Machine learning approaches as tools to accelerate drug discovery
Posted: 12 December 2017 | Alexey Zakharov, Anton Simeonov | No comments yet
Computational methods based on machine learning approaches are being introduced increasingly widely to screen the large number of molecules that have never participated in the drug discovery process, but which might have significant drug development potential. This article considers the latest advances in machine learning as applied to drug discovery…
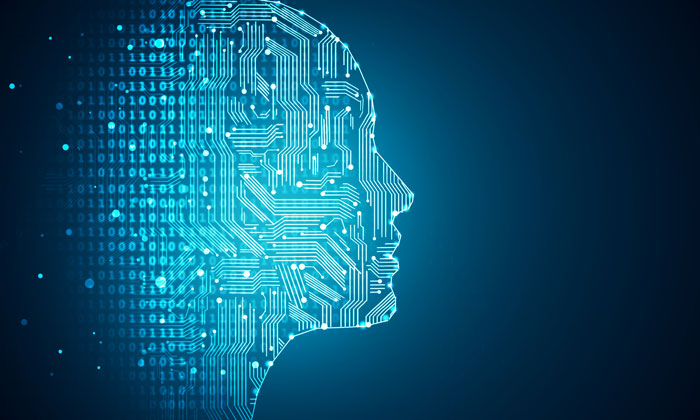
THE process of drug development is time consuming and costly. Several years are required for lead identification, optimisation, in vitro and in vivo testing, before the first clinical trials begin. The cost of developing a prescription drug that gains market approval, accounting for the very high failure rate, exceeds $2.5bn.1 Different approaches have been developed in recent years to accelerate and improve the success rate of the early drug discovery process, including high-throughput screening technology,2 3D culture screening,3 tissue printing4 and organs-on-chips technology.5 A significant advancement in the process of screening was introduced over a decade ago with quantitative high-throughput screening (qHTS),6 which allows the efficient identification of biological activities in chemical libraries by testing each library compound in a dose-response format. It was shown that qHTS can be successfully applied to finding new inhibitors for druggable7 and undruggable8 targets, epigenetics modulators,9 de-orphanisation of GPCR receptors,10 characterisation of cytochrome P450 isozyme selectivity across chemical libraries,11 and profiling of environmental chemicals’ potential to disrupt processes in the human body that may lead to negative health effects.12 qHTS can be used to screen large chemical libraries,13 producing high-quality chemical genomic data sets, which can be made publicly available through sites such as PubChem.
However, despite the high-throughput capacity made available by modern technologies such as assay miniaturisation and screening robotics, the number of compounds which can be screened using qHTS or traditional single-concentration screening is still limited by reagents and labour costs, especially as screening assays evolve into more complex model systems and physical formats. Thus, these limitations restrict the chemical space of screening compounds and might be the reason for failures to develop new chemical probes or hit compounds.
Indeed, the industry and other organisations typically screen chemical libraries which consist of between half a million and a million compounds. However, the number of commercially available compounds currently exceeds 85 million.14 It has also been estimated that the possible chemical space for small molecules consisting of 30 or fewer heavy atoms exceeds 1060 molecules.15 Therefore, an exceedingly large number of molecules have never participated in the drug discovery process, but might have significant drug development potential.
Quantitative structure-activity relationship (QSAR)
To fill this gap, computational methods based on machine learning approaches are being introduced increasingly widely. One of the most powerful methods is a quantitative structure-activity relationship (QSAR).16 This approach constructs a model which describes relationships between chemical structures and their biological responses to a specific target, assay, or disease. To develop a model, first, the chemical structures need to be characterised or described by molecular descriptors. Current software17,18 allows for the calculation of over 6,000 molecular descriptors for a particular compound, which cover different characteristics of molecules, including: physicochemical properties (molecular weight, LogP, etc); fragments of the molecule (CH2R2, CH2RX, etc); structural features (number of rings, number of triple bonds, etc); functional group counts (number of sulfoxides, aromatic group, thioacids, etc); quantum-chemical properties (electron-correlation contribution, electron affinity, ionisation potential, etc). This large amount of descriptors provides a good ability to identify the molecules with the best characteristics to properly describe the drug-target interactions.
The aim of machine learning approaches in QSAR is to establish relationships between molecular descriptors and their biological responses. Different endpoints are used to quantify biological responses. Explicit values can be obtained by measuring the concentration of a compound where 50% of its maximal effect is observed (eg, inhibitory, effective, activity, or lethal concentrations). Categorical responses are often used if compounds are active or inactive at certain concentrations (1μM, 10μM or 100μM). Thus, depending on the end point, the QSAR models can be separated into regression models for quantitative data and classification models for categorical data.
To construct QSAR models, various machine learning approaches are used. The most known are Bayesian, decision trees, fuzzy logic, genetic algorithms, multiple regressions (MLR), partial least squares, radial basis function, support vector machines (SVF), random forest (RF), and neural networks (NN). Some of the methods are relatively simple, like multiple regressions, decision trees or naïve Bayes, and can be clearly interpreted. Indeed, coefficients obtained from MLR easily explain the contribution of each descriptor into structure-activity relationships. Other approaches, like SVM, RF, or NN, are most powerful, but difficult to interpret. The models obtained using these approaches are sophisticated and are often called ‘black box’.
However, recent studies19,20 suggest some methods for interpretation of complicated machine learning techniques. Most of these use an estimation of molecular fragments’ contributions to activity value, and as a result, they suggest which part of a molecule must be modified to increase or decrease its activity.21,22 Developed QSAR models are broadly used for the main computational tasks of drug discovery: virtual screening of additional active compounds with attractive chemotypes and structure optimisation of already-identified hit compounds. Since QSAR models are easy to deploy, virtual screening can be carried out for libraries of millions of compounds commercially available from vendors or even billions of compounds generated in silico with further possible synthesis.
Virtual screening of targets of phenotypic outcomes
It was shown23 that QSAR models have been used for virtual screening of different targets or phenotypic outcomes. For example, Guasch et al24 have developed the QSAR model to predict HIV-1 integrase strand transfer inhibitors and applied it to a combinatorial library of 29,736 compounds generated in silico. Some of the hit compounds were synthesised and confirmed in biological experiments. Pantaleao et al25 have developed QSAR models for virtual screening of new drugs for the treatment of type 2 diabetes. QSAR models are actively used for virtual screening by the pharmaceutical industry, eg, Martin et al26 showed that activity predictions obtained by in-house QSAR models were statistically comparable to medium-throughput four-concentration IC50 measurements derived from qHTS screening. Sheridan et al27 described the use of QSAR models for predictions of off-target activities for drug discovery projects.
Another application of QSAR models is in silico hit-to-lead optimisation. Such an optimisation can be done using only primary activity data or taking into account multiple biological activities (eg, off-targets effects), physical-chemical (solubility, permeability) or ADME (absorption, distribution, metabolism and excretion) properties. For example, Kokurkina et al22 used QSAR models for design and optimisation of compounds against six antifungal activities. Several developed compounds were found to be the most active against all six fungi. Fedorova et al28 developed predictive QSAR models for the design of vanadium-containing complexes as antidiabetic agents. The developed compounds were tested in vitro and in vivo and showed the improved activity. More examples can be found elsewhere.29,30,31
Recent developments in machine learning reveal a new approach called deep learning.32,33 Deep learning is a neural net which typically has two or more hidden layers. The number of hidden layers is the main feature besides many others, which distinguish classical or shallow neural nets and deep nets. Previously, many attempts to build the neural net with more than one hidden layer were faced with a vanishing gradient problem,34 which leads to loss of model predictivity. A little over a decade ago, several methods (long short-term memory, deep belief network, dropout) were proposed to overcome this problem.35,36,37
Intensive research in this area has produced different types of deep learning algorithms and architectures, which are used to construct the current models: deep believe nets;36 convolutional nets;38 recurrent neural nets;35,39 stacked autoencoders;40 and others.41 During the past five years deep learning techniques have become dominant in the area of speech recognition, image classification, natural language processing, motion detection, and others.32
The superiority of deep learning over other machine learning approaches for drug discovery purposes was revealed by two independent challenges/competitions organised by a pharmaceutical company (Merck) in 201242 and a government research division (National Center for Advancing Translational Sciences (NCATS)) in 2014.43 The goal of Merck’s competition was to predict 15 biological activities for blind sets, while the NCATS challenge was focused on prediction of compound bioactivity in 12 toxicity-related assays screened through the Tox 21 initiative.12 In both challenges the winners were teams using deep learning approaches for model development. It was shown44 that deep nets allow not only for the capture of different degrees of abstraction by varying numbers of layers and their sizes to avoid feature engineering by translating the data into compact intermediate representation, but also for building a model using huge amounts of data, and to construct a multitask model33 which borrows the knowledge across the different tasks.
Multitask, or joint, learning allows one to solve multiple different tasks, or in the case of QSAR the multiple biological activities of compounds, at the same time. Thus, the biological activities embedded in a joint deep learning model share the same feature representations as well as weights and bias in the hidden layers, but have their own unique weights and bias in the output layer. Several studies have shown45,46 that multitask learning improves the prediction accuracy for the related task models, compared to models trained separately. Korotcov et al47 have compared the deep learning approach with multiple state-of-the-art machine learning methods using different diverse drug discovery data sets and showed the superiority of deep learning. Altae-Tran et al48 have developed new deep learning architecture, which can be used to significantly lower the amounts of data required to make meaningful predictions in drug discovery applications. They have shown that this architecture offers significant boosts in predictive power for a variety of problems meaningful for computational drug discovery. Thus, these studies clearly demonstrate that deep learning approaches are changing the paradigm of ligand-based modeling.
Since deep learning algorithms are capable of automated feature learning with different degrees of abstraction, incorporating different types of omics data,49 such as genomics, proteomics, metabolomics, transcriptomics, together with qHTS data gathered from multiple assays into a single model, they offer new opportunities to develop predictive models and accelerate drug discovery processes.50 In addition, these methods open up a great avenue for providing some insight into target deconvolution of phenotypic screening hits, discovering novel mechanisms of action, selecting candidates for drug repurposing, and even developing candidates for personalised medicine. Thus, more examples of advances in this field are highly anticipated in the near future.
Acknowledgements
We thank members of the Informatics team for discussions on the topics reviewed here, including Tongan Zhao, Dac-Trung Nguyen, and Noel Southall.
Biography
ALEXEY ZAKHAROV joined the Informatics group at the National Center for Advancing Translational Sciences (NCATS), NIH in 2015. His work focuses on supporting drug discovery projects, analyses of screening data, identification of chemical series for lead optimisation and follow-up studies, developing and applying QSAR models for virtual screening of compounds through large chemical libraries, and supporting medicinal chemistry studies. Dr Zakharov received his PhD in Bioinformatics from the Institute of Biomedical Chemistry, Russian Academy of Medical Sciences.
ANTON SIMEONOV is the Scientific Director of the Division of Pre-Clinical Innovation (DPI) at the National Center for Advancing Translational Sciences (NCATS). He received his PhD in Bioorganic Chemistry from the University of Southern California. Dr Simeonov’s current research interests include novel detection chemistries and techniques, assays and devices for diagnostics, assay miniaturisation, and novel approaches to screening and therapeutics development.
References
- Mullin, R. Tufts Study Finds Big Rise In Cost Of Drug Development. Chemical & Engineering News. https://cen.acs.org/articles/92/web/2014/11/Tufts-Study-Finds-Big-Rise.html (accessed Nov 28, 2017).
- Seo J, Shin J-Y, Leijten J, Jeon O, Camci-Unal G, Dikina, AD, Brinegar K, Ghaemmaghami AM, Alsberg E, Khademhosseini A. High-Throughput Approaches for Screening and Analysis of Cell Behaviors. Biomaterials 2018;153:85-101.
- Breslin S, O’Driscoll L. Three-Dimensional Cell Culture: The Missing Link in Drug Discovery. Drug Discov Today. 2013; 18 (5-6):240-249.
- Zhang YS, Yue K, Aleman J, Moghaddam KM, Bakht SM, Yang J, Jia W. Dell’Erba V, Assawes P, Shin SR, Dokmeci MR. Oklu R, Khademhosseini A. 3D Bioprinting for Tissue and Organ Fabrication. Ann Biomed Eng. 2017;45(1):148-163.
- Balijepalli A, Sivaramakrishan V. Organs-on-Chips: Research and Commercial Perspectives. Drug Discov Today. 2017;22(2):397–403.
- Inglese J, Auld DS, Jadhav A, Johnson RL, Simeonov A, Yasgar A, Zheng W, Austin CP. Quantitative High-Throughput Screening: A Titration-Based Approach That Efficiently Identifies Biological Activities in Large Chemical Libraries. Proc Natl Acad. Sci USA. 2006;103(31):11473–11478.
- Li S, Huang R, Solomon S, Liu Y, Zhao B, Santillo MF, Xia M. Identification of Acetylcholinesterase Inhibitors Using Homogenous Cell-Based Assays in Quantitative High-Throughput Screening Platforms. Biotechnol J. 2017;12(5).
- Yasgar A, Titus SA, Wang Y, Danchik C, Yang S-M, Vasiliou V, Jadhav A, Maloney DJ, Simeonov A, Martinez NJ. A High-Content Assay Enables the Automated Screening and Identification of Small Molecules with Specific ALDH1A1-Inhibitory Activity. PLoS ONE. 2017;12(1).
- Hsu C-W, Shou D, Huang R, Khuc T, Dai S, Zheng W, Klumpp-Thomas C, Xia, M. Identification of HDAC Inhibitors Using a Cell-Based HDAC I/II Assay J Biomol Screen. 2016;21(6):643–652.
- Chen CZ, Southall N, Xiao J, Marugan JJ, Ferrer M, Hu X, Jones RE, Feng S, Agoulnik IU, Zheng W, Agoulnik AI. Identification of Small-Molecule Agonists of Human Relaxin Family Receptor 1 (RXFP1) by Using a Homogenous Cell-Based CAMP Assay. J Biomol Screen. 2013;18(6):670–677.
- Veith H, Southall N, Huang R, James T, Fayne D, Artemenko N, Shen M, Inglese J, Austin CP, Lloyd DG, Auld DS. Comprehensive Characterization of Cytochrome P450 Isozyme Selectivity across Chemical Libraries. Nat Biotechnol. 2009;27 (11):1050-1055.
- Huang R, Xia M, Cho MH,Sakamuru S, Shinn P, Houck KA, Dix DJ, Judson RS, Witt KL, Kavlock RJ, Tice RR, Austin CP. Chemical Genomics Profiling of Environmental Chemical Modulation of Human Nuclear Receptors. Environ Health Perspect. 2011;119(8):1142-1148.
- Crowe A, Huang W, Ballatore C, Johnson RL, Hogan A-ML, Huang R, Wichterman J, McCoy J, Huryn D, Auld DS, Smith AB, Inglese J, Trojanowski JQ, Austin CP, Brunden KR, Lee VM-Y. Identification of Aminothienopyridazine Inhibitors of Tau Assembly by Quantitative High-Throughput Screening. Biochemistry (Mosc.) 2009;48(32):7732-7745.
- ChemNavigator https://www.chemnavigator.com/cnc/products/iRL.asp (accessed Nov 28, 2017).
- Bohacek RS, McMartin C, Guida WC. The Art and Practice of Structure-Based Drug Design: A Molecular Modeling Perspective. Med Res Rev. 1996;16(1):3-50.
- Tropsha A. Best Practices for QSAR Model Development, Validation, and Exploitation. Mol Inform. 2010;29(6-7):476-488.
- Dragon 6 user’s manual. http://www.talete.mi.it/help/dragon_help/index.html (accessed Dec 9, 2014).
- Hong H, Xie Q, Ge W, Qian F, Fang H, Shi L, Su Z Perkins R, Tong W. Mold2, Molecular Descriptors from 2D Structures for Chemoinformatics and Toxicoinformatics. J Chem Inf Model. 2008;48(7):1337-1344.
- Polishchuk P. Interpretation of Quantitative Structure-Activity Relationship Models: Past, Present, and Future. J Chem Inf Model. 2017;57(11):2618-2639.
- Polishchuk P,Tinkov O, Khristova T, Ognichenko L, Kosinskaya A, Varnek A, Kuz’min V. Structural and Physico-Chemical Interpretation (SPCI) of QSAR Models and Its Comparison with Matched Molecular Pair Analysis. J Chem Inf Model. 2016;56(8): 1455-1469.
- Polishchuk PG, Kuz’min VE, Artemenko AG, Muratov EN. Universal Approach for Structural Interpretation of QSAR/QSPR Models. Mol Inform. 2013;32(9-10):843-853.
- Kokurkina GV, Dutov MD, Shevelev SA, Popkov SV, Zakharov AV, Poroikov VV. Synthesis, Antifungal Activity and QSAR Study of 2-Arylhydroxynitroindoles. Eur J Med Chem. 2011;46 (9):4374–4382.
- Wang T, Wu M-B, Lin J-P, Yang L-R. Quantitative Structure-Activity Relationship: Promising Advances in Drug Discovery Platforms. Expert Opin Drug Discov. 2015;10(12):1283-1300.
- Guasch L, Zakharov AV, Tarasova OA, Poroikov VV, Liao C, Nicklaus MC. Novel HIV-1 Integrase Inhibitor Development by Virtual Screening Based on QSAR Models. Curr Top Med Chem. 2016;16(4):441-448.
- Pantaleao SQ, Fujii DGV, Maltarollo VG, da C Silva D, Trossini GHG, Weber KC, Scott LPB, Honorio KM. The Role of QSAR and Virtual Screening Studies in Type 2 Diabetes Drug Discovery. Med Chem. Shariqah United Arab Emir. 2017;13(8):706-720.
- Martin EJ, Polyakov VR, Tian L, Perez RC. Profile-QSAR 2.0: Kinase Virtual Screening Accuracy Comparable to Four-Concentration IC50s for Realistically Novel Compounds. J Chem Inf Model. 2017;57(8):2077–2088.
- Sheridan RP. Global Quantitative Structure–Activity Relationship Models vs Selected Local Models as Predictors of Off-Target Activities for Project Compounds. J Chem Inf. Model. 2014;54(4):1083–1092.
- Fedorova EV, Buryakina AV, Zakharov AV, Filimonov DA, Lagunin AA, Poroikov VV. Design, Synthesis and Pharmacological Evaluation of Novel Vanadium-Containing Complexes as Antidiabetic Agents. PloS One. 2014;9(7):e100386.
- Kuz’min VE, Artemenko AG, Muratov EN. Hierarchical QSAR Technology Based on the Simplex Representation of Molecular Structure. J Comput Aided Mol Des. 2008;22(6-7):403-421.
- Kuz’min VE, Artemenko AG, Muratov EN, Volineckaya IL, Makarov VA, Riabova O. B, Wutzler P, Schmidtke M. Quantitative Structure-Activity Relationship Studies of [(Biphenyloxy)Propyl]Isoxazole Derivatives. Inhibitors of Human Rhinovirus 2 Replication. J Med Chem. 2007;50(17):4205–4213.
- Pan J, Zhang Y, Ran T, Xu A, Qiao X, Yin L, Zhou W, Zhu L, Zhao J, Lu T, Chen Y, Jiang Y. QSAR Modeling and in Silico Design of Small-Molecule Inhibitors Targeting the Interaction between E3 Ligase VHL and HIF-1[Formula: See Text]. Mol Divers. 2017.
- Schmidhuber J. Deep Learning in Neural Networks: An Overview. Neural Netw.2015;61:85-117.
- Ma J, Sheridan RP, Liaw A, Dahl GE, Svetnik V. Deep Neural Nets as a Method for Quantitative Structure–Activity Relationships. J Chem Inf Model. 2015;55(2):263-274.
- Hochreiter S, Bengio Y, Frasconi P, Schmidhuber, J. Gradient Flow in Recurrent Nets: The Difficulty of Learning Long-Term Dependencies; 2001.
- Hochreiter S, Schmidhuber J. Long Short-Term Memory. Neural Comput. 1997;9(8):1735-1780.
- Hinton GE, Osindero S, Teh Y-W. A Fast Learning Algorithm for Deep Belief Nets. Neural Comput. 2006;18(7):1527-1554.
- Hinton GE, Srivastava N, Krizhevsky A, Sutskever I, Salakhutdinov RR. Improving Neural Networks by Preventing Co-Adaptation of Feature Detectors. ArXiv12070580 Cs 2012.
- Gradient-based learning applied to document recognition – IEEE Journals & Magazine http://ieeexplore.ieee.org/document/726791/ (accessed Nov 28, 2017).
- Sak H, Senior A, Beaufays F. Long Short-Term Memory Based Recurrent Neural Network Architectures for Large Vocabulary Speech Recognition. ArXiv14021128 Cs Stat 2014.
- Vincent P, Larochelle H, Lajoie I, Bengio Y, Manzagol, P-A. Stacked Denoising Autoencoders: Learning Useful Representations in a Deep Network with a Local Denoising Criterion. J Mach Learn Res. 2010;11:3371–3408.
- Bengio Y. Learning Deep Architectures for AI. Trends® Mach Learn. 2009;2(1): 1-127.
- Merck Molecular Activity Challenge https://www.kaggle.com/c/MerckActivity (accessed Nov 28, 2017).
- Tox21 Data Challenge 2014 https://tripod.nih.gov/tox21/challenge/ (accessed Nov 28, 2017).
- Bengio Y, Courville A, Vincent P. Representation Learning: A Review and New Perspectives. ArXiv12065538 Cs 2012.
- Ramsundar B, Kearnes S, Riley P, Webster D, Konerding D, Pande V. Massively Multitask Networks for Drug Discovery. ArXiv150202072 Cs Stat 2015.
- Ramsundar B, Liu B, Wu Z, Verras A, Tudor M, Sheridan RP, Pande V. Is Multitask Deep Learning Practical for Pharma? J Chem Inf Model. 2017;57(8):2068–2076.
- Korotcov A, Tkachenko V, Russo DP, Ekins S. Comparison of Deep Learning With Multiple Machine Learning Methods and Metrics Using Diverse Drug Discovery Data Sets. Mol Pharm. 2017.
- Altae-Tran H, Ramsundar B, Pappu AS, Pande V. Low Data Drug Discovery with One-Shot Learning. ACS Cent Sci 2017;3(4):283-293.
- Mamoshina P, Vieira A, Putin E, Zhavoronkov A. Applications of Deep Learning in Biomedicine. Mol Pharm. 2016;13(5):1445-1454.
- Aliper A, Plis S, Artemov A, Ulloa A, Mamoshina P, Zhavoronkov A. Deep Learning Applications for Predicting Pharmacological Properties of Drugs and Drug Repurposing Using Transcriptomic Data. Mol Pharm. 2016;13(7):2524–2530.
Related topics
Drug Development, Drug Targets, High-Throughput Screening (HTS), Informatics, Lab Automation, Screening, Technology
Related conditions
Type-2 diabetes
Related organisations
NIH Center for Advancing Translational Sciences (NCATS)
Related people
Alexey Zakharov, Anton Simeonov