Using machine learning to guide and enhance bioengineering
Posted: 3 November 2020 | Hannah Balfour (Drug Target Review) | 1 comment
The Automated Recommendation Tool (ART) uses machine learning to accelerate the development of cells for specified goals, eg, bioprocessing and bioproduction.
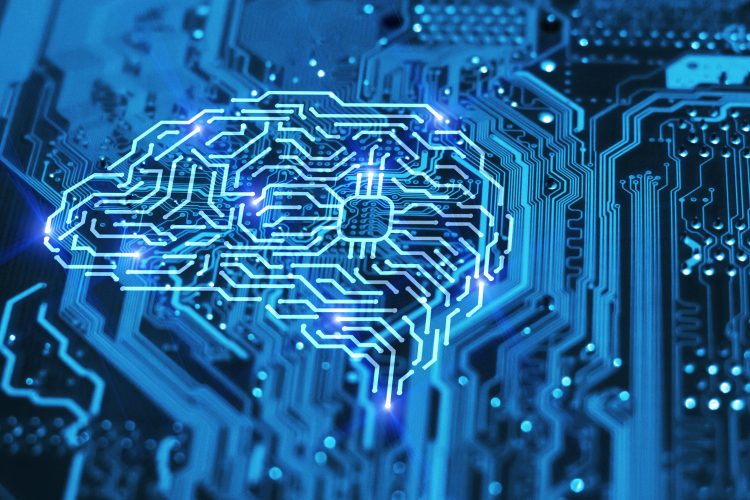
Scientists have developed a new tool that adapts machine learning (ML) algorithms to the needs of synthetic biology. According to the team, the innovative approach could speed up the process of bioengineering, revolutionising the field.
Synthetic biology allows scientists to design biological systems to specification, examples include engineering a microbe or plant to produce a metabolite, drug or reagent (ie, bioproduction). However, conventional methods of bioengineering are slow and laborious, predominantly relying on trial and error.
To enhance this process, scientists at the Department of Energy’s Lawrence Berkeley National Laboratory (Berkeley Lab), US, have developed a tool to guide bioengineering systematically. The team said that with their innovation scientists would not need to spend years developing a meticulous understanding of each part of a cell and what it does in order to manipulate it; instead, with a limited set of training data, the ML algorithms are able to predict how changes in a cell’s DNA or biochemistry will affect its behaviour. From these predictions the system makes recommendations for the next engineering cycle along with probabilistic predictions for attaining the desired goal.
Hector Garcia Martin, leader of the project and a researcher in Berkeley Lab’s Biological Systems and Engineering (BSE) Division, said: “The possibilities are revolutionary. Right now, bioengineering is a very slow process. It took 150 person-years to create the anti-malarial drug, artemisinin. If you can create new cells to specification in a couple weeks or months instead of years, you could really revolutionise what you can do with bioengineering.”
Garcia Martin worked in collaboration with BSE data scientist Tijana Radivojevic and an international group of researchers to develop and demonstrate their patent-pending algorithm called the Automated Recommendation Tool (ART), described in a pair of papers recently published in the journal Nature Communications.
In the first paper the researchers presented the algorithm and demonstrated its capabilities in synthetic biology with simulated and historical data from previous metabolic engineering projects, such as improving the production of renewable biofuels.
In the second paper the team used ART to guide the metabolic engineering process to increase the production of tryptophan by a species of yeast called Saccharomyces cerevisiae (also known as baker’s yeast). Tryptophan is an amino acid with various uses.
In this paper the researchers selected five genes, each controlled by different gene promoters and other mechanisms within the cell. The total potential combinations of biological pathways encompassed by their selection was nearly 8,000. Using experimental data obtained on 250 of the pathways, just three percent of the total possible combinations, the team trained ART to learn how amino acid production is associated with gene expression.
The algorithm then used statistical inference to extrapolate how each of the remaining 7,000+ combinations would affect tryptophan production. Once this was achieved, ART recommended a design that increased tryptophan production by 106 percent over the state-of-the-art reference strain and by 17 percent over the best designs used for training the model.
“This is a clear demonstration that bioengineering led by machine learning is feasible, and disruptive if scalable. We did it for five genes, but we believe it could be done for the full genome,” said Garcia Martin. “This is just the beginning. With this, we have shown that there is an alternative way of doing metabolic engineering. Algorithms can automatically perform the routine parts of research while you devote your time to the more creative parts of the scientific endeavour: deciding on the important questions, designing the experiments and consolidating the obtained knowledge.”
The team said that while they were surprised by how little data ART needed to generate results, the true potential of the system would only be realised if it is trained using more data. Garcia Martin describes synthetic biology as being only in its infancy – the equivalent of where the Industrial Revolution was in the 1790s: “It is only by investing in automation and high-throughput technologies that you will be able to leverage the data needed to really revolutionise bioengineering.”
Radivojevic added: “We provided the methodology and a demonstration on a small dataset; potential applications might be revolutionary given access to large amounts of data.”
Garcia Martin concluded: “If we could automate metabolic engineering, we could strive for more audacious goals. We could engineer microbiomes for therapeutic or bioremediation purposes. We could engineer microbiomes in our gut to produce drugs to treat autism, for example, or microbiomes in the environment that convert waste to biofuels. The combination of machine learning and CRISPR-based gene editing enables much more efficient convergence to desired specifications.”
Related topics
Artificial Intelligence, Bioengineering, Genomics, Technology
Related organisations
Department of Energy's Lawrence Berkeley National Laboratory (Berkeley Lab)
Related people
Hector Garcia Martin, Tijana Radivojevic
Technology is making things easier and on advanced knowledge and thank you for sharing this thorough post on use of machine learning ins bio-engineering.