Adapting drug discovery to Artificial Intelligence
Posted: 25 July 2018 | Ami S Lakdawala (GSK's In-silico drug discovery unit), George Okafo (GSK's In-silico drug discovery unit), John Baldoni (GSK's In-silico drug discovery unit), Michael Palovich (GSK's In-silico drug discovery unit), Tobias Sikosek (GSK's In-silico drug discovery unit), Vishal Sahni (GSK's In-silico drug discovery unit) | No comments yet
Drug discovery has always been challenging; today, more so than ever. While there has been success in addressing many diseases, others remain intractable…
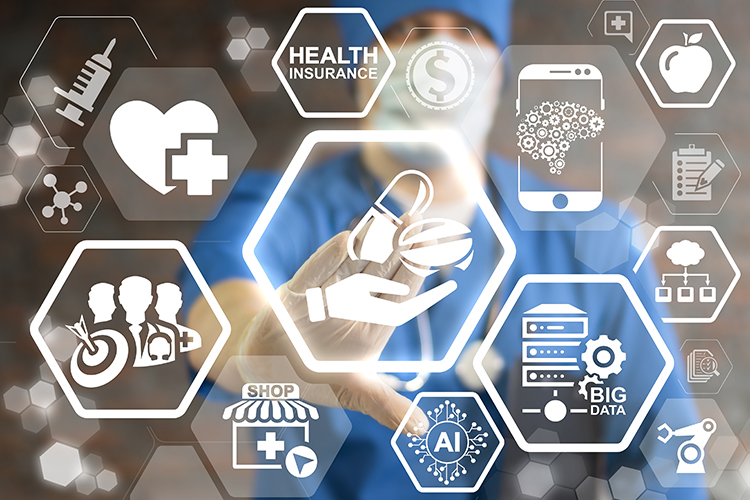
There is a need and opportunity to explore new drug discovery approaches that harness immense datasets (public and private), which have been built upon the successes and failures of the past to guide in-silico approaches to new therapies. Advances in genetics and molecular biology have revealed potential new targets for developing medicines. Deciding which target to pursue is challenging and an area in which there is opportunity to increase productivity. We want to move to a highly efficient model that utilises emerging technologies and harnesses the potential of ‘big data’.
GSK formed a new drug discovery unit in 2017 to test whether an integrated artificial intelligence and machine learning approach can expedite drug discovery from biological hypothesis to proof-of-human pharmacology. This unit forms part of GSK’s overall approach in digital, data, and analytics (DDA). The unit applies artificial intelligence (AI) – specifically, machine learning (ML) and, especially, deep learning (DL) – to five key workflows in drug discovery (Figure 1).
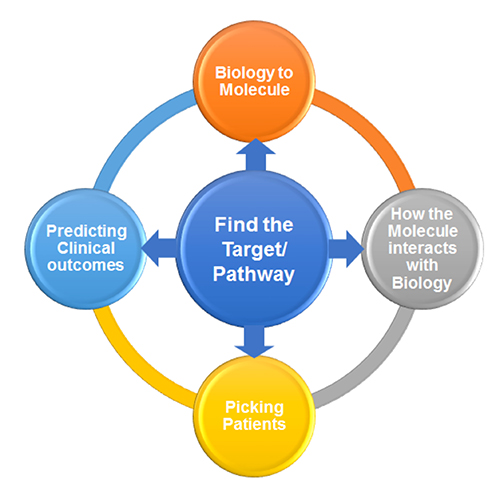
Figure 1 Workflow integrating AI / ML in drug discovery.
The aim is to:
- Understand the patient and disease to find the target / pathway
- Find the molecule
- Understand how it interacts with biology
- Identify a suitable patient population on which to test the hypothesis and predict clinical outcomes.
We believe that integrating the tools in three axes – deep biology, machines designed to quantify and integrate biology and chemistry, and data-driven algorithms – could help transform the drug discovery process for selected diseases.
Related topics
Analytical Techniques, Artificial Intelligence, Disease Research, Drug Discovery, Therapeutics