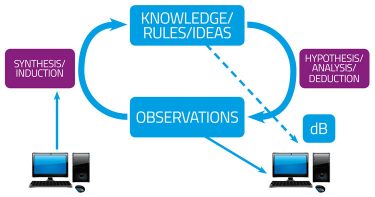
Figure 1A: The cycle of knowledge
Classical drug discovery was entirely phenotypic: substances would have bioeffects that cured diseases, and therapies could be developed and tested (often then in whole animals) without any knowledge of their mechanisms; such knowledge, if required, could come later. By contrast, the advent of molecular cloning, recombinant protein production and (possibly surprisingly) genomics itself led to the desire to find molecular ‘targets’ whose activity could be assayed and modulated using very high-throughput screens in vitro.
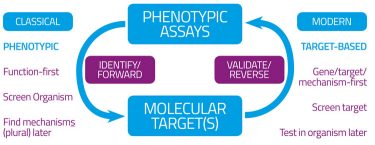
Figure 1B: Forward and reverse (chemical) genomics and drug discovery
Of course (Figure 1B) this would tell you that a particular molecule was highly potent at binding to and affecting (usually inhibiting) such a protein (receptor) but equally could tell nothing of whether off-target effects could occur (as they inevitably do6) and – more pertinently here – whether the drug might be able to reach an appropriate compartment such that it might have access to its targets, let alone whether it might also be toxic.
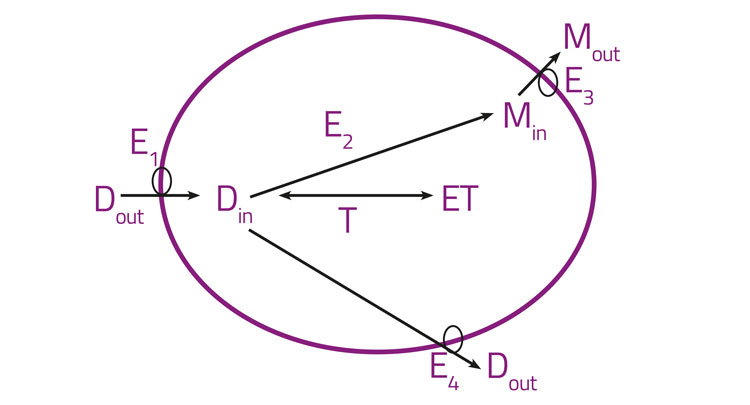
Figure 2: Orally active drugs crossing epithelial cell layers
Specifically, orally active drugs must necessarily cross epithelial cell layers and any drugs with intracellular binding targets must necessarily enter cells. Figure 2 gives a high-level illustration of these features, lumping together all the import transporters (E1), drug metabolising enzymes (E2), and the efflux transporters for the drug (E3) and metabolites (E4). Binding sites (T, targets) for the free drug, as well as metabolisers and effluxers, lower its intracellular concentration and hence its availability. Many textbooks and reviews7-11 imply that there is an alternative (and indeed major) pathway of transmembrane drug transport that is often (misleadingly)12,13 referred to as ‘passive’ and is said to occur by simple diffusion through the phospholipid bilayer, mainly as a function of lipophilicity as assessed via log P or log D. Perhaps surprisingly, this is simply an assumption without any concrete evidence in intact cells, as the involvement of any such bilayer-mediated pathway has never actually been measured directly in real biological cells12,14 (and it would be almost impossible to do so). Years of evolution have almost certainly ensured that cells do not, in fact, tend to take up potentially toxic xenobiotics in such a way at substantial rates. Consequently, the evidence is, in our view,3,12-22 overwhelmingly to the effect that the transmembrane transport of pharmaceutical drugs occurs essentially exclusively via protein transporters (SLCs23), that have been selected during natural evolution for the transport of both intermediary metabolites24-27 and natural products.28,29 The detailed and extensive evidence is given in the papers cited,3,12-22 but the recognition that ‘phospholipid bilayer transport is negligible’ (PBIN)12 in intact biological cells clearly accounts for the very heterogeneous uptake of drugs in different cells,30 tissues, individual organisms and species (including or otherwise via the blood-brain barrier). The same principles (bilayer transport is negligible and transmembrane transport is transporter-mediated only) can be exploited for drug targeting.31-34 Consequently, no such phospholipid bilayer transport is considered to occur in intact biological cells, and is therefore not shown in Figure 2. In addition, there are many drugs that are known to have transporters whose molecular identity has not yet been uncovered: the natural product cocaine35 and the antipsychotic clozapine36 are just two examples.
Attrition
Attrition37-41 is a term used to describe the fact that even starting from phase 1 (‘first into humans’) presently some 92 percent of drug candidates fail to make it to market. This is of course extraordinarily costly. It has not improved since the move from classical to target-based strategies, and frankly, it is highly unlikely to – at least until the number of targets increases significantly as per systems pharmacology. The reasons are multiple and complex;38-44 mainly lack of efficacy or palpable toxicity. In our view, chief among them is the failure to recognise that the demonstrably heterogeneous distribution of drug transporters in different cells and tissues means that some tissues may have no uptake transporters (hence lack of efficacy) or they may have all too much of such activity and accumulate the drug to high levels (hence toxicity).
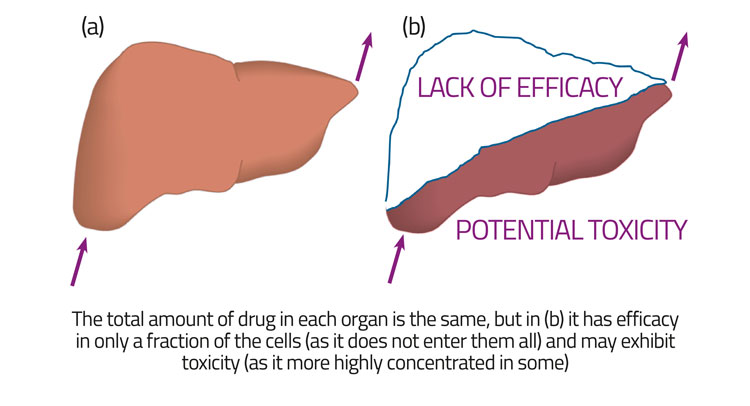
Figure 3: Heterogeneous distributions of a drug in an organ can lead to a lack of efficacy while retaining the same gross PK/PD
For fundamental reasons,45,46 bulk measurements at the level of the organ or tissue cannot detect or discriminate this, a point illustrated in Figure 3. Importantly, phenotypic screening overcomes all of this, in the sense that one can at least hope to detect both efficacy and lack of toxicity in something closer to in vivo conditions – including the need for transmembrane transport – and consequently most successful drugs nowadays are discovered via early phenotypic screening.3,47-51
SLCs – the family of human SoLuteCarriers
Some 10 percent of the human genome encodes transporters and related proteins; they are the second largest component of the membrane proteome52 and an area of emerging excitement.53 Hediger and colleagues have helpfully systematised the taxonomy of human transporters;23 at the beginning of 2018 there were some 52 families,30 while the present total (maintained at http://www.bioparadigms.org/slc/intro.htm) is 65. These encode over 400 proteins. In addition, there are some 48 ABC- (ATP-binding cassette-) type transporters, mainly involved in efflux.54
The role of transporters in phenotypic screening
Space limitations mean that we must be selective in our illustrations, but because carrier-mediated transport is necessarily performed by genetically encoded protein transporters it is possible to modify the activity of named transporters by genetic or pharmacological means and observe the effects of such modifications, both on the consequent uptake of drugs and on their effects. This uptake may be measured directly (eg, using radioisotopes, fluorimetrically,55,56 or by mass spectrometry) but may also be inferred by the consequent bioactive effects of drugs that are taken up. The most extreme such effect, pertinent for instance in anti-cancer drug discovery, is cell death. The idea,57 then, is that cells that lack the transporters for toxic substrates are more resistant to those substances, and can be selected accordingly in competition experiments; analysis of the ‘winners’ can thereby indicate the relevant transporters. Transporters themselves can of course also be drug targets,58,59 although this is not a focus of the present review.
The modern availability of systematic collections of genetically modified strains (and the ease of their creation) makes such studies relatively straightforward.60,61 Thus, we found concentrations of drugs that lowered the growth rate of yeast cells by 90 percent and used57 the yeast knockout collection62,63 to assess those cells that were more resistant to cytotoxic drugs than were the wild-type strains. Typically (18/26 drugs tested), we could find between one and six knockouts that were more resistant to the drug than was the wild-type, although there were eight occasions when no such knockout had this effect. The interpretation of the latter is that when there are a great many possible uptake transporters the removal of just one does not result in an obviously sensitive phenotype. This is as expected given the theory of metabolic control analysis. 64,65
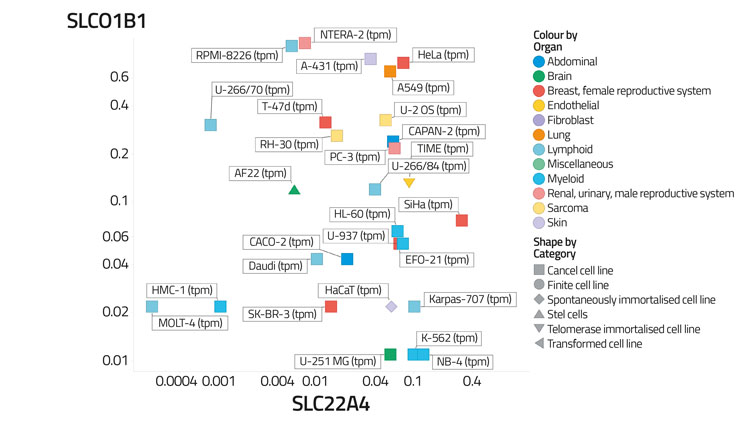
Figure 4A: Differential transporter expression in cell lines
A particularly good example in the context of drug discovery came from a set of comparable experiments in mammalian cells reported by Superti-Furga and colleagues.66 They used a (near-)haploid cell line plus a ‘gene trap’ (retrovirus) that can insert itself into any gene.67 When such cells were exposed to normally lethal concentrations of the candidate anticancer drug sepantronium bromide (YM155), surviving cells were always found to have the retrovirus inserted into a gene encoding the solute carrier SLC35F2 (its ‘natural’ substrate is unknown). Such cells typically required 500-fold greater concentrations of YM155 to exhibit the same levels of toxicity, implying that at most 0.5 percent of the transmembrane flux could go via other routes (including the bilayer), which we certainly regard as negligible. Indeed, when studied in a series of cell lines, the toxicity varied over four orders of magnitude, in line with the expression level of the transcript for SLC35F2.66
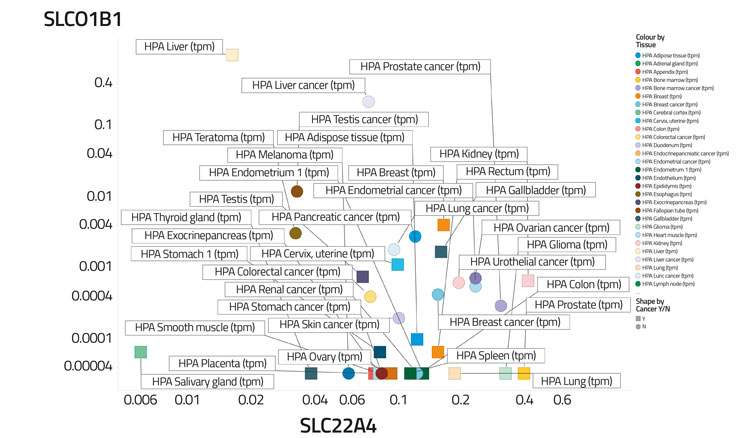
Figure 4B: Differential transporter expression in tissues
In a recent study,30 based on certain data,68 we assessed the variability of transporter expression in some 59 tissues and 56 cell lines; transporter expression levels typically varied to a significantly greater degree than did those of most other protein types,30 almost always by 100-fold and often by 10,000 times. We illustrate this in Figure 4 by showing the covariation of the distribution of two transporters (SLCO1B1, the main ‘statin’ transporter,69 and SLC22A4, a transporter for ergothioneine70,71) among 56 cell lines (Figure 4A) and 589 tissues (Figure 4B), that serves to illustrate how very heterogeneous their expression levels can be, often in cells from the same kinds of tissue. Within a tissue, methods such as imaging mass spectrometry show72 that they can be even greater. Data such as those in the present and previous paragraph indicate that predicting the phenotypic activity (toxicity) of drugs such as YM155 in the absence of knowledge of the transporters involved and their expression levels would be a hazardous enterprise indeed.
Conclusions
There is a widespread and erroneous belief that most drugs can enter and exit cells ‘passively’ (ie, equilibrate) via a phospholipid bilayer common to them all. Similarly, the word ‘passive’ has often been misused to conflate the two ideas – one thermodynamic (equilibrative rather than concentrative) and one mechanistic (bilayer versus transporter-mediated transport). In fact, the bilayer route is negligible (or there is no evidence for it in intact biological cells). Consequently, to assess the potency of drugs with intracellular targets in phenotypic screens, we need first to know the transporters that they use to gain entry to (and exit from) the cells, and then their expression levels and activities (just as in any other systems biology programme).73-75 A failure to do so makes it much harder to understand the highly varying potencies (or toxicities) of drugs in different cells. By contrast, such knowledge can be of immense value, not least in targeting specific drugs to specific tissues – something that offers great hope for improving the therapeutic indices of potentially toxic drugs.31
Acknowledgments
We thank the BBSRC for financial support (grant BB//P009042/1).
Biography
Douglas Kell is a former Chief Executive of BBSRC and has a CBE for services to science and research. He is currently Research Chair in Bioanalytical Science at the University of Manchester and in December 2018 will take up the position of Chair in Systems Biology at the University of Liverpool. He is also Associated Scientific Director at the Novo Nordisk Foundation Centre for Biosustainability at the Technical University of Denmark.
Douglas has been a pioneer in many areas of computational biology and experimental metabolomics, including the use of evolutionary, closed-loop methods for optimisation. He also contributed to the discovery of the first bacterial cytokine, currently on trial as part of a vaccine against tuberculosis.
He has published over 400 scientific papers with >26,000 citations (H-index 84) in WoK and >46,000 citations (H-index 105) in Google Scholar.
References
- Bowman WC, Rand MJ, West GB. Textbook of Pharmacology. Oxford: Blackwell; 1967.
- Kell DB, Oliver SG. Here is the evidence, now what is the hypothesis? The complementary roles of inductive and hypothesis-driven science in the post-genomic era. Bioessays. 2004;26:99-105.
- Kell DB. Finding novel pharmaceuticals in the systems biology era using multiple effective drug targets, phenotypic screening, and knowledge of transporters: where drug discovery went wrong and how to fix it. FEBS J. 2013;280:5957-80.
- Kell DB, Goodacre R. Metabolomics and systems pharmacology: why and how to model the human metabolic network for drug discovery. Drug Disc Today. 2014;19(2):171-82.
- Kell DB, Darby RM, Draper J. Genomic computing: explanatory analysis of plant expression profiling data using machine learning. Plant Physiol. 2001;126:943-51.
- Mestres J, Gregori-Puigjané E, Valverde S, Solé RV. The topology of drug-target interaction networks: implicit dependence on drug properties and target families. Mol Biosyst. 2009 Sep;5(9):1051-7.
- Waring MJ. Lipophilicity in drug discovery. Expert Opinion on Drug Discovery. 2010 Mar;5(3):235-48.
- van De Waterbeemd H, Testa B, editors. Drug Bioavailability: Estimation of Solubility, Permeability, Absorption and Bioavailability. Weinheim: Wiley; 2009.
- Krogsgaard-Larsen P, Liljefors T, Madsen U, editors. A textbook of drug design and development, 2nd Ed. New York: Harwod Academic Publishers; 1996.
- Cao X, Yu LX, Sun D. Drug Absorption Principles. In: Krishna R, Yu L, editors. Biopharmaceutics Applications in Drug Development. New York: Springer; 2006. p. 75-100.
- Avdeef A. Absorption and drug development: solubility, permeability and charge state. 2nd ed. New York: Wiley; 2012.
- Kell DB, Oliver SG. How drugs get into cells: tested and testable predictions to help discriminate between transporter-mediated uptake and lipoidal bilayer diffusion. Front Pharmacol. 2014;5:231.
- Kell DB. What would be the observable consequences if phospholipid bilayer diffusion of drugs into cells is negligible? Trends Pharmacol Sci. 2015;36(1):15-21.
- Kell DB. How drugs pass through biological cell membranes – a paradigm shift in our understanding? Beilstein Magazine. 2016;2(5):http://www.beilstein-institut.de/download/628/09_kell.pdf.
- Dobson PD, Kell DB. Carrier-mediated cellular uptake of pharmaceutical drugs: an exception or the rule? Nat Rev Drug Disc. 2008;7:205-20.
- Dobson PD, Patel Y, Kell DB. “Metabolite-likeness” as a criterion in the design and selection of pharmaceutical drug libraries. Drug Disc Today. 2009;14:31-40.
- Dobson P, Lanthaler K, Oliver SG, Kell DB. Implications of the dominant role of cellular transporters in drug uptake. Curr Top Med Chem. 2009;9:163-84.
- Kell DB, Dobson PD. The cellular uptake of pharmaceutical drugs is mainly carrier-mediated and is thus an issue not so much of biophysics but of systems biology. In: Hicks MG, Kettner C, editors. Proc Int Beilstein Symposium on Systems Chemistry. Berlin: Logos Verlag; 2009. p. 149-68 – http://www.beilstein-institut.de/Bozen2008/Proceedings/Kell/Kell.pdf.
- Kell DB, Dobson PD, Oliver SG. Pharmaceutical drug transport: the issues and the implications that it is essentially carrier-mediated only. Drug Disc Today. 2011;16(15/16):704-14.
- Kell DB, Dobson PD, Bilsland E, Oliver SG. The promiscuous binding of pharmaceutical drugs and their transporter-mediated uptake into cells: what we (need to) know and how we can do so. Drug Disc Today. 2013;18(5/6):218-39.
- Kell DB. The transporter-mediated cellular uptake of pharmaceutical drugs is based on their metabolite-likeness and not on their bulk biophysical properties: Towards a systems pharmacology Perspect Sci. 2015;6:66-83.
- Kell DB. Implications of endogenous roles of transporters for drug discovery: hitchhiking and metabolite-likeness. Nat Rev Drug Disc. 2016;15(2):143-4.
- Hediger MA, Clemencon B, Burrier RE, Bruford EA. The ABCs of membrane transporters in health and disease (SLC series): Introduction. Mol Aspects Med. 2013 Apr;34(2-3):95-107.
- O’Hagan S, Swainston N, Handl J, Kell DB. A ‘rule of 0.5′ for the metabolite-likeness of approved pharmaceutical drugs. Metabolomics. 2015;11(2):323-39.
- O’Hagan S, Kell DB. Understanding the foundations of the structural similarities between marketed drugs and endogenous human metabolites. Front Pharmacol. 2015;6:105.
- O’Hagan S, Kell DB. MetMaxStruct: a Tversky-similarity-based strategy for analysing the (sub)structural similarities of drugs and endogenous metabolites. Front Pharmacol. 2016;7:266.
- O’Hagan S, Kell DB. Analysis of drug-endogenous human metabolite similarities in terms of their maximum common substructures. J Cheminform. 2017;9:18.
- O’Hagan S, Kell DB. Consensus rank orderings of molecular fingerprints illustrate the ‘most genuine’ similarities between marketed drugs and small endogenous human metabolites, but highlight exogenous natural products as the most important ‘natural’ drug transporter substrates. ADMET & DMPK. 2017;5(2):85-125.
- O’Hagan S, Kell DB. Analysing and navigating natural products space for generating small, diverse, but representative chemical libraries. Biotechnol J. 2018;13(1):1700503.
- O’Hagan S, Wright Muelas M, Day PJ, Lundberg E, Kell DB. GeneGini: assessment via the Gini coefficient of reference ‘‘housekeeping’’ genes and diverse human transporter expression profiles Cell Syst. 2018;6(2):230-44.
- Grixti J, O’Hagan S, Day PJ, Kell DB. Enhancing drug efficacy and therapeutic index through cheminformatics-based selection of small molecule binary weapons that improve transporter-mediated targeting: a cytotoxicity system based on gemcitabine. Front Pharmacol. 2017;8:155.
- Pfefferkorn JA, Litchfield J, Hutchings R, Cheng XM, Larsen SD, Auerbach B, et al. Discovery of novel hepatoselective HMG-CoA reductase inhibitors for treating hypercholesterolemia: A bench-to-bedside case study on tissue selective drug distribution. Bioorg Med Chem Lett. 2011 May 1;21(9):2725-31.
- Pfefferkorn JA, Guzman-Perez A, Litchfield J, Aiello R, Treadway JL, Pettersen J, et al. Discovery of (S)-6-(3-cyclopentyl-2-(4-(trifluoromethyl)-1H-imidazol-1-yl)propanamido)nicotini c acid as a hepatoselective glucokinase activator clinical candidate for treating type 2 diabetes mellitus. J Med Chem. [In Vitro]. 2012 Feb 9;55(3):1318-33.
- Pfefferkorn JA. Strategies for the design of hepatoselective glucokinase activators to treat type 2 diabetes. Expert Opin Drug Discov. 2013 Mar;8(3):319-30.
- Chapy H, Smirnova M, Andre P, Schlatter J, Chiadmi F, Couraud PO, et al. Carrier-Mediated Cocaine Transport at the Blood-Brain Barrier as a Putative Mechanism in Addiction Liability. Int J Neuropsychopharmacol. 2014 Jan;18(1):1-10.
- Dickens D, Radisch S, Chiduza GN, Giannoudis A, Cross MJ, Malik H, et al. Cellular uptake of the atypical antipsychotic clozapine is a carrier-mediated process. Mol Pharm. 2018 Jun 26.
- Arrowsmith J, Miller P. Trial watch: phase II and phase III attrition rates 2011-2012. Nat Rev Drug Disc. [News]. 2013 Aug;12(8):569.
- Empfield JR, Leeson PD. Lessons learned from candidate drug attrition. IDrugs. 2010 Dec;13(12):869-73.
- Kola I, Landis J. Can the pharmaceutical industry reduce attrition rates? Nat Rev Drug Discov. 2004 Aug;3(8):711-5.
- Leeson PD. Molecular inflation, attrition and the rule of five. Adv Drug Deliv Rev. 2016 Jun 1;101:22-33.
- Waring MJ, Arrowsmith J, Leach AR, Leeson PD, Mandrell S, Owen RM, et al. An analysis of the attrition of drug candidates from four major pharmaceutical companies. Nat Rev Drug Discov. 2015 Jul;14(7):475-86.
- Khanna I. Drug discovery in pharmaceutical industry: productivity challenges and trends. Drug Disc Today. [Review]. 2012 Oct;17(19-20):1088-102.
- Leeson PD, Empfield JR. Reducing the risk of drug attrition associated with physicochemical properties. Annu Rep Med Chem. 2010;45:393-407.
- Kola I. The state of innovation in drug development. Clin Pharmacol Ther. 2008 Feb;83(2):227-30.
- Kell DB, Ryder HM, Kaprelyants AS, Westerhoff HV. Quantifying heterogeneity: Flow cytometry of bacterial cultures. Antonie van Leeuwenhoek. 1991;60:145-58.
- Davey HM, Kell DB. Flow cytometry and cell sorting of heterogeneous microbial populations: the importance of single-cell analysis. Microbiol Rev. 1996;60:641-96.
- Prior M, Chiruta C, Currais A, Goldberg J, Ramsey J, Dargusch R, et al. Back to the future with phenotypic screening. ACS Chem Neurosci. 2014 Jul 16;5(7):503-13.
- Zheng W, Thorne N, McKew JC. Phenotypic screens as a renewed approach for drug discovery. Drug Discov Today. 2013 Nov;18(21-22):1067-73.
- Swinney DC. Phenotypic vs. Target-Based Drug Discovery for First-in-Class Medicines. Clin Pharmacol Ther. 2013 Apr;93(4):299-301.
- Swinney DC. Opportunities for phenotypic screening in drug discovery. Drug Disc World. 2014;15(4):33-42.
- Swinney DC, Xia S. The discovery of medicines for rare diseases. Future Med Chem. 2014 Jun;6(9):987-1002.
- Almén MS, Nordström KJ, Fredriksson R, Schiöth HB. Mapping the human membrane proteome: a majority of the human membrane proteins can be classified according to function and evolutionary origin. BMC Biol. [Research Support, Non-U.S. Gov’t]. 2009;7:50.
- César-Razquin A, Snijder B, Frappier-Brinton T, Isserlin R, Gyimesi G, Bai X, et al. A call for systematic research on solute carriers. Cell. 2015 Jul 30;162(3):478-87.
- Chen Z, Shi T, Zhang L, Zhu P, Deng M, Huang C, et al. Mammalian drug efflux transporters of the ATP binding cassette (ABC) family in multidrug resistance: A review of the past decade. Cancer Lett. 2016 Jan 1;370(1):153-64.
- Tegos GP, Evangelisti AM, Strouse JJ, Ursu O, Bologa C, Sklar LA. A high throughput flow cytometric assay platform targeting transporter inhibition. Drug Disc Today Technol. 2014 Jun;12:e95-e103.
- Fardel O, Le Vee M, Jouan E, Denizot C, Parmentier Y. Nature and uses of fluorescent dyes for drug transporter studies. Expert Opin Drug Metab Toxicol. 2015 Jun 7;11(8):1233-51.
- Lanthaler K, Bilsland E, Dobson P, Moss HJ, Pir P, Kell DB, et al. Genome-wide assessment of the carriers involved in the cellular uptake of drugs: a model system in yeast. BMC Biol. 2011;9:70.
- Viereck M, Gaulton A, Digles D. Insights into Transporter Classifications: an Outline of Transporters as Drug Targets. Transporters as Drug Targets. 2017;70:1-20.
- Lin L, Yee SW, Kim RB, Giacomini KM. SLC transporters as therapeutic targets: emerging opportunities. Nat Rev Drug Discov. 2015 Aug;14(8):543-60.
- Stovicek V, Holkenbrink C, Borodina I. CRISPR/Cas system for yeast genome engineering: advances and applications. FEMS Yeast Res. 2017 May 15.
- Zhou Y, Zhu S, Cai C, Yuan P, Li C, Huang Y, et al. High-throughput screening of a CRISPR/Cas9 library for functional genomics in human cells. Nature. 2014 May 22;509(7501):487-91.
- Giaever G, Flaherty P, Kumm J, Proctor M, Nislow C, Jaramillo DF, et al. Chemogenomic profiling: identifying the functional interactions of small molecules in yeast. Proc Natl Acad Sci U S A. 2004 Jan 20;101(3):793-8.
- Giaever G, Nislow C. The yeast deletion collection: a decade of functional genomics. Genetics. 2014 Jun;197(2):451-65.
- Kacser H, Burns JA. The molecular basis of dominance. Genetics. 1981;97:639-66.
- Kell DB, Westerhoff HV. Metabolic control theory: its role in microbiology and biotechnology. FEMS Microbiol Rev. 1986;39:305-20.
- Winter GE, Radic B, Mayor-Ruiz C, Blomen VA, Trefzer C, Kandasamy RK, et al. The solute carrier SLC35F2 enables YM155-mediated DNA damage toxicity. Nat Chem Biol. 2014;10:768-73.
- Bürckstümmer T, Banning C, Hainzl P, Schobesberger R, Kerzendorfer C, Pauler FM, et al. A reversible gene trap collection empowers haploid genetics in human cells. Nat Methods. 2013;10(10):965-71.
- Thul PJ, Åkesson L, Wiking M, Mahdessian D, Geladaki A, Ait Blal H, et al. A subcellular map of the human proteome. Science. 2017 May 26;356(6340).
- Hagenbuch B, Stieger B. The SLCO (former SLC21) superfamily of transporters. Mol Aspects Med. 2013 Apr;34(2-3):396-412.
- Gründemann D, Harlfinger S, Golz S, Geerts A, Lazar A, Berkels R, et al. Discovery of the ergothioneine transporter. Proc Natl Acad Sci. 2005 Apr 5;102(14):5256-61.
- Kerley RN, McCarthy C, Kell DB, Kenny LC. The potential therapeutic effects of ergothioneine in pre-eclampsia. Free Radic Biol Med. 2018;117:145-57.
- Römpp A, Guenther S, Takats Z, Spengler B. Mass spectrometry imaging with high resolution in mass and space (HR2 MSI) for reliable investigation of drug compound distributions on the cellular level. Anal Bioanal Chem. [Evaluation Studies Research Support, Non-U.S. Gov’t]. 2011 Jul;401(1):65-73.
- Kell DB, Knowles JD. The role of modeling in systems biology. In: Szallasi Z, Stelling J, Periwal V, editors. System modeling in cellular biology: from concepts to nuts and bolts. Cambridge: MIT Press; 2006. p. 3-18.
- Kell DB. Metabolomics, modelling and machine learning in systems biology: towards an understanding of the languages of cells. The 2005 Theodor Bücher lecture. FEBS J. 2006;273:873-94.
- Kell DB. Systems biology, metabolic modelling and metabolomics in drug discovery and development. Drug Disc Today. 2006;11(23/24):1085-92.
Related topics
Biopharmaceuticals, Drug Discovery, Ligands