Using machine learning to predict symptoms
Posted: 2 January 2019 | Iqra Farooq (Drug Target Review) | No comments yet
Machine learning could, in future, be the way that clinicians decide on particular courses of treatments for patients affected by cancer…
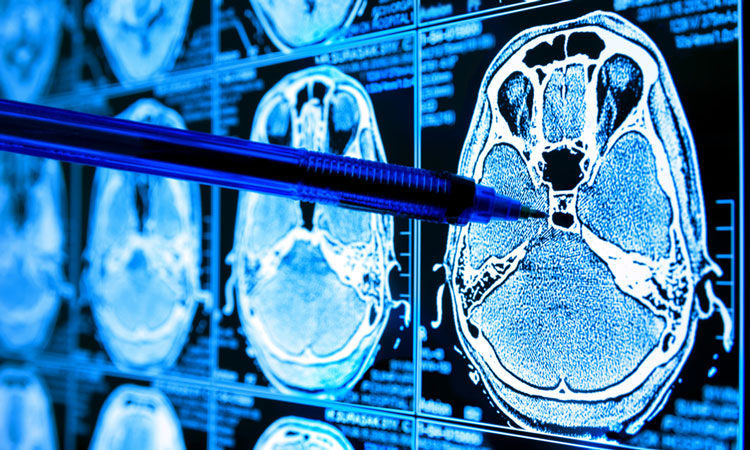
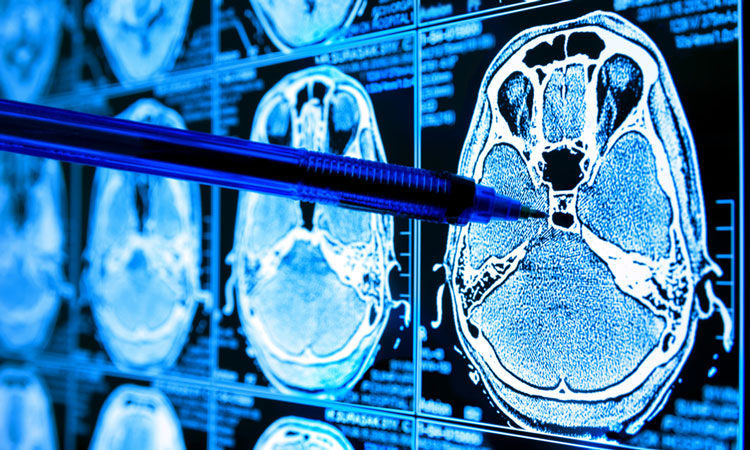
Researchers at the University of Surrey have developed Artificial Intelligence that is able to predict symptoms and how severe they could be through the course of a patient’s treatment.
Two machine learning models were developed and are able to accurately predict the severity of common symptoms that affect cancer patients; namely depression, sleep disturbance and anxiety. Each of these symptoms can lead to a huge reduction in the patients’ quality of life.
The effective management of symptoms is critical in cancer treatment, and steps to assist oncology clinicians in planning their patients’ course of treatment could progress into more effective treatments, and ones that are more aggressive against the cancer.
Whilst patients were undergoing computed tomography x-ray treatment, researchers analysed symptoms they experienced. They used different time periods during the dataset, and tested whether the machine learning algorithms were able to accurately predict when and if symptoms surfaced.
The study, conducted through a collaboration between the University of Surrey and the University of California in San Francisco (UCSF), found that the actual symptoms undergone by the patients were very close to those predicted by the machine learning methods.
“These exciting results show that there is an opportunity for machine learning techniques to make a real difference in the lives of people living with cancer. They can help clinicians identify high-risk patients, help and support their symptom experience and pre-emptively plan a way to manage those symptoms and improve quality of life,” said Dr Payam Barnaghi, Professor of Machine Intelligence.
“I am very excited to see how machine learning and AI can be used to create solutions that have a positive impact on the quality of life and well-being of patients,” said Nikos Papachristou, who worked on designing the machine learning algorithms for this study.
The study was published in the journal PLO One.
Related topics
Disease Research, Oncology, Research & Development, Therapeutics
Related conditions
Cancer
Related organisations
University of California in San Francisco, University of Surrey
Related people
Nikos Papachristou, Professor Payam Barnaghi