Adapting drug discovery to Artificial Intelligence
Posted: 25 July 2018 | Ami S Lakdawala (GSK's In-silico drug discovery unit), George Okafo (GSK's In-silico drug discovery unit), John Baldoni (GSK's In-silico drug discovery unit), Michael Palovich (GSK's In-silico drug discovery unit), Tobias Sikosek (GSK's In-silico drug discovery unit), Vishal Sahni (GSK's In-silico drug discovery unit) | No comments yet
Drug discovery has always been challenging; today, more so than ever. While there has been success in addressing many diseases, others remain intractable…
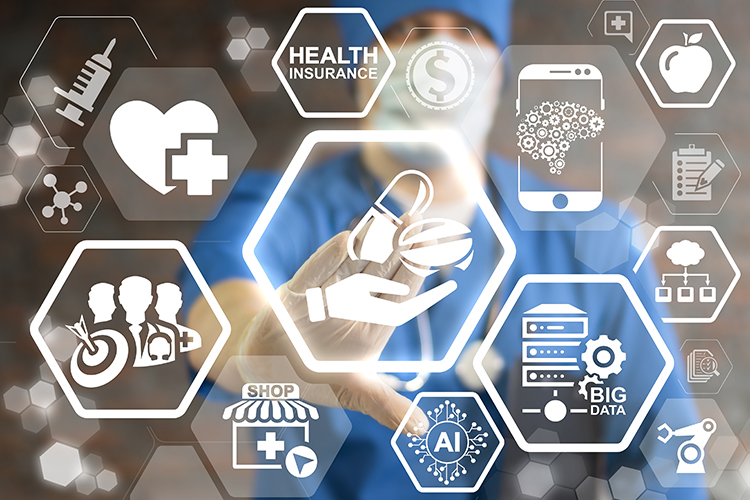
Understanding the pathway / finding the targets
In select diseases, AI / ML can accelerate identification of pathways and targets to modulate disease biology, by integrating 1, genomic information; 2, high content, multi-dimensional image analysis in human-relevant disease phenotypes; 3, deep feature selection of the pathology for pathway reconstruction and pathway scoring; and 4, target tractability assessments based upon accessibility, modality and molecular design. In combination with public-private initiatives such as Open Targets,1 that use human genetics and genomics data for systematic drug target identification and prioritisation, we use deep learning to correlate genes / targets with the potential to modulate diseases that do not currently have disease-modifying or targeted treatments.
Finding the molecule
Identifying small molecules that modulate disease biology is centred on three components: virtual chemical space as the source of molecules; AI / ML techniques to identify the target-specific virtual molecules; and a suite of predictive models / algorithms to associate the molecule with target features, optimising concomitantly on both favourable safety and efficacy attributes. Molecules are prepared and assessed in physical systems to test the design attributes and the empirical data fed back into the molecule generation algorithm to reinforce learning. We anticipate this approach will have an impact on cycle time and success rates by reducing the number of molecules synthesised and tested in in-vitro and in-vivo systems.
The virtual chemical space (estimated as 1024 molecules with drug-like criteria) offers a real opportunity to identify novel, high quality molecules. It also represents a challenge to search and extract the molecules that match desired profiles. A panel of in-silico techniques, at varying degrees of validation, are available and can be selected based upon the data available to drive the decision. For example, virtual ligand or structure-based design approaches are used when probe small molecule modulators or structural biology data are available.2 When structural data are lacking, deep learning platforms based on phenotypic data, or disease / biology / molecule network-based algorithms can be used.3-5 Some of these techniques are well characterised, while others are early and require validation or refinement to reach their full value. The unit will utilise established and emerging techniques to increase the probability of success and explore algorithm diversity.
A rate-limiting step is synthesising molecules selected from a virtual chemical space – they often lack published or challenging synthetic routes, which can cause delays. The ability to prioritise molecules based on ease of synthesis, or the development of tools that are effective in devising the optimal synthetic route, are needed and are progressing rapidly.6 This is something we are exploring at GSK.
How the molecule interacts with biology
It is desirable to be able to predict a molecule’s in vivo safety profile before it is synthesised or progressed. This would reduce the attrition rate.7 It is also desirable to be able to predict the on-target effects as well as any off-target pharmacological effects. Several AI / ML approaches are underway in this area including their application to the Tox21 challenge winner,8 DeepTox©9 and PrOCTOR© (used for clinical trial outcomes).10 Each of these predictors has its specific application. To improve these predictors, larger and better datasets will be needed from a diverse set of molecules, disease indications, and toxicological findings. This requires a paradigm shift and the creation of a mechanism that allows more openness and sharing of data amongst the major industry players. Initiatives such as the public-private ATOM consortium are a first step in this direction.11 At the same time, more powerful deep learning approaches are constantly being developed by social media giants such as Google and these are now quickly being adopted into healthcare.12
Selecting patients to test the clinical hypothesis, operationalising trials and predicting outcomes
To this point, a connected workflow has been described that associates the disease and patient to the pathway; to the patient gene targets; to the molecules designed; to on- and off-target effects. The ultimate test is that these designed molecules can modulate this disease in this patient phenotype. Hence, patient selection is critical at this point. In an ideal workflow, the patient’s disease would be defined mathematically, which should be any drug-developer’s aspiration in an in-silico enabled environment. At this stage, the workflow and data should have revealed phenotypic, multi- ‘omics biomarkers of disease based upon the human-relevant, empirical phenotypic models used to corroborate the genetic association that underpins the medicine hypothesis.
The unit’s intent is to begin with a specific patient phenotype and move to a more heterogeneous patient phenotype, directed by in-vitro and biomarker determinations of potential response predicted by a pathway specific in-vitro / in-vivo translation algorithm. Linking biomarker and in-vitro phenotype patient data to therapy affords a more predicted, quantifiable assessment of the uncertainty of therapeutic response in a specific patient.
Over time, quantifiable, precise, reliable data will be collected on disease specific phenotypes and healthy subjects. In this context, the development of AI / ML approaches to identify and predict patterns will allow more informed and outcome-relevant clinical trials13 (Figure 2).
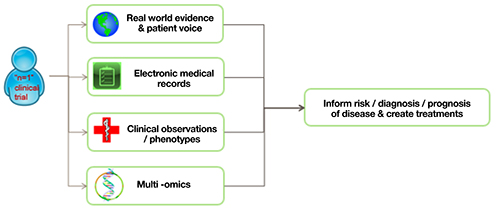
Figure 2 Clinical dataset examples.
These data can be available to simulate a trial during its design phase and to inform its direction based on intermediate outcomes, predicted by multidimensional analyses of existing data. For example, algorithm-driven dynamic decisions to adapt individual participants to emerging information (eg, randomisation to appropriate trial arms, dose administered based upon patient unique physiological, behavioural, genetic profile, disease progression, etc). This type of trial is already being affected by data-driven algorithms. Coupling this with some of the concepts above could mean that fewer trial participants would be needed to show a statistically significant intervention response due to a reduction in data variability and concomitant increase of statistical power.
If successful, this approach will enable the industry to design and conduct clinical trials that have been highly informed by predictive AI / ML models and have the potential to increase success rates in the future.
Biographies
GEORGE OKAFO is Senior Director at GlaxoSmithKline’s (GSK’s) In-silico drug discovery unit, Stevenage. George has over 28 years’ experience in drug discovery and development and has led numerous drug discovery and development projects through to IND, MAA and NDA, including diseases of the developing world portfolio. George has a BSc (Chemistry and Biochemistry) and PhD (Chemical Carcinogenesis) from Imperial College, London and completed a Postdoctoral Research Fellowship (Cancer Chemistry), University of Toronto, Canada. George is a member of Royal College of Sciences (ARCS), Fellow Royal Society of Chemistry (FRSC), Society for Chemical Industry (SCI) and Science Innovation Advisory Committee, UK Bio-Industry Association (SIAC, UK BIA) and a STEM ambassador.
JOHN BALDONI is Senior Vice President and Head of GSK’s In-silico drug discovery unit, Philadelphia, USA. He has over 30 years’ experience in the pharmaceutical industry and has held numerous senior executive positions at GSK including Senior Vice President and Global Head of Platform Technology and Sciences (PTS). John has been instrumental in driving innovative thinking in GSK and recently proposed the creation of the in-silico drug discovery unit. John is also the co-founder and Chairman of the Accelerating Therapeutics for Opportunities in Medicine (ATOM) Consortium.
MIKE PALOVICH is Senior Scientific Director, In-silico drug discovery unit, Philadelphia, USA. After his BSc, he received a PhD degree in organic chemistry from the University of Pittsburgh and did postdoctoral research studies at the University of Virginia. Mike joined GSK in 1998 and has spent much of his career in the drug discovery part of the organisation performing small molecule lead discovery and lead optimisation activities across several therapeutic and disease areas.
VISHAL SAHNI is Director, R&D Esprit Associate, in GSK’s In-silico drug discovery unit, Stevenage, UK, with 10 years of experience in the pharmaceutical industry. He has led global project teams at several stages of the drug discovery, development and commercialisation value chain and is currently focused on applying AI / ML methodologies in translational pre-clinical R&D and Clinical Development. Vishal holds a PhD (Developmental Neurobiology) from University College London, a Master’s degree (Experimental Neuroscience) from Imperial College London and a BSc degree (Biomedical Science) from King’s College London.
AMI S LAKDAWALA is Director of Operations, In-silico drug discovery unit, Heidelberg, Germany and has over 14 years of experience in pharmaceutical R&D. Ami has a BSc (Chemistry and Mathematics) from Texas Christian University and PhD (Organic Chemistry) from Emory University, and started her career in GSK as a computational chemist.
TOBIAS SIKOSEK is a Senior Data Scientist, In-silico drug discovery unit, Heidelberg, Germany. Tobias is working on machine learning solutions for various data types to accelerate drug discovery. His background is in computational and theoretical protein evolution with a PhD from the University of Muenster, Germany. He is based at GSK’s Cellzome site on the Heidelberg campus of the European Molecular Biology Labs (EMBL) in Germany.
References
- Koscielny G, et al. Open Targets: a platform for therapeutic target identification and validation. Nucleic Acids Res, 2017. 45(D1): p. D985-D994.
- Reymond J-L. The Chemical Space Project; Acc. Chem. Res. 2015, 48; p722−730.
- Filzen TM, et al. Representing high throughput expression profiles via perturbation barcodes reveals compound targets. PLoS Comput Biol, 2017. 13(2): p. e1005335.
- Ozerov IV, et al. In silico Pathway Activation Network Decomposition Analysis (iPANDA) as a method for biomarker development. Nat Commun, 2016. 7: p. 13427.
- Tan J, et al. Unsupervised Extraction of Stable Expression Signatures from Public Compendia with an Ensemble of Neural Networks. Cell Syst, 2017. 5(1): p. 63-71 e6.
- Segler MHS, Preuss M, Waller MP. Planning chemical syntheses with deep neural networks and symbolic AI. Nature, 2018. 555(7698): p. 604-610.
- Waring MJ, et al. An analysis of the attrition of drug candidates from four major pharmaceutical companies. Nat Rev Drug Discov, 2015. 14(7): p. 475-86.
- Thomas RS, et al. The US Federal Tox21 Program: A strategic and operational plan for continued leadership. ALTEX, 2018. 35(2): p. 163-168.
- Mayr A, et al. DeepTox: Toxicity Prediction using Deep Learning. Frontiers in Environmental Science, 2016. 3(80).
- Gayvert KM, Madhukar NS, Elemento O. A Data-Driven Approach to Predicting Successes and Failures of Clinical Trials. Cell Chem Biol, 2016. 23(10): p. 1294-1301.
- GlaxoSmithKline. Public-private consortium aims to cut preclinical cancer drug discovery from six years to just one. 2017 [cited 2018 27 May]; Available from: http://www-origin.gsk.com/engb/media/press-releases/public-private-consortium-aims-to-cut-preclinical-cancer-drug-discovery from-six-years-to-just-one/.
- Google. Predicting Properties of Molecules with Machine Learning 2017 [cited 2018 27 May]; Available from: https://ai.googleblog.com/2017/04/predicting-properties-of-molecules-with.html.
- Schork NJ. Personalized medicine: Time for one-person trials. Nature, 2015. 520(7549): p. 609 11.
Related topics
Analytical Techniques, Artificial Intelligence, Disease Research, Drug Discovery, Therapeutics