Advantages of using cfDNA sequencing for early detection of GDM
Posted: 2 February 2024 | Drug Target Review | No comments yet
A deep learning model developed using circulating cell-free DNA outperformed traditional screening methods for gestational diabetes mellitus.
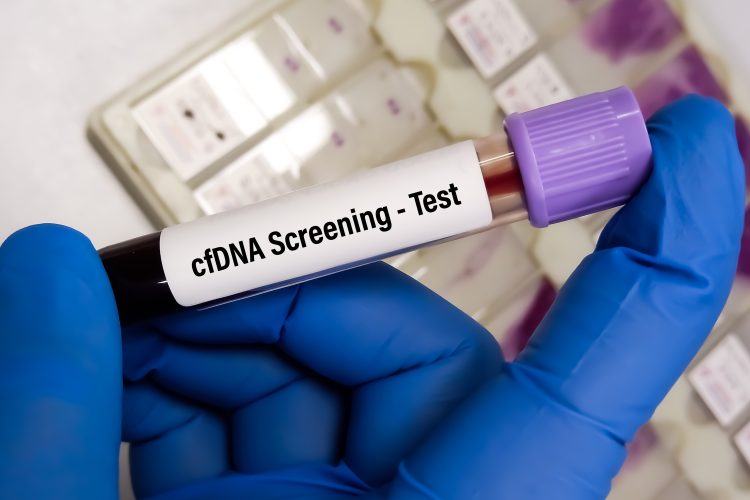
Researchers from BGI Genomics, in collaboration with the Beijing Obstetrics and Gynecology Hospital, have developed a non-invasive method for early detection of gestational diabetes mellitus (GDM), the pregnancy complication that presents great health risks to mothers and their babies, using cell-free DNA (cfDNA) from maternal plasma and deep learning models.
GDM, which typically affects younger women, is characterised by any degree of glucose intolerance that arises during pregnancy and it is crucial to detect and treat early. Current screening methods, including glucose tolerance tests, are done after 24 weeks of pregnancy, but these have limitations regarding patient compliance and accuracy.
To predict GDM status, the deep learning model was developed using circulating cell-free DNA samples from 5,085 pregnant women. Of these, 1,942 were GDM patients, and the others were healthy controls. A Convolutional Neural Networks (CNN)-based deep neural network was built. This had a self-attention layer to analyse copy numbers of cfDNA sequencing data linked to GDM, focusing on discovering essential genetic regions for accurate classification.
The results showed that this model has many advantages. The risk of GDM can be predicted non-invasively in the first trimester, which is much earlier than traditional glucose tolerance tests performed near the end of the second trimester. The deep learning model outperformed traditional screening methods as it had an accuracy of 93.5 percent in predicting GDM status. Also, this model has the potential to improve patient compliance.
The analysis of cfDNA copy number variations (CNVs) associated with diabetes genes also enabled the identification of crucial genetic regions for accurate classification of GDM and CNV fragments covering 2190 genes. These included known genes related to GDM such as alpha- and beta- defensin genes (DEFA1, DEFA3, DEFB1). It enabled the identification of enriched biological processes and pathways linked to diabetes, like glutamate signalling, forebrain development and GTPase regulator activity.
Overall, these results show a major step forward in understanding molecular mechanisms underlying GDM and provide insights for future therapeutic strategies.
This study was published in Briefings in Bioinformatics.
Related topics
Disease Research, DNA, Genetic Analysis, Screening
Related conditions
gestational diabetes mellitus (GDM)
Related organisations
Beijing Obstetrics and Gynecology Hospital, BGI Genomics