Novel AI tool takes a DeepCE dive into potential drugs for COVID-19
Posted: 2 February 2021 | Hannah Balfour (Drug Target Review) | No comments yet
A new phenotype-based compound screening technology, called DeepCE, identified 10 compounds that could be repurposed for COVID-19.
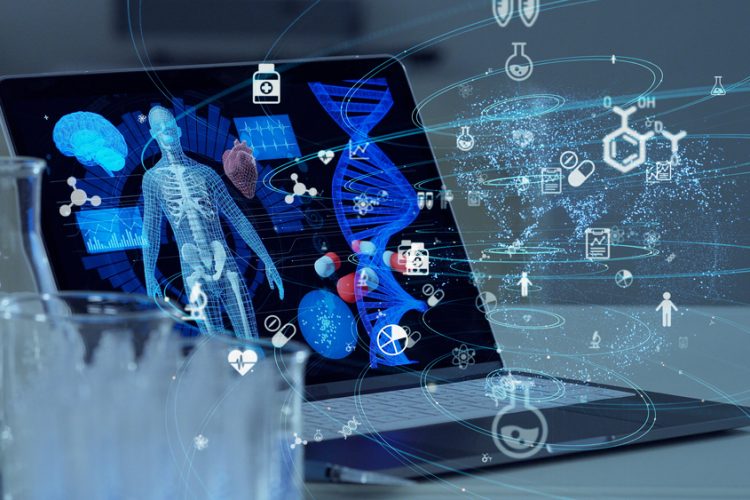
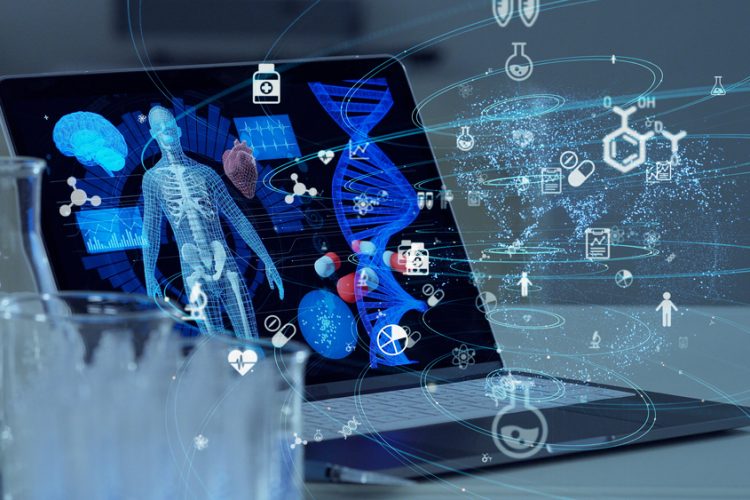
DeepCE (pronounced ‘Deep Sea’), a novel deep-learning computer model that can predict how gene expression will change in response to medicines, has been used to identify 10 compounds that could be repurposed as treatments for COVID-19.
Two of the drugs – cyclosporin, an immunosuppressant used to prevent transplant organ rejection, and anidulafungin, an antifungal agent – are currently approved for clinical use. The others are investigational and being evaluated in a wide range of indications, from hepatitis C and fungal disease to cancer and heart disease. According to the team, some of these candidates have already been evaluated in COVID-19 patients.
The computer scientists who developed DeepCE, a phenotype-based compound screening technology, said using artificial intelligence (AI) to discover these drugs has saved the pharma industry the time and money it would take to identify the compounds on a piecemeal basis. However, they cautioned that more studies would be required before any of these medications would be confirmed as safe and effective treatments for people infected with SARS-CoV-2.
“When no-one has any information on a new disease, this model shows how artificial intelligence can help solve the problem of how to consider a potential treatment,” said the study’s senior author, Ping Zhang, assistant professor of computer science and engineering and biomedical informatics at The Ohio State University, US.
The scientists explained that DeepCE was completed in May 2020, just as the first papers detailing how COVID-19 patients’ genes responded to the virus were published. The new information provided an important test for the computer model.
To make predictions about how genes and medicines will interact and yield drug repurposing candidates, DeepCE relies on two primary sources of publicly available data: L1000, a National Institutes of Health (NIH)-funded repository of human cell-line data showing how gene expression changes in response to specific drugs, and DrugBank, which contains information on the chemical structures and other details of about 11,000 approved and investigational drugs.
The researchers trained the DeepCE model by running all of the L1000 data through an algorithm against specific chemical compounds and their dosages. To fill in data gaps, the model converts chemical compound descriptions into figures, allowing for automatic consideration of their separate components’ effects on genes. In addition, for genes not represented in L1000, the team used a deep learning approach called an attention mechanism to increase the model’s “learned” sample of gene-chemical compound interactions, which improves the framework’s performance.
“This way, the output demonstrates multitask learning – we can predict gene expression values for new chemicals not from one cell to one cell, but automatically predict the role of a drug on different cell lines and different genes,” said Zhang, who leads the Artificial Intelligence in Medicine Lab and is a core faculty member in the Translational Data Analytics Institute at Ohio State. “We can use the computer to simulate drug-induced gene expression. This provides real value.”
To repurpose drugs for COVID-19, the team applied DeepCE’s gene expression prediction matrix – focusing on data from lung and airway cell lines and the entire DrugBank catalogue of compounds – to the genetic information provided by early COVID-19 papers and additional government data. The COVID-19 data demonstrated how human gene expression had responded to being infected with the SARS-CoV-2 virus, creating a “disease signature”.
Zhang explained that the model compares the gene expression pattern caused by known drugs to the disease signature, the expression profile caused by the disease. “Wherever we find the disease and a drug show opposite gene expression profiles, suggesting the drug would reverse the effects of the disease, you have found a drug that may treat the disease.”
Zhang concluded that DeepCE compliments a drug-repurposing model he described in a recent paper that simulates clinical trials using observational clinical data and that he wants to pull the resources together and work with collaborators to help find new drugs for diseases – including some that are yet to be discovered.
The study was published in Nature Machine Intelligence.
Related topics
Analysis, Analytical Techniques, Artificial Intelligence, Big Data, Cell-based assays, Drug Repurposing, Genomics, Therapeutics
Related conditions
Coronavirus, Covid-19
Related organisations
DrugBank, The Ohio State University
Related people
Ping Zhang