Exploring chemical space for epigenetic drug discovery using AI
Posted: 17 December 2019 | Alp Tegin Sahin (Fraunhofer-IME), Sheraz Gul (Fraunhofer Institute) | No comments yet
It is said that, on average, it takes a new drug 12 years to go from research lab to patient, with many thousands of candidates discarded along the way. Can artificial intelligence (AI) help to speed things up? Sheraz Gul and Alp Sahin provide an overview of an AI approach to accelerate epigenetic drug discovery.
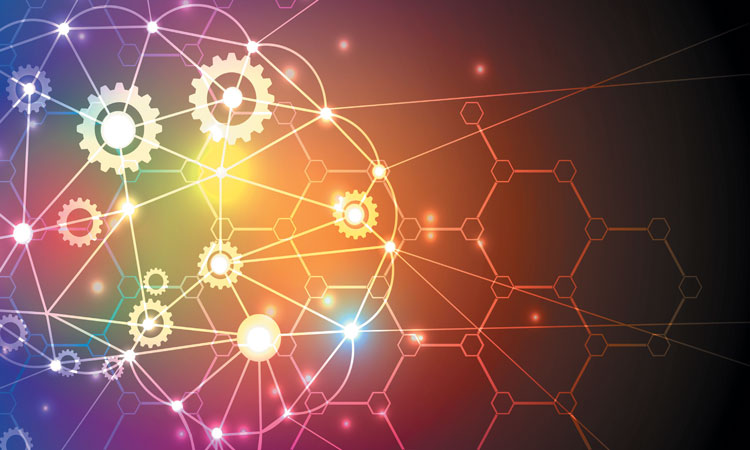
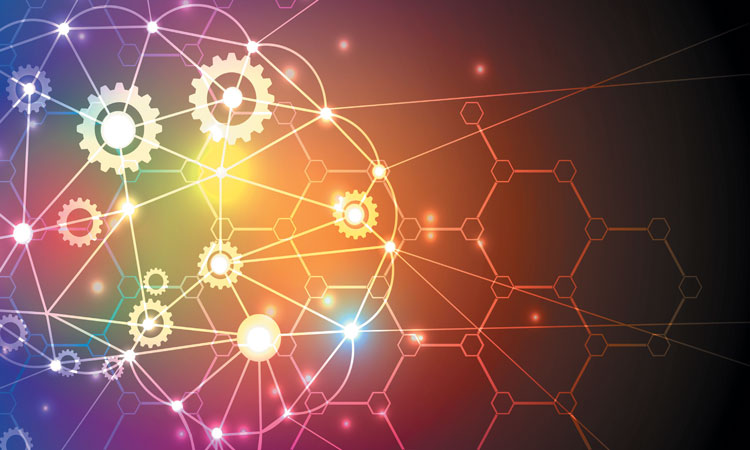
Artificial intelligence (AI) is making inroads in drug discovery. One aim for its application has been to shrink the pre-clinical phase in drug discovery from a typical five years to less than one year. This would involve designing compounds with optimised physico-chemical, off-target liability, ADMET, pharmacokinetic and pharmacodynamic properties, together with novel intellectual property rights. The designed compound should be suitable for in vivo studies and would necessitate the exploitation of all available knowledge of the target. This article relates to the application of this approach to accelerate epigenetic drug discovery.
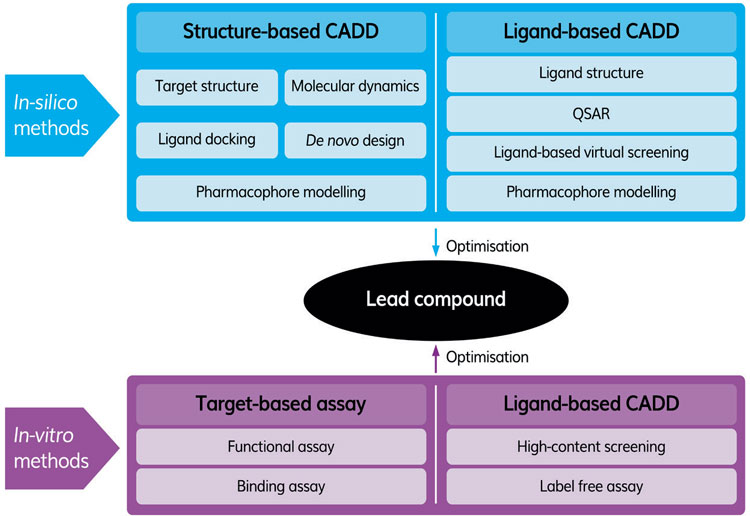
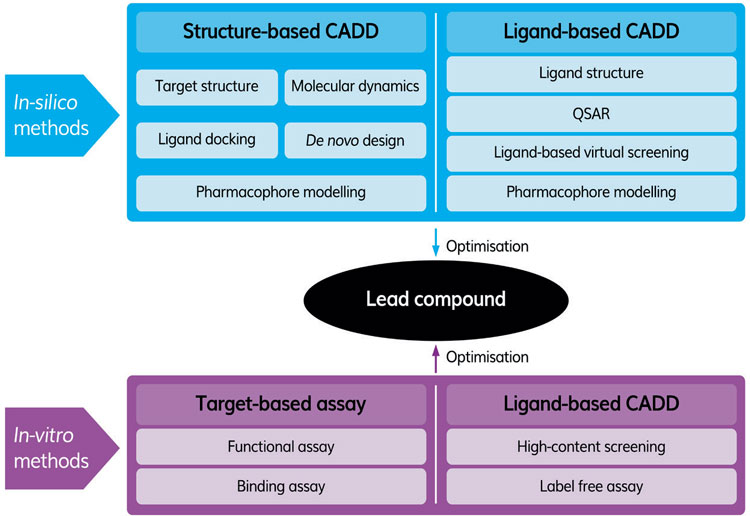
Table 1: Typical in silico and in vitro workflow to identify a lead compound.
Epigenetic drug targets are particularly suited for AI studies as various contributions have shown them to be involved in diseases such as cancer and complex biological processes such as ageing, where a multi-targeting approach may be required. Within the epigenetic drug target class, there are three main categories: writers, readers and erasers. The writers ‘mark’ histones and DNA by adding chemical groups including acetyl, phosphoryl and methyl. The readers recognise and act upon these modifications and the erasers remove them.1 In some cases, drugs that modulate the activities of specific epigenetic targets, eg, Vorinostat and Romidepsin, which inhibit the histone deacetylase class of enzymes,2 have been approved for clinical use. Other members of this class of protein are being explored as potential therapeutic targets to treat metabolic and cardiovascular disease and cancer.3 In order to improve the chances of AI delivering compounds that are likely to be successful in vivo, maximum use of existing in vitro (assays, screening campaigns, design and evaluation of compounds), in silico (structural studies to identify compounds that bind the target protein) and in vivo (animal and human) studies should be made. A typical workflow for identifying a lead compound using in silico and in vitro methods is shown in Figure 1.
The use of AI in pre-clinical drug discovery offers the potential to reduce the cycle time for lead identification. The activities involved in the AI-driven workflow are as follows:
1. Analysis of databases
Interrogate all publicly available compound databases (eg, ChEMBL, PubChem, ZINC and DrugBank) and prepare an independent database of all available drug-like compounds. This will form the starting point for applying AI to design new compounds against the relevant drug targets.
2. Prioritisation of drug targets
The drug targets should be prioritised based upon the literature and databases with a focus on a) existing approved drugs (DrugBank), b) agents in clinical trials, c) structural information and d) druggability.
3. Applying AI methods
This activity should make use of those AI techniques necessary to allow the design of novel compounds that are potential modulators of the epigenetic drug targets. The applications of AI at exascale – ie, the rate achieved by supercomputers – will allow a) preparation of a virtual library of chemically accessible compounds to increase the probability of technical success of the project, b) parallel docking to defined targets of interest with structural information, together with off targets and c) the AI-driven analysis of the results and design of novel compounds.
4. Applying in vitro methods
Having used the AI methods to design novel compounds, screening can be undertaken to confirm their activities following their synthesis. The focus at this stage should be on the assay development and screening; particularly, development of primary bioassays, compound profiling, automated data analysis and visualisation. In the case of epigenetic drug targets, many assays have been reported, which can facilitate the completion of this in vitro work (see Table 1).
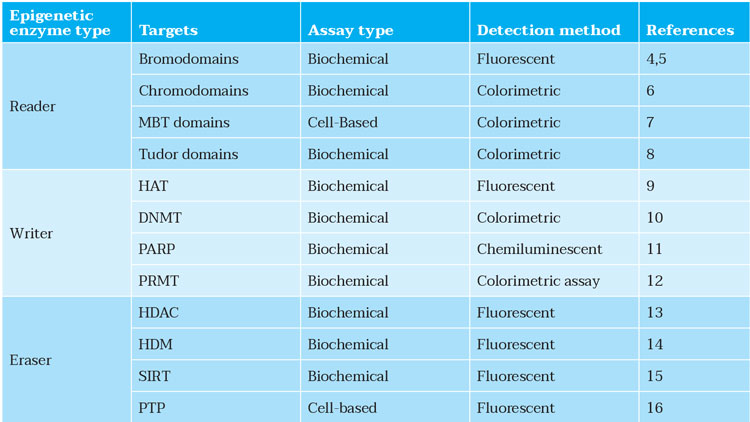
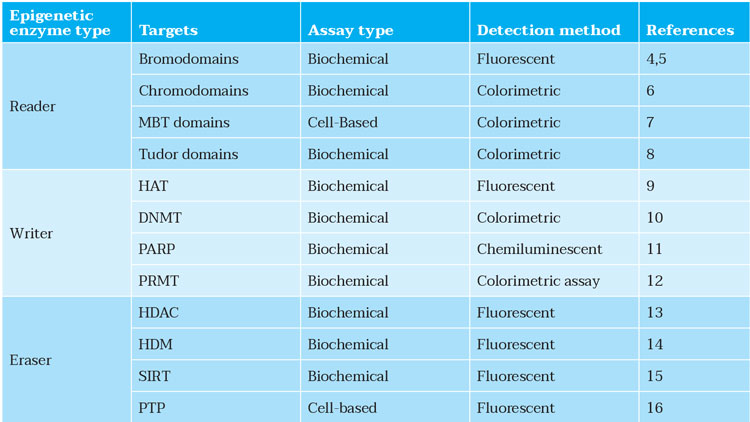
Table 1: Assay types for epigenetic drug targets. MBT: malignant brain tumour; HAT: histone acetyltransferases; DNMT: DNA methyltransferases; PARP: poly (ADP-ribose) polymerase; PRMT: protein arginine methyltransferase; HDAC: histone deacetylase; HDM: histone demethylase; SIRT: sirtuin; PTP: protein tyrosine phosphatase.
5. Further development of hit compounds
The most promising compounds identified above should possess acceptable physico-chemical, off-target liability, ADME-toxicity, pharmacokinetic and pharmacodynamic properties together with novel intellectual property rights (Table 2).
The use of AI to quickly design compounds that are suitable for in vivo validation is now becoming a reality.20-24 Although the outputs of AI require confirmation using in vitro methods, it is anticipated that in the near future compounds will be designed almost entirely using AI methods; significantly shortening the length of time a project spends in the pre-clinical phase of drug discovery.
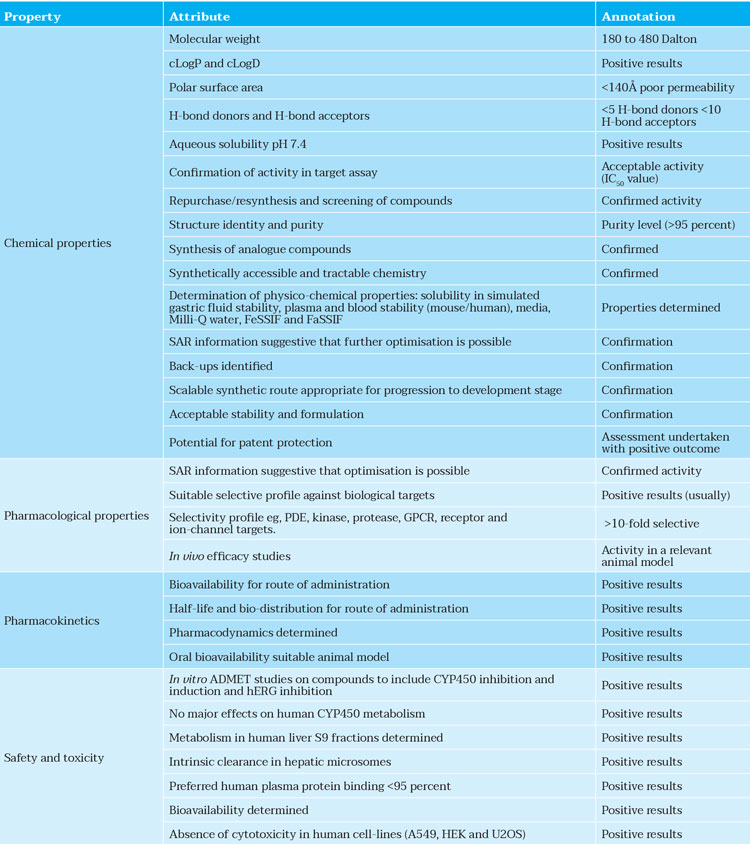
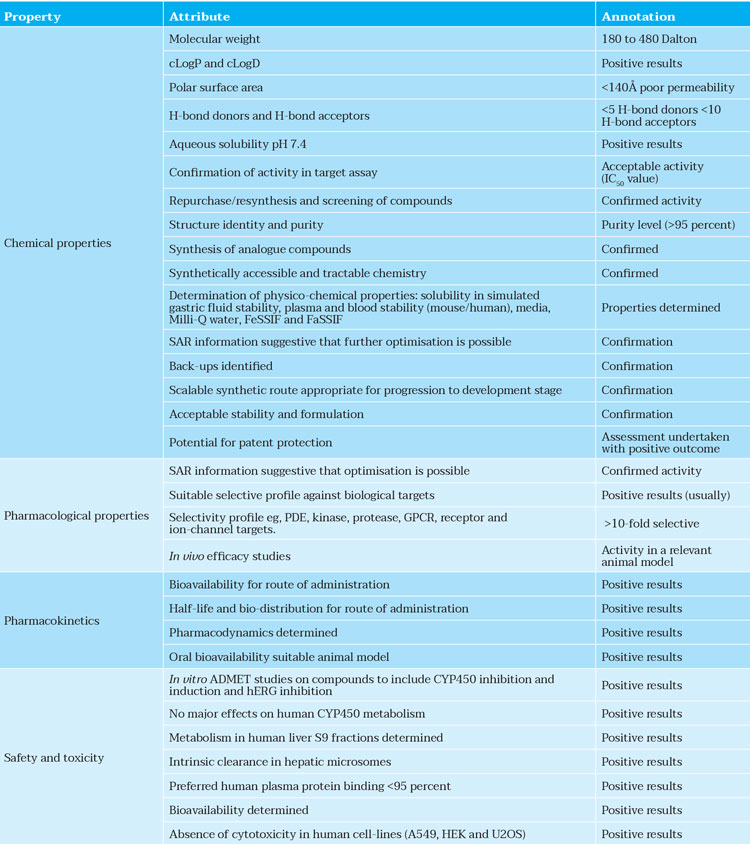
Table 2: Typical properties of a lead compound designed by AI.
About the authors
References
- Prachayasittikul V, et al. Exploring the epigenetic drug discovery landscape. Exp Opin Drug Discov. 2017, 12, 345–362.
- Bates SE, Robey RW, Piekarz RL. CCR 20th Anniversary Commentary: Expanding the Epigenetic Therapeutic Portfolio. Clin Can Res. 2015, 21, 2195–2197.
- Gul S. Epigenetic assays for chemical biology and drug discovery. Clin Epigen. 2017, 9, 41.
- Gillette TG, Hill JA. Readers, Writers, and Erasers. Circ Research. 2015, 116, 1245–1253.
- Wagner JM, et al. Histone deacetylase (HDAC) inhibitors in recent clinical trials for cancer therapy. Clin Epigen. 2010, 1, 117–136.
- Murga C, et al. G Protein-Coupled Receptor Kinase 2 (GRK2) as a Potential Therapeutic Target in Cardiovascular and Metabolic Diseases. Front Pharm. 2019, 10.
- Zhan Y, et al. Development of novel cellular histone-binding and chromatin displacement assays for bromodomain drug discovery. Epigen Chrom. 2015, 8, 37.
- Xue X, et al. Discovery of Benzo[cd]indol-2(1H)-ones as Potent and Specific BET Bromodomain Inhibitors: Structure-Based Virtual Screening, Optimization, and Biological Evaluation. J Med Chem. 2016, 59, 1565–1579.
- Wong WR, et al. Autism-associated missense genetic variants impact locomotion and neuro development in Caenorhabditis elegans. Human Mol Gen. 2019, 28, 2271–2281.
- Mielke JG, et al. Biochemical and functional characterization of diet- induced brain insulin resistance. J Neurochem. 2015, 93, 1568–1578.
- Kim M, et al. Tudor Domain Containing Protein TDRD12 Expresses at the Acrosome of Spermatids in Mouse Testis. Asian-Australasian J Ani Sci. 2015, 29, 944–951.
- Jha PK, et al. HAT2 mediates histone H4K4 acetylation and affects micrococcal nuclease sensitivity of chromatin in Leishmania donovani. PLoS One. 2017, 12:e0177372.
- Mao SQ, et al. DNA G-quadruplex structures mold the DNA methylome. Nat Struct Mol Biol. 2018, 25, 951–957.
- Wang JC, et al. Loss of Sfrp2 contributes to the neurological disorders related with morphine withdrawal via Wnt/ß-catenin signaling. Behav Brain Res. 2019, 359, 609–618.
- ZhaoY,etal.PRMT1regulatesthetumour-initiatingpropertiesof esophageal squamous cell carcinoma through histone H4 arginine methylation coupled with transcriptional activation. Cell Death Dis. 2019, 10, 359.
- Chriett S, et al. Prominent action of butyrate over ß-hydroxybutyrate as histone deacetylase inhibitor, transcriptional modulator and anti- inflammatory molecule. Sci Rep. 2019, 9, 742.
- Galoian K, et al. Effect of cytostatic proline rich polypeptide-1 on tumor suppressors of inflammation pathway signaling in chondrosarcoma. Mol Clin Oncol. 2016, 5, 618–624.
- Chen Y, et al. Hydroquinone-induced malignant transformation of TK6 cells by facilitating SIRT1-mediated p53 degradation and up-regulating KRAS. Toxi Lett. 2016, 259, 133–142.
- LorenzU.ProteinTyrosinePhosphataseAssays.CurrentProtocolsin Immunology. John Wiley & Sons Inc. 2011 93, 1–11.
- StrangBL,etal.Identificationofleadanti-humancytomegalovirus compounds targeting MAP4K4 via machine learning analysis of kinase inhibitor screening data. PLoS One. 2018, 13, e0201321.
- BajorathJ,etal.TheFutureIsNow:ArtificialIntelligenceinDrug Discovery. J Med Chem. 2019, 62, 5249.
- EkinsS,etal.Exploitingmachinelearningforend-to-enddrugdiscovery and development. Nat Mater. 2019, 18, 435–441.
- Bhhatarai B, et al. Opportunities and challenges using artificial intelligence in ADME/Tox. Nat Mater. 2019, 18, 418–422.
- Engkvist O, et al. Computational prediction of chemical reactions: current status and outlook. Drug Discov Today. 2018, 6, 1203–1218.
Related topics
Artificial Intelligence, Assays, Drug Discovery, Drug Targets, In Vitro, Screening