New AI process for accurate enrolment of patients to clinical trial
Posted: 20 June 2024 | Drug Target Review | No comments yet
The AI model screened the medical records of heart failure patients more accurately, quickly and cheaply, compared to study staff.
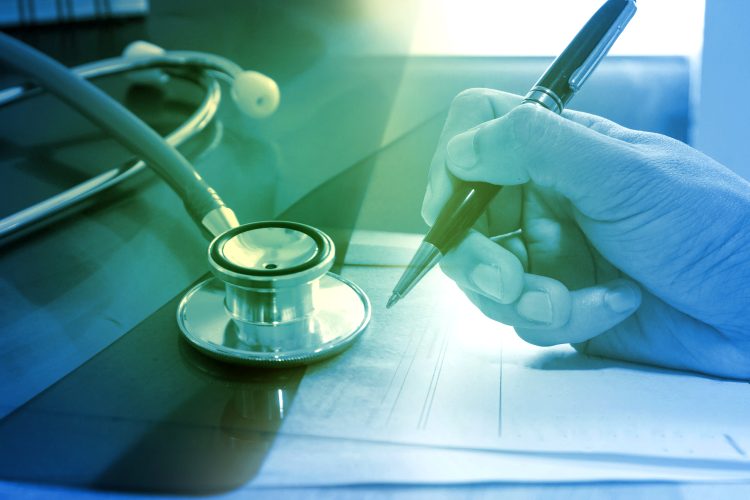
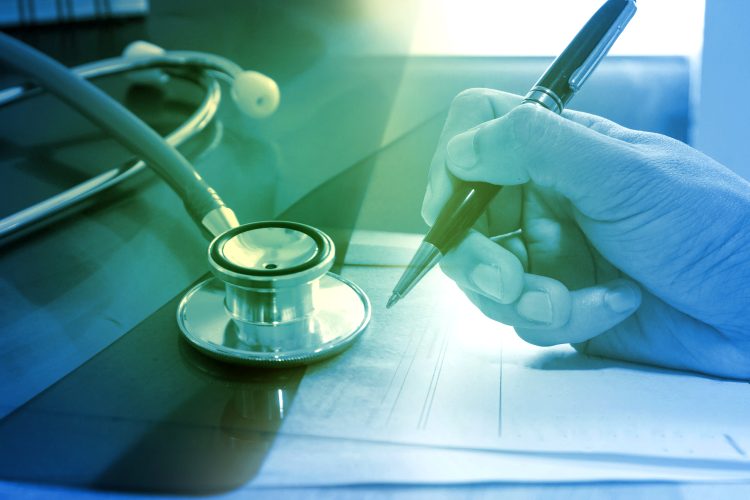
Researchers at Mass General Brigham have studied the accuracy and cost of a Generative Artificial Intelligence (Gen AI) process, called RAG-Enabled Clinical Trial Infrastructure for Inclusion Exclusion Review (RECTIFIER). Based on their medical records, this accurately, cheaply and quickly identified individuals who are eligible for enrolment in a heart failure clinical trial.
Dr Samuel (Sandy) Aronson, co-senior author, stated: “We saw that large language models hold the potential to fundamentally improve clinical trial screening… Now the difficult work begins to determine how to integrate this capability into real world trial workflows in a manner that simultaneously delivers improved effectiveness, safety, and equity.” Dr Aronson is executive director of IT and AI Solutions for Mass General Brigham Personalized Medicine and senior director of IT and AI Solutions for the Accelerator for Clinical Transformation.
Co-lead author Dr Ozan Unlu, a fellow in Clinical Informatics at Mass General Brigham and a fellow in Cardiovascular Medicine at Brigham and Women’s Hospital, highlighted: “Screening of participants is one of the most time-consuming, labour-intensive, and error-prone tasks in a clinical trial.”
The scientists assessed RECTIFIER’s ability to find patients suitable for The Co-Operative Program for Implementation of Optimal Therapy in Heart Failure (COPILOT-HF) trial. This trial recruits those with symptomatic heart failure, detecting potential individuals using electronic health record (EHR) data. To evaluate clinical trial eligibility, the team designed 13 prompts, testing and changing them using medical charts of a small group of patients. The prompts were then applied to a dataset of 1,894 patients with an average of 120 notes per patient.
Comparing the screening performance of the AI process to the performance of study staff, the AI was 97.9 percent to 100 percent accurate, based on alignment with an expert clinician’s “gold standard” assessment of whether the patients met trial criteria. Study staff examining the same medical records were less precise, with accuracy rates between 91.7 percent and 100 percent.
Furthermore, the team estimated the AI model costs around $0.11 to screen each patient, which is cheaper than traditional screening approaches. Co-senior author Dr Alexander Blood, cardiologist at Brigham and Women’s Hospital and associate director of the Accelerator for Clinical Transformation, commented: “If we can accelerate the clinical trial process and make trials cheaper and more equitable without sacrificing safety, we can get drugs to patients faster and ensure they are helping a broad population.”
However, because AI may introduce bias and miss nuances, the model should be continuously examined when it is included to routine workflows. An alteration in how data is captured could substantially impact its performance. The scientists recommended that a final check of the trial’s participants should be conducted by a clinician.
Dr Blood concluded: “Our goal is to prove this works in other disease areas and use cases while we expand beyond the walls of Mass General Brigham.”
This study was published in NEJM AI.
Related topics
Artificial Intelligence, Clinical Trials, Targets, Therapeutics, Translational Science
Related conditions
Heart failure
Related organisations
Brigham and Women's Hospital, Mass General Brigham