Unravelling cell biology through artificial intelligence
Posted: 18 August 2022 | Ria Kakkad (Drug Target Review) | No comments yet
The AI algorithm was able to predict the presence and the location of nuclei in more than 8,000 cells.
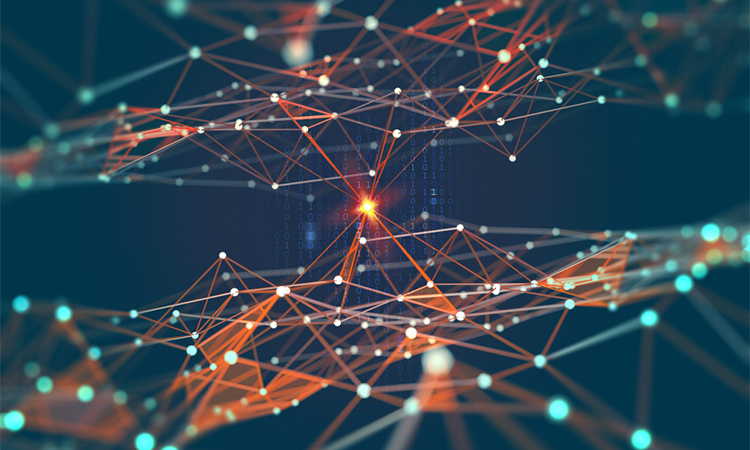
Scientists from the Singapore University of Technology and Design (SUTD) and the National University of Singapore and the Nanyang Technological University, Singapore have used artificial intelligence (AI) to demonstrate a correlation between cytoskeleton organisation and nuclear position. The study was recently published in PLOS.
To ensure that the study’s parameters would not be limited by human conceptualisation, they developed a unique generative algorithm to interpret the cytoskeleton of eukaryotic cells using qualitative data, without telling the system what it was observing and how to measure it.
“We separated the information related to the nucleus and the fibres in independent databases of images, ensuring that there was not any information about the nucleus found in the images of the fibres, so that the system could not cheat. Then we trained the system to find the location of the nucleus using only information specific to fibres. To do so, the system had to take the qualitative data and figure out on its own if there was a relation between the organisation of the fibres and the position of the nucleus. This forced the programme to find the parameters defining the system, free from human interpretation and predefined concepts,” Associate Professor Javier G. Fernandez explained.
The algorithm was able to successfully predict the presence and the location of the nuclei in more than 8,000 cells, with almost half of those predictions resulting in a deviation of less than 1 μm from their exact position. This demonstrated, with astounding significance, the hypothesis of a deterministic relation between the arrangements of the actin filaments and the position of the nucleus, one of the most basic relations in cell biology, which the researchers argue that this has also resulted in an epistemological outcome.
Missed the news? Researchers have developed a machine learning method to quantitatively analyse and compare microscopy images of proteins.
READ MORE
“This study has transformed the way we think about adapting our scientific research methods to allow machine learning to not just be used as a tool to analyse data, but to also interpret reality. For the inherently complex systems in biology, this will undoubtedly accelerate the next technological revolution – the ‘biologisation’ of technology. This will enable the complexities and intricacies of biological systems to be truly unravelled and dominated using machine learning,” concluded Fernandez.
Related topics
Artificial Intelligence, Bioinformatics, Cell Cultures, Imaging