New bioinformatics tool accurately tracks synthetic DNA
Posted: 1 March 2021 | Victoria Rees (Drug Target Review) | No comments yet
A team has demonstrated that their bioinformatics approach, PlasmidHawk, can analyse DNA sequences to identify the source of engineered plasmids.
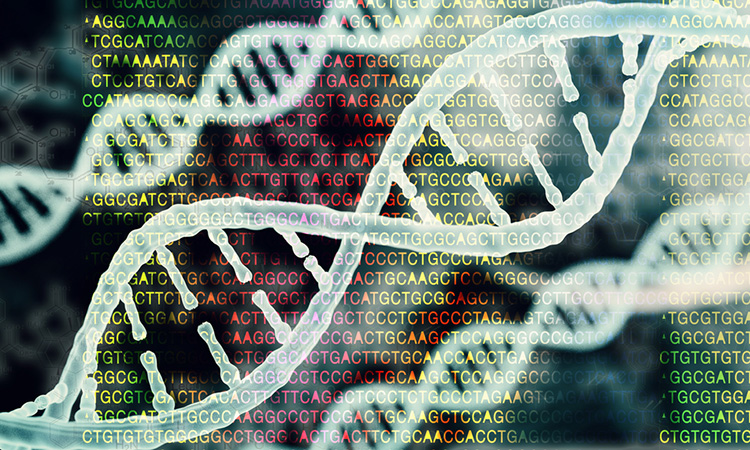
New bioinformatics research by computer scientist Todd Treangen of Rice University, US, has focused on whether sequence alignment and pan-genome-based methods can outperform recent deep learning approaches when tracking the origin of synthetic genetic code.
“This is, in a sense, against the grain given that deep learning approaches have recently outperformed traditional approaches,” Treangen said. “My goal with this study is to start a conversation about how to combine the expertise of both domains to achieve further improvements for this important computational challenge.”
Treangen and his team at Rice introduced PlasmidHawk, a bioinformatics approach that analyses DNA sequences to help identify the source of engineered plasmids of interest.
“We show that a sequence alignment-based approach can outperform a convolutional neural network (CNN) deep learning method for the specific task of lab-of-origin prediction,” he said.
According to the researchers, the programme may be useful not only for tracking potentially harmful engineered sequences but also for protecting intellectual property.
“The goal is either to help protect intellectual property rights of the contributors of the sequences or help trace the origin of a synthetic sequence,” Treangen said. PlasmidHawk directly aligns unknown strings of code from genome data sets and matches them to pan-genomic regions that are common or unique to synthetic biology research labs
“To predict the lab-of-origin, PlasmidHawk scores each lab based on matching regions between an unclassified sequence and the plasmid pan-genome and then assigns the unknown sequence to a lab with the minimum score,” said lead author Qi Wang.
The researchers reported the successful prediction of “unknown sequences’ depositing labs” 76 percent of the time. They found that 85 percent of the time the correct lab was in the top 10 candidates.
Unlike the deep learning approaches, they say PlasmidHawk requires reduced pre-processing of data and does not need retraining when adding new sequences to an existing project. It also differs by offering a detailed explanation for its lab-of-origin predictions in contrast to the previous deep learning approaches.
“The goal is to fill your computational toolbox with as many tools as possible,” said co-author Ryan Leo Elworth, a postdoctoral researcher at Rice. “Ultimately, I believe the best results will combine machine learning, more traditional computational techniques and a deep understanding of the specific biological problem you are tackling.”
The researchers reported their results in Nature Communications. The open-source software is available here.
Related topics
Bioinformatics, DNA, Informatics, Patents
Related organisations
Rice University
Related people
Qi Wang, Ryan Leo Elworth, Todd Treangen