Navigating the challenges and opportunities of AI in drug development and personalised medicine
Posted: 18 July 2023 | Izzy Wood (Drug Target Review) | No comments yet
This article is the second part of Drug Target Review’s Izzy Wood’s discussion with Olivia Cavlan, Chief Corporate Development and Strategy Officer at Alchemab Therapeutics Ltd, exploring the role of AI in target discovery, its applications in personalised medicine, and the evolving landscape of pharmaceutical development.
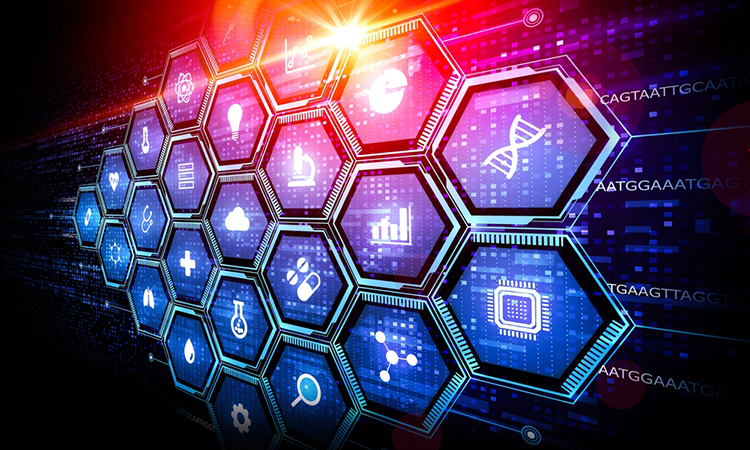
Artificial Intelligence (AI) is poised to transform the field of target discovery in drug development, offering immense potential to enhance efficacy, personalised medicine, and accelerate the development of innovative compounds. However, as AI becomes increasingly integrated into the drug discovery process, challenges related to data quality, collaboration, and regulatory adaptation need to be addressed to ensure its successful implementation. This article is the second part of my interview with Olivia Cavlan, Chief Corporate Development and Strategy Officer at Alchemab Therapeutics Ltd, this time exploring the AI applications in personalised medicine, and the evolving landscape of drug development.
According to Cavlan, a simplistic way of looking at AI in target discovery is to think about its application along the discovery workflow in three different steps. AI is being used to find the targets themselves, design the drugs to manipulate that biology, and thirdly, AI is being used to support validation of those targets and drugs as part of pre-clinical development. Cavlan notes that underpinning this use of AI in early discovery is the need to better understand biology.
The challenge with AI advancements is whether health systems can keep up with the swift landscape of personalised medicine.
If we look at the pharmaceutical industry’s discovery and development success rate over the last 20 to 30 years, it has not improved, staying stubbornly at around 10 percent. We continue to see costly and time consuming phase 2 and later stage failures despite advances in our understanding of genetics, so we must ask why.
A significant chunk of molecules fail in clinic because of efficacy reasons. They do not have the efficacy that you need them to, and the underlying factor behind efficacy is biology. We must then ask: “Are you impacting the biological system that you want to in the right kind of way?”
“A way to really improve efficacy is to select the right targets.” Cavlan, to some extent, has a biased view of this, suggesting the key to selecting these targets is to understand human samples. “I think it is astonishing that The Human Genome Project was completed exactly 20 years ago and DNA was discovered by Franklin and published by Watson and Crick exactly 70 years ago, yet that 10 percent success rate has still not improved. This means that we are not doing something right. Where AI can help us is to interrogate that biological question and make sure that we are identifying and drugging disease modifying targets in the right way.”
How to utilise AI applications for target discovery
As Cavlan and I previously discussed, there is a broad range of AI applications within target discovery. Taking a general approach, we can consider AI applications to mine for example GWAS data and combine biological data sets. Whilst combining certain data sets and mining them can be helpful, Cavlan suggests that to really unveil new horizons for treating disease, using AI in a focused way and fully integrating it with wet-lab based capabilities to create iterative rapid feedback loops is important. This is where biotechs in particular can excel, because of their laser-like focus on a particular hypothesis and the integration of capabilities along the discovery workflow.
She uses Alchemab’s platform as one example of this approach: “Alchemab’s platform focuses on unbiased target discovery, using a database of 350 million unique B cells derived from patient samples. From the database the natural evolution and selection of antibodies in silico can be understood, such that the team can identify naturally occurring protective auto-antibodies such as PD-1 and PDL-1 inhibitors before anything has even been expressed. Once expressed, the in silico predictions can be tested and validated and in addition, new targets can be found. The end-to-end workflow is only possible down to the latest advances in sequencing and AI, combined with classical approaches to drug discovery.”
Limitations and challenges
AI in drug discovery does not come without its limitations, the first of which, addressed by Cavlan, is quality data.
“There is a plethora of data available to researchers, but it is not all standardised and lacks the same metadata. It can have gaps in it, meaning sometimes you are unaware of the validity of that data, and you don’t know when you are wrong. This creates a huge challenge: it’s vital to ensure there is enough quality data for companies to be able to mine in order to create valid AI approaches and models that deliver the correct answers.”
A solution, Cavlan suggests, is that companies can generate their own data, which can mean significant expense and using high throughput sequencing technologies from companies such as Illumina. It also means working with collaborators, such as charities, biobanks and academia, to receive well-curated sample sets. She continues that “we are going to have to see more of this collaboration across the industry to get certain samples, and then establish creative ways of generating the data, either on a company level or pre-competitive collaborations that create syndicates for this data.”
AI and personalised medicine
What is AI’s potential for accelerating new medicines and revolutionising drug development?
Cavlan expressed her optimism for AI applications in the development of new medicines: “It will still take a few more years of us mining data, creating data sets, improving models and validating them through bench work and ultimately clinical trials, to be able to see those gains.
But…ultimately, if you can replace in vivo models with, for example, a spatial transcriptomics approach based on organised structures, it would significantly accelerate our ability to do drug discovery. Alongside that, there is a need for some regulatory changes, or creative approaches to reviewing data packages, but regulators are well aware of that, and seem to be quite adaptive to change.”
In terms of personalised medicine, the variability of outcomes across patients is enormous, meaning that the size of the challenge is a multitude of that. Cavlan believes that it is an area that could really benefit from AI because AI is uniquely geared towards tacking vast data questions. However, Cavlan cautions, this will probably take at least 10 years but with incremental gains along the way.
Cavlan is optimistic about the use of AI in the future development of new medicines. Sharing her thoughts, AI applications will accelerate the speed at which we develop compound” and will change the types of compounds that we have.
Yet, with these AI advancements, the challenge creeps up of whether health systems can keep up with the swift landscape of personalised medicine.
Will systems like the NHS be able to deliver it?
With support from pharmaceutical companies, ultimately, the answer to this question should be yes.
Pharmaceutical companies are an absolutely pivotal part of the life sciences industry and ecosystem. Cavlan explains that “they are brilliant at developing and bringing new drugs to market.”
In the future, these new drugs will most likely be developed with the help of AI through the clinical development process as well. But ultimately the question is, will these new drugs move the needle on that stubborn 10 percent success rate?
Author bio:
Olivia Cavlan
Olivia is Alchemab’s Chief Corporate Development and Strategy Officer where she oversees global business development and partnerships and leads corporate strategy.
Prior to Alchemab Olivia was an Investment Principal at SV Health Investors and a Partner with McKinsey & Company where she was part of the leadership team in the Healthcare Private Equity and Pharmaceutical practices. While at McKinsey, Olivia co-led the global Real World Evidence practice and QuantumBlack biopharmaceutical offering where she led work on target discovery and clinical development. She has also led several global biopharma R&D transformation efforts and developed clinical and commercial strategies for a number of preclinical assets.
Olivia is a Non-Executive Director for the UK BioIndustry Association and a member of the Investment Committee for Cancer Research UK’s (CRUK) Seed fund. She also co-founded and sits on the Board of Directors of Sevenless Therapeutics, a small molecule pain company.
Olivia holds an MBChB in Medicine and a BSc in Pharmacology from Bristol University where she graduated with the top First Class Honors.
Related topics
Artificial Intelligence, DNA, Drug Development, In Vivo, Informatics, Personalised Medicine
Related organisations
Alchemab Therapeutics Ltd
Related people
Olivia Cavlan (Alchemab)