Using proteomics to overcome glioblastoma resistance
Posted: 17 January 2024 | Ellen Capon (Drug Target Review) | No comments yet
Using an AI algorithm to predict glioblastoma’s most active kinase, researchers hope for a next-generation precision therapy targeting resistant cancers.
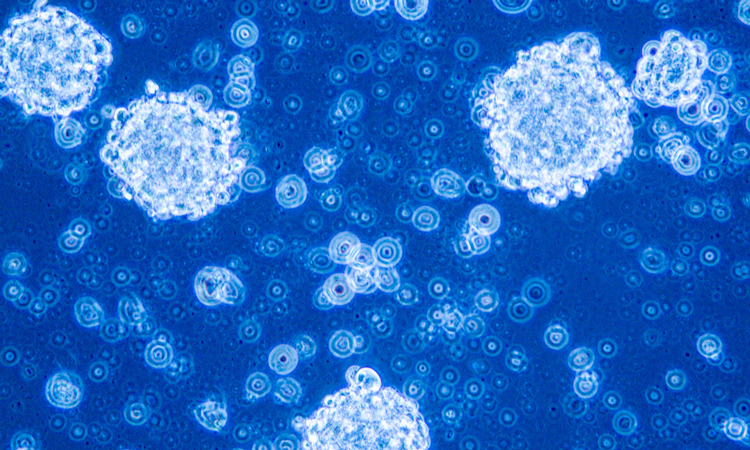
Scientists at the Sylvester Comprehensive Cancer Center at the University of Miami Miller School of Medicine, and collaborating organisations, have provided a new understanding of how glioblastoma cells mimic healthy human neurons. This insight could lead to potential therapies to prevent treatment resistance.
Glioblastoma is the most common malignant primary brain tumour, with a poor prognosis of a median survival of less than two years.1 Advances in treatment are challenged owing to several resistance mechanisms that involve limited drug entry into the central nervous system (CNS) compartment by the blood-brain barrier (BBB) and by actions of the normal brain to counteract tumour-targeting medications.2
Also, glioblastomas have a vast heterogeneity, which contributes to resistance by preventing a single drug controlling the entire tumour and facilitating escape mechanisms from targeted agents. Furthermore, the stem cell-like characteristics promote resistance to treatment through upregulation of efflux transporters, promotion of glioblastoma stem cell proliferation in neurogenic zones, and immune suppression.3
Dr Antonio Iavarone, Deputy Director at Sylvester, led the study with Dr Jong Bae Park from the National Cancer Center in Korea. Dr Iavarone explained that the team used a platform designed to study glioblastoma cells’ proteomes, including specific modifications on those proteins that indicate enzyme activity in cells. He said: “These platforms can provide a landscape of alterations in individual tumours that you cannot get from genetics alone.”
Proteomics
According to the researchers, the new study represents the first time proteomics has been used to investigate glioblastoma’s transition from treatable to treatment resistant. The dataset became the largest of its kind, which featured matched tumour samples from 123 glioblastoma patients at diagnosis and then recurrence after initial therapy.
By examining the tumours’ proteomes and protein modifications in the samples, the scientists observed significant changes not previously detected in similar cancer studies that focused on tumour genomes or transcriptomes. A specific modification, phosphorylation, showed that prior to treatment, glioblastoma cells were in a proliferative state where the cells expend energy towards replicating themselves.
Cancer cells normally grow much faster than healthy cells, so many chemotherapies target the cell functions in self-replication. However, once tumours recurred in glioblastoma patients months later, the cells looked different: like healthy neurons. Initial glioblastoma treatment is a combination of chemotherapy, radiation and surgery, meaning that some aspect of the replication-to-neuronal transition aids cancer cells’ evasion of this treatment.
Kinases
This new dataset was used to find potential therapies to kill these resistant cancers, focusing on kinases, which are responsible for phosphorylating other proteins. One of the study’s lead authors Dr Simona Migliozzi, Assistant Scientist at Slyvester, employed a machine-learning (ML) approach that she and her team had previously developed to identify the most active kinases in the neuron-like glioblastomas.
The BRAF kinase stood out. The raf murine sarcoma viral oncogene homolog B (BRAF) proto-oncogene is located in the long arm of chromosome 7 (7q34) and encodes for the B-Raf protein, a serine/threonine kinase belonging to the RAF protein family which includes A-Raf and C-Raf.4 In some cancers, such as melanoma, the gene encoding for BRAF is frequently mutated, although in glioblastoma BRAF protein levels increase without corresponding gene changes. This would not have been discovered without examining the cancer proteome.
An existing BRAF inhibitor, vemurafenib, was assessed on treatment-resistant glioblastoma cells in a petri dish and a patient-derived xenograft tumour in mice. Vemurafenib, used in combination with temozolomide, destroyed the formerly resistant tumours in both models. The BRAF inhibitor extended the mouse model’s survival over chemotherapy alone.
AI algorithm
The practice of using the artificial intelligence (AI) algorithm to predict glioblastoma’s most active kinase could be applied to other cancer types, Dr Iavarone believes. Alongside colleagues, he is developing a clinical test that would use AI to find therapeutic weaknesses in multiple cancers by finding each tumour’s most active kinase. This would enable the tumour to be treated with an existing kinase inhibitor.
Dr Iavarone commented: “Proteomics gives us a much more direct prediction of protein activity…We hope this analysis can be seamlessly translated into the clinic as a next-generation precision therapy for this very challenging disease and other resistant cancers as well.”
This study was published in Cancer Cell.
References
1 Ashely DM, Friedman HS, Khasraw M, et al. Management of glioblastoma: State of the art and future directions. CA: A Cancer Journal for Clinicians. 2020 June 1 [2024 January 12]; 70(4):299-312. Available from: https://acsjournals.onlinelibrary.wiley.com/doi/full/10.3322/caac.21613
2 Magge R, Noch EK, Ramakrishna R. Challenges in the Treatment of Glioblastoma: Multisystem Mechanisms of Therapeutic Resistance. World Neurosurgery 2018 August [2024 January 12]; 116:505-17. Available from: https://www.sciencedirect.com/science/article/abs/pii/S1878875018307381
3 Magge R, Noch EK, Ramakrishna R. Challenges in the Treatment of Glioblastoma: Multisystem Mechanisms of Therapeutic Resistance. World Neurosurgery 2018 August [2024 January 12]; 116:505-17.
Available from: https://www.sciencedirect.com/science/article/abs/pii/S1878875018307381
4Aldegheri G, Bouche V, Donofrio CA, et al. BRAF Signaling Inhibition in Glioblastoma: Which Clinical Perspectives? Frontiers in Oncology 2021 November 3 [2024 January 12]; 11. Available from: https://www.frontiersin.org/journals/oncology/articles/10.3389/fonc.2021.772052/full
Related topics
Artificial Intelligence, Cancer research, Central Nervous System (CNS), Precision Medicine
Related conditions
Cancer Research, Glioblastoma
Related organisations
National Cancer Center Korea, Sylvester Comprehensive Cancer Center