Computational method used to quantify tRNAs in any organism
Posted: 1 March 2021 | Victoria Rees (Drug Target Review) | No comments yet
Researchers have developed a new technique called mim-tRNAseq to measure, map and analyse tRNAs in cells, providing insight into disease.
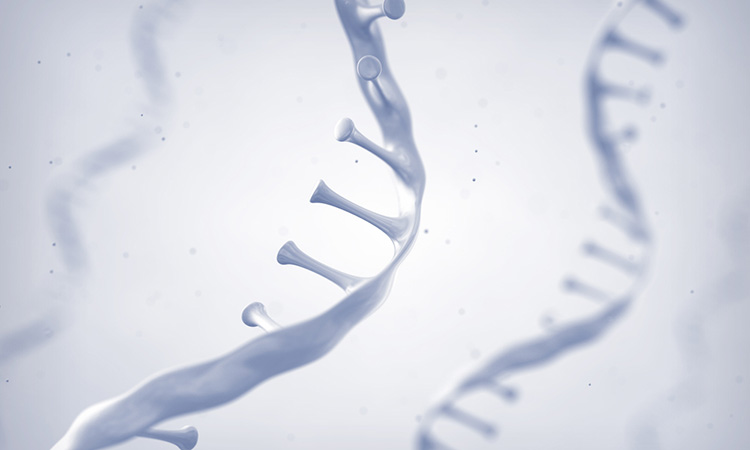
According to researchers, measuring the amount of transfer RNAs (tRNAs) in cells has been limited by technical challenges. Now, a team from the Max Planck Institute of Biochemistry, Germany, has overcome these limitations with mim-tRNAseq, a method that can be used to quantify tRNAs in any organism and will help improve understanding of its regulation in health and disease.
The researchers say that tRNA delivers specific amino acids to ribosomes during translation of messenger RNA into proteins. The abundance of these can therefore have a profound impact on cell physiology.
Furthermore, a cell contains several hundred thousand tRNA molecules, each of which consists of only 70 to 90 nucleotides folded into a cloverleaf-like pattern. At one end, they carry one of the 20 amino acids that serve as protein building blocks, while the opposite end pairs with the codon specifying this amino acid in messenger RNA during translation. Although there are only 61 codons for the 20 amino acids, cells from different organisms can contain hundreds of unique tRNA molecules, some of which differ from each other by only a single nucleotide. Many nucleotides in tRNAs are also decorated with chemical modifications, which help them fold or bind the correct codon.
tRNA defects are linked to neurogical diseases and cancer, but the molecular origins of these links remained unclear.
To measure the levels of multiple RNAs simultaneously, the researchers from the study used an enzyme called reverse transcriptase to first rewrite RNA into DNA. Millions of these DNA copies can then be quantified in parallel by high-throughput sequencing. Rewriting tRNAs into DNA has been difficult since many tRNA modifications block the reverse transcriptase, causing it to stop synthesising DNA.
“Many researches have proposed elegant solutions to this problem, but all of them relieve only a fraction of the modification roadblocks in tRNAs,” explained Danny Nedialkova, the lead researcher of the study. “We noticed that one specific reverse transcriptase seemed to be much better at reading through modified tRNA sites. By optimising the reaction conditions, we could significantly improve the enzyme’s efficiency, enabling it to read through nearly all tRNA modification roadblocks.”
This made it possible to construct DNA libraries from full-length tRNA copies and use them for high-throughput sequencing. However, the analysis of the resulting sequencing data also presented significant challenges.
“We identified two major issues: the first one is the extensive sequence similarity between different tRNA transcripts,” said Andrew Behrens, PhD student and first author of the paper. “The second one comes from the fact that an incorrect nucleotide (a misincorporation) is introduced at many modified sites during reverse transcription. Both make it extremely challenging to assign each DNA read to the tRNA molecule it originated from.”
The team tackled these issues with novel computational approaches, including the use of modification annotation to guide accurate read alignment. The resulting comprehensive toolkit is packaged into a freely available pipeline for alignment, analysis and visualisation of tRNA-derived sequencing data. Researchers can use mim-tRNAseq to not only measure tRNA abundance, but also to map and quantify modifications that induce nucleotide misincorporations by the reverse transcriptase.
“mim-tRNAseq opens up myriad possibilities moving forward,” said Nedialkova. “We expect it will help us and others to tackle many outstanding questions about tRNA biology in health and disease.”
The study was published in Molecular Cell.
Related topics
Amino Acids, Bioinformatics, Cell Cultures, Informatics, Next-Generation Sequencing (NGS), RNAs, Sequencing
Related conditions
Cancer
Related organisations
Max Planck Institute of Biochemistry
Related people
Andrew Behrens, Danny Nedialkova