How AI is accelerating the future of precision medicine
Posted: 12 January 2024 | Ellen Capon (Drug Target Review) | No comments yet
Dr Ketan Patel, Clarivate, shares his insights about the use of Real-World Data and genomic biomarker data and discusses how researchers can use these to better detect and diagnose diseases.
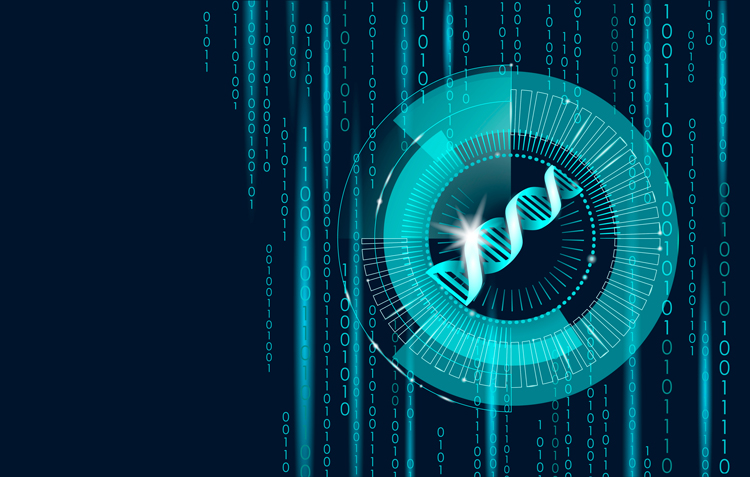
How does the integration of Real-World Data (RWD) with genomic biomarker data contribute to a more comprehensive understanding of disease progression and treatment response?
Most drugs do not work in all people. This one size fits all approach to drug prescribing still pervades in mostly all therapy areas except oncology. So, knowing an individual and something about their profile, whether that be biomarkers such as genomic data, blood-based markers or even transcriptomics of tissues or blood, provides a way to observe how that individual’s molecular pathways are configured. Based on the drugs mechanism of action, we can make informed decisions.
Whether the drug will benefit the patient or not is an important consideration, as much of the time not taking a drug is equally important as it spares a lot of pain and side effects which may not be needed. Therefore, having biomarker information is useful for separating individuals and ensuring that you treat an individual as an individual and can tailor their therapy.
Biomarker information is also useful for tracking progression of the disease and treatment response. For example, circulating tumour cells in the blood can give evidence of whether the tumour is still present and what kind of genetic makeup it has, like if the tumour is still harbouring the original mutations that was used to determine the original course of treatment, or has it changed? This can lead to a new treatment being offered to the patient.
By combining both real-world data (RWD) and biomarker information, we can be provided with a real understanding of the causes and effects of whether a drug treatment is influencing some downstream pathways as measured by biomarkers. Biomarkers could also be imaging based, and even wearable technology can track certain attributes about the patient.
In what ways can AI algorithms effectively analyse vast data sets to identify patterns that could serve as crucial diagnostic markers?
Most diseases are multigenic, so there are several genetic and external factors that can affect the disease and the subtype that an individual has. The human genome has around 20,000 genes, meaning there are many potential combinations to sift through: too many for a human. Algorithms that leverage AI can sift through the new datasets that we are generating, like using whole genome sequencing.
However, algorithms must be goal directed. For example, if you are looking for markers of type 2 diabetes patients who are still unable to maintain their blood sugar level even after insulin treatment, you could give these patients’ genetic data and RWD to the AI to find patterns in their genomics or other molecular data to find diagnostic markers.
These markers can be analysed with the current knowledge about molecular pathways. At Clarivate we have a database of molecular interactions and molecular pathways that could help to contextualise those molecular markers. These could then give you a way of producing a therapeutic hypothesis, or potential drug targets that could lead to drugs for that specific cohort of diabetes patients.
Today, diseases are only what we created as a way of naming disorders, a historic creation from doctors who studied patients that had common symptoms. Deep phenotyping and AI could assist in the production of patterns which describe a set of patients – in effect, creating a new disease definition.
Using AI, we could leverage the large amount of phenotypical data and RWD to begin to stratify diseases into different sub-groups. For example, there is not just one type of diabetes as we know it. There are probably lots of different subtypes, and each subtype may have a different root cause and therapeutic strategy, opening the door for new precision medicines.
What strategies are being developed to address inconsistencies within Real World Data sets, ensuring that AI-driven approaches in precision medicine deliver reliable and unbiased insights?
The need for clean, high quality, deduplicated data to do machine learning is crucial. RWD includes things that are captured in routine care, including diagnosis, the pills prescribed, and the length of hospital stay. Much of the time that is not the same as what is measured in a clinical trial for an outcome. Surrogate endpoints can mimic endpoints used in clinical trials. There is a lack of surrogate endpoints, for example in real world progression data in oncology. Integrating surrogate endpoints into the EMR so that data is captured routinely would improve this.
Data cleansing and quality is a real issue with RWD, but should be made easier using AI, like standardising units of measurements for lab tests and mapping lab tests to a standard code set so they can be compared across patients and hospitals. AI could have a significant role in data harmonisation.
Regarding bias, to curate RWD cohorts with clarity requires a test of the data set against a gold standard data like US Census data or the SEER cancer registry. This will ensure that we can check whether this data set is like what we see from a broad population of patients. For example, 85 percent of lung cancers are non-small cell lung cancers and 15 percent are small cell lung cancer. This ratio should be preserved in the data set. Within the non-small cell lung cancers, about 15 percent of those are EGFR positive. If you saw 50 percent EGFR positive, instead of 15 percent, this would indicate some type of bias, like too many academic medical centres who do more genetic testing were used in your group of hospitals. The gold standard of data is something that needs to be addressed at more of an industry level.
How does the integration of AI algorithms in precision medicine facilitate the identification of personalised treatment plans based on individual patient characteristics?
Organisations like academic medical centres have always collected a lot of data on patients, their genetics and their past treatments and outcomes. The Moffitt Cancer Center is a notable example of this, which asks each patient if they can collect their molecular data and their outcomes, and whether they can follow up with them afterwards for further research to benefit future patients. Therefore, AI can be leveraged to produce a specific treatment plan for patients based on specific mutations etc. to find the optimal outcome.
Currently, using a multidisciplinary team is the way this is done. Typically, this includes a pathologist, a geneticist and an oncologist or radiologist. They review the patient’s data together, ascertaining the best treatment plan. AI could become a member of that team to augment their knowledge and produce suggestions based on the patient’s data. It could suggest a new clinical trial of an experimental agent that could be exactly fitting the patient’s tumour genetic profile.
In the future, AI could also help with designing a personalised treatment regimen using cell therapies – eg, the patient’s tumour is analysed using molecular omics techniques, which is fed into an AI which produces a personalised optimal cell therapy. Sent to a machine, which uses CRISPR guided genomic editing, the cell therapy can be precisely tailored. Within a short time, the patient is infused with that cell therapy regimen which completely ameliorates the disease.
Can you discuss specific examples where AI applications have significantly advanced the field of precision medicine?
Tempus health have a product, ‘Tempus One,’ that uses AI and databases they have developed along with data held in the medical centre to help doctors with treatment planning. It allows doctors to ask questions based on patient’s data to the AI which shows which drugs are available for treatment or guides them to further tests. It does not give recommendations because this is up to the doctor to decide how to treat their patients.
Google DeepMind partnered with an eye clinic in London to take all their retinal scans data and use them to train an AI to correctly diagnose multiple eye conditions, like glaucoma. This enables doctors to triage which cases that are easy to diagnose, and which need referral to a specialist. This technology is fairly accurate but there is still a chance it could misdiagnose so, like any other diagnostic tool, it needs to be used as part of a multi-disciplinary setup.
Lastly, there are several AI companies which have drugs in the clinic now, which were completely designed using AI technology. AI can sift through the mountains of biological literature and OMICS data to produce a plausible target hypothesis for a drug for a particular disease and can generate candidate molecules that can hit that target.
AI technology can accelerate the rate at which we develop these drugs, meaning it is more cost-effective – research into smaller diseases and rare diseases will benefit from this. Also, patients will benefit as there will be more drugs developed. Last year, the number of new drug approvals by the FDA reached 56, which was a record for one year. Hopefully we can continue that rate.
About the author
Dr Ketan Patel
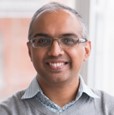
Vice President, Product Management, Clarivate
Ketan Patel is a VP of Product Management at Clarivate. He has fifteen years of experience in the pharmaceutical industry having begun his career in early discovery research for Eli Lilly and Company before moving to a more translational research role at Pfizer. Most recently, before joining Clarivate, Ketan worked for AstraZeneca in the health informatics domain. He has also worked in the technology space for the likes of Oracle and Teradata.
Related topics
Artificial Intelligence, Biomarkers, Drug Discovery, Drug Targets, Genomics, Machine learning, Oncology, Personalised Medicine, Precision Medicine
Related conditions
Cancer, Glaucoma, Type-2 diabetes
Related organisations
Clarivate
Related people
Dr Ketan Patel (Clarivate)