Laboratory automation in early drug discovery
Posted: 26 March 2020 | Darren Plant (AstraZeneca), Dr David Murray (AstraZeneca), Dr Mark Wigglesworth (AstraZeneca), Geoff Holdgate (AstraZeneca), Helen Plant (AstraZeneca), John Vincent (AstraZeneca), Paul Harper (AstraZeneca) | No comments yet
The future of drug discovery lies in an automated world where the workflows for biological assays, chemical synthesis and data analysis are connected by flexible, mobile and modular hardware, integrated with software solutions that will interface with scientists for increased efficiency and productivity (the realisation of Industry 4.0). This article explores these concepts through the eyes of researchers at AstraZeneca’s High-Throughput Screening Centre.
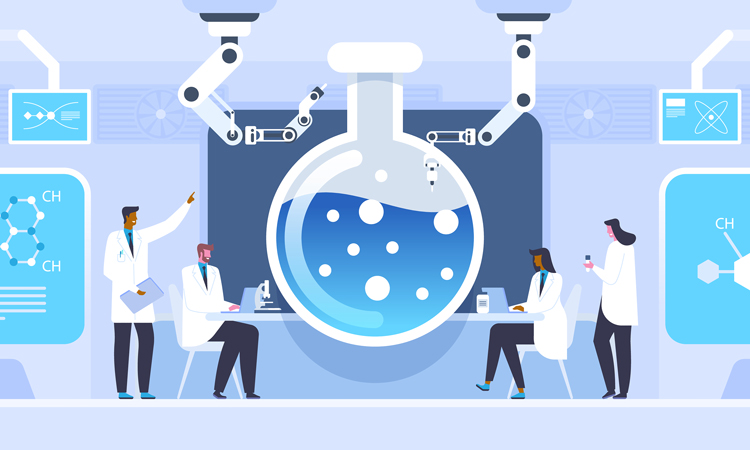
DRUG DISCOVERY is a complex and costly process, with the average cost recently estimated at almost $2.9 billion per approval. It has long been appreciated that improving large pharma’s poor track record on productivity requires an increase in the number and quality of new medicines, while preventing the unsustainable rise in R&D costs.2 AstraZeneca has historically focused on a 5R framework3 to improve decision making and productivity, with substantial success. However, with success rates remaining below 20 percent, there is continued focus on opportunities to improve. As pharma companies pursue these goals, automation will be a key component in future research and development. Throughout the drug discovery value chain, automation has the potential to both increase laboratory efficiency and decrease costs. New technologies, as well as new applications including the combination with artificial intelligence (AI), and automated data analysis procedures are reducing the time to agency approval and the number of scientists needed per data point. However, there are additional benefits, alongside the reduced costs and decreased timelines, that make automated processes essential for the future of drug discovery. Investment in automation permits the safer generation of data with improved accuracy, precision, reproducibility and traceability, allowing drug discovery scientists to exploit higher‐quality data in the hypothesis‐driven research required to discover new medicines. Further to this, automation allows greater numbers of hypotheses to be tested and can enable complex workflows and screening scenarios that simply cannot be achieved reliably by a human.
Speed and accuracy
High‐throughput screening (HTS) is critical to delivering starting points to drug discovery projects and remains the most successful approach across large portfolios.4 The automation of biological assays plays a key role in HTS, utilising a wide range of commercial equipment for screening purposes,5 including reagent dispensers,6 plate washers7,8 and multiparameter plate readers. Both speed and accuracy are important in the HTS environment, where equipment is integrated onto automation platforms. HTS labs are required to screen large numbers of compounds (1‐3 million), employing a variety of assay formats. Implementing robust automation that can be adapted to new technologies is, therefore, key for success in this field. Consequently, screening automation systems can be some of the most complex laboratory automation designs, incorporating multiple robots and a diverse range of peripheral equipment.
An automated approach
HTS of a wide array of biochemical and cell-based assay formats underpins success across large drug
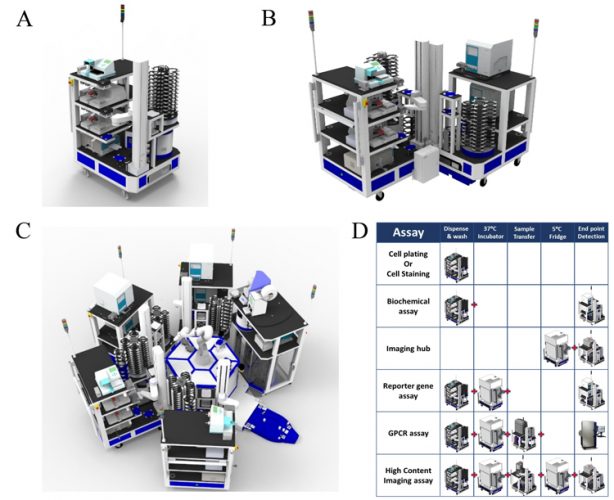
Figure 1: Modular automation used for biochemical and cell-based screening. Easily scaled automation (A) A single CoLAB Flex cart (High Res® Biosolutions, Beverly MA) which can be combined with another Flex cart on a semi-automated platform (B) or with several Flex carts centred around a central robot on a Microstar automation system (C). Task based automation is versatile to a variety of assay formats and library size, from simple mix-&-measure biochemical formats to complex functional or high-content cell assays (D).
discovery portfolios9 and this requires adaptable and flexible automation, with modular approaches offering significant advantages. Thus, a ‘plug and play’ reconfigurable system, with modules that are self‐sufficient in operation, has been installed at AstraZeneca (Figure 1). Each module performs a dedicated task, which can be combined to undertake a full assay protocol. Rapid reconfiguration of these modular platforms enables automation to meet varied screening demands, from simple mix‐and‐ measure biochemical formats to complex functional or high-content cell assays. Modular automation minimises equipment redundancy and provides the ability to multiplex different assays on the same screening platform, bringing further efficiencies. Automation allows screening operations to occur out‐of‐hours.
However, to realise this benefit in productivity, automation platforms must be robust and reliable, with minimal errors and operator intervention. The ability to automatically mitigate or recover errors through remote operation interfaces, such as smartphones or tablets, is also advantageous. In many ways the flexibility required in generating the breadth of different assay formats for HTS makes it quite unique. In common scientific processes, such as repetitive compound processing steps or in manufacturing plants, robotic systems are produced that simply repeat a common set of activities. Establishing these systems is far less complex, as reliability and process optimisation may be established from a single optimisation step, whereas HTS configurations may need to change on a robotic system four to five times a year and under constant time pressure.
The introduction of more collaborative robots, incorporating safety sensors and simple AI, enables ‘human‐friendly’ equipment that can be directly accessed by the scientist without the need for restrictive safety guarding. Thus, users can interact more closely with equipment, allowing better monitoring of equipment during screening activities and facilitating rapid recovery in the event of errors. The incorporation of simple and efficient user interfaces enables more intuitive interaction with complex automation systems.
In addition to the hardware infrastructure, scheduling software is critical in maximising assay performance and system reliability. Careful protocol design is key and minimising robot moves is important to increase efficiency and minimise ‘white space’ wherever possible. Implementing key decision points within screening protocols is beneficial; for example, in the event of an automation error, assigning “failed” microtitre plates to a quarantine location can allow other downstream labware to be progressed successfully through to assay completion. Use of barcoded labware enables tracking of the steps in the protocol and system scheduling ensures uniformity of all processes when handling large numbers of microtitre plates. Automated quality control can further increase assay robustness; for example, monitoring real-time liquid dispensing during screening can highlight any issues, allowing them to be resolved immediately ahead of biological results.
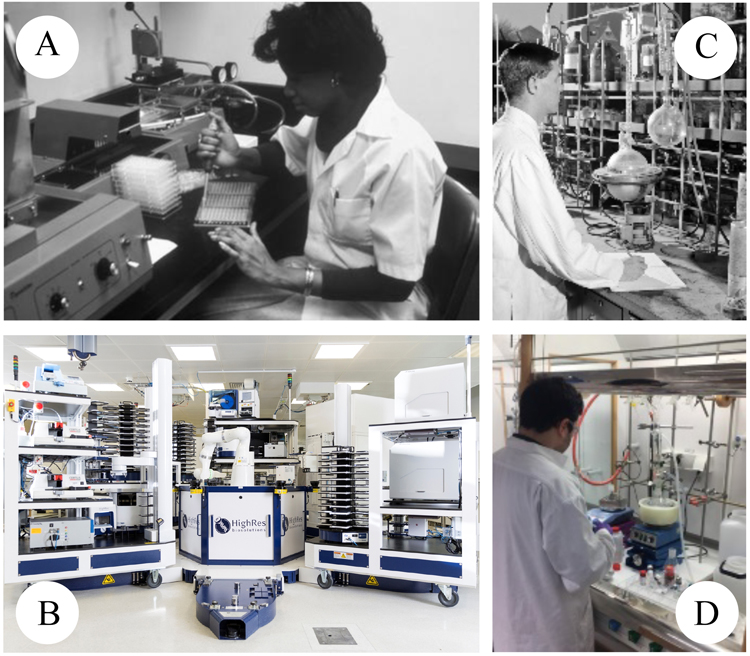
Figure 2: Biological screening has evolved from a manual process (A) to its highly automated and efficient form today (B). In contrast organic synthesis of designed compounds has remained markedly unchanged (C & D).
As described above, the drive to accelerate medicines through the early research pipeline has often centred on optimising the biology-focused components. Reagents, assays and small molecule screening have been repeatedly optimised over the past 30 years to generate well recognised standard practice,10 with the biological screening laboratory of today being unrecognisable from those of the past, through the adoption of automation, technology and IT (Figures 2A & B). The same has not been true for the chemistry-focused aspects11 (Figures 2C & D). Automation of chemistry has mainly evolved for screening library design. Fundamentally, the organic synthesis of drug-like molecules has remained mostly unchanged, being a highly manual process. As biology has been miniaturised, the quantity of compound required for assays has dramatically reduced, while the demand for structural diversity, enabling molecular evolution, has rapidly increased.
Design, make, test, analyse
It presently takes about 12 months from initiating a biological target, through assay development and screening to deliver potential chemical hits. This is followed by approximately 18 months of molecular evolution to progress these compounds into ‘drug‐ like’ leads (the design, make, test and analyse cycle “DMTA”) (Figure 3). Subsequent lead optimisation takes about another 12 months of continued chemical evolution to realise a candidate drug. Each synthesis round of the many DMTA cycles takes a chemist three to six weeks to manufacture, purify, quantify and qualify the required compound, ahead of a panel of biological assays to profile properties including potency, selectivity and toxicity.
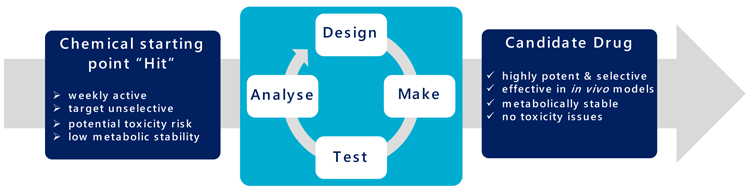
Figure 3: The DMTA cycle.
“Automation allows these large data volumes to be handled effectively and to ensure the maximum information and value is extracted” Drug Target Review | Volume 7, Issue 01, Spring 2020 Biological screening has evolved from a manual process (A) to its highly automated and efficient form today (B). In contrast organic synthesis of designed compounds has remained markedly unchanged (C & D). Representation of the cyclic process for compound design, make, test and analyse to evolve the structure of a chemical starting point into a candidate drug.
Automating chemical synthesis12-14 is an area of increasing interest, providing three parallel benefits. Firstly, the existing DMTA process has many physical handovers between the different chemistry disciplines. Each handover generates ‘white space’, resulting in lost time between individuals and locations. Full automation centralises these activities to reap time savings. Secondly, chemical transformations are often optimised to fit the human work pattern, with reactions occurring overnight, around meetings or over lunch. Once automated, the reaction can progress for the optimal period at any time of day, to provide true 24-hour processing. Finally, automated chemistry releases chemists from mundane synthesis, to allow focus on the challenging and complex chemistry that can only be performed manually.
While still in its infancy,15 automated chemical synthesis platforms integrated with automated compound purification and structure confirmation, as well as platforms for full automation of the DMTA cycle, will permit non-stop generation of structure-activity-relationship data.16 By consolidating and automating analytical and purification instruments for more effective use, better maintenance and instrument performance, quality will improve and the potential to bring synthesis times down from three to six weeks to three to 10 days will be realised.
Modular automation minimises equipment redundancy and provides the ability to multiplex different assays on the same screening platform, bringing further efficiencies”
Automating physical assays, whether in primary screening or as part of an automated DMTA cycle, enables the use of data-rich assay technologies such as imaging, including multiparametric assays like cell painting, flow cytometry and genomic approaches. This is leading to an exponential growth in both numerical and image-based data as genomics, transcriptomics, proteomics and metabolomics give rise to potentially thousands of results per screened compound. This growth in volume and complexity presents several challenges to data analysts. Automation allows these large data volumes to be handled effectively and to ensure the maximum information and value is extracted, while also accelerating the progress of projects to meet the productivity challenge. Data storage is the first challenge, and this is being tackled with the use of offline cloud‐based storage alongside on-premises repositories. Use of database technologies such as ObjectStore can enable the storage, retrieval and use of the vast amounts of current and future data. This facilitates the flow of data into AI protocols for downstream analysis.
An automated flow
The FAIR‐ification (FAIR – Findable, Accessible, Interoperable and Reusable)17 of data also plays a key role in understanding the purpose of an assay and how data generated from it can and should be used in combination with other datasets. This information will be vital in exploiting the undoubted power of machine and deep learning to extract context and meaning from the volume of data and metadata, the scale of which is unfathomable to the human scientist. Equally, data retention policies will be essential in maintaining data, ensuring that only required data is kept, to reduce the burden of ever-increasing data volumes.
As mentioned previously, productivity remains a key issue in the pharma industry. At AstraZeneca, screening data analysis software has the ability to read in data automatically both from lab instruments and compound/plate databases and to create analysis sessions with no user interaction, leaving the analyst to analyse the data without spending time on data input. Automation has facilitated significant improvement in the analysis of thousands of concentration response (CR) curves. An automated workflow now characterises key features of the curves, placing them into defined categories, saving the analyst many hours of time and removing subjectivity. Automated hit compound enrichment and near neighbour selection removes time lost by having serial steps carried out by separate groups, thereby fast‐tracking the flow of compounds through an HTS assay cascade. This delivers time savings that allow analysts to engage thoroughly in extracting the relevant information from the generated data. Data volumes and complexity will undoubtedly continue to grow, but by increasing automation of routine operations for data extraction and upload using sophisticated database technologies, and by expansion of AI methods for identifying and exploiting data trends, data analysis processes will remain efficient and central to effective decision‐making in drug discovery.
To deliver new medicines faster, the cycle time to key decisions must be shortened, while ensuring the correct information to make the right choices for new medicine progression is obtained. Automation applied to biological screening will improve the ability to generate large amounts of high-quality data during hit identification. Coupling this to an increasing ability to automate the design and synthesis of new compounds during the hit-to-lead and lead optimisation phases of drug discovery, will enable a more effective workflow for characterisation and progression of those initial hits. As alternative screening approaches are applied at ever increasing capacities (DNA encoded libraries, in silico screening), the ability to rapidly synthesise and follow up on far greater numbers of potential chemistries will become paramount. Automating analysis of data collected during the initial automated screening activity and during the automated DMTA cycle, will enable scientists across the early drug discovery value chain to focus less on repetitive tasks and to devote more time to the characterisation of those test compounds with the desired mechanisms of action required to deliver effective new medicines.
Further to this, there remain opportunities to target other areas of the drug discovery process: automation of DNA synthesis, vector construction, cell line generation and protein production are all areas that may allow further optimisation of biological process. Acceleration of these relatively routine yet critical and multifactorial steps, would help accelerate projects as well as enable efficient decisions on methodology applied to prosecute ever more challenging portfolios of novel targets.
About the authors
Having obtained his BSc in Applied Biology at the Manchester Metropolitan University, UK, Darren Plant has worked in the pharmaceutical industry for 30 years. He is an experienced drug- discovery bioscientist, working in the field of biochemical and cell high- throughput screening and laboratory automation. He has been employed within the AstraZeneca Global High-Throughput Screening Centre since inception, currently as a Senior Research Scientist, with a responsibility for delivering screening data to a global internal and external customer base.
Co authors:
Paul Harper, Geoff Holdgate, David Murray, Helen Plant, John Vincent and Mark Wigglesworth
Hit Discovery, Discovery Sciences, R&D, AstraZeneca, Alderley Park, UK
References
- DiMasi JA, Grabowski HG, Hansen RW. Innovation in the pharmaceutical industry: New estimates of R&D costs. Journal of Health Economics 2016; 47: 23-30.
- Paul SM, Mytelka DS, Dunwiddie CT, Persinger CC, Munos BH, Lindborg SR, Schacht AL. How to improve R&D productivity: the pharmaceutical industry’s grand challenge. Nat. Rev. Drug Discov.2010; 9: 203-214.
- Morgan P, Brown DG, Lennard S, et al. Impact of a five-dimensional framework on R&D productivity at AstraZeneca. Nat Rev Drug Discov. 2018; 17(3): 167-181.
- Brown DG, Bostrom J. Where do recent small molecule clinical development candidates come from? J Med Chem. 2018; 61(21): 9442-9468.
- Preston M, Murray D, Wigglesworth M. Open innovation – a collaboration between academia and the pharmaceutical industry to further leverage drug discovery expertise and assets. Drug Target Review. 2019; 6(4): 10-15.
- Butendeich H, Pierret NM, Numao S. Evaluation of a liquid dispenser for assay development and enzymology in 1536-well format. J Lab Autom. 2013; 18(3): 245-250.
- Knight S, Plant H, McWilliams L, Wigglesworth M. Techniques to enable 1536-well phenotypic screening. Methods Mol Biol. 2018; 1787: 263-278.
- Thomas-Fowlkes B, Cifelli S, Souza S, et al. Cell-based in vitro assay automation: Balancing technology and data reproducibility/predictability. SLAS Technol. 2020:2472630320902095. [Epub ahead of print]
- Clark R, Harper P, Wigglesworth MJ. Technological and sociological advances in HTS: evolution and revolution? European Pharmaceutical Review. 2013; 18(6): 55.
- Murray D, Wigglesworth M. HTS methods: Assay design and optimisation. In: Bittker JA, Ross NT, eds. High throughput screening methods: Evolution and refinement. The Royal Society of Chemistry; 2017: 1-15.
- Service, R. The synthesis machine. Science 2015; 347(6227): 1190-1193.
- Sutherland JD et al. An automated synthesis-purification-sample-management platform for the accelerated generation of pharmaceutical candidates. Lab. Autom. 2014; 2: 176-82.
- Bedard AC, Adamo A, Aroh KC, et al. Reconfigurable system for automated optimisation of diverse chemical reactions. Science. 2018; 361(6408): 1220-1225.
- Gioiello A, Piccinno A, Lozza AM, Cerra B. The medicinal chemistry in the era of machines and automation: Recent advances in continuous flow technology. J Med Chem. 2020. [Epub ahead of print]
- Peplow, M. Organic synthesis: The robo-chemist. Nature 2014; 512(7512): 20-22.
- Yang, B et al. Discovery of Potent KIFC1 Inhibitors Using a Method of Integrated High-Throughput Synthesis and Screening. Journal of Medicinal Chemistry 2014; 57(23): 9958-9970.
- Wilkinson MD et al. The FAIR Guiding Principles for scientific data management and stewardship. Sci Data. 2016; 3: 160018.
Related topics
Assays, Drug Discovery, Drug Discovery Processes, High-Content Assays, High-Throughput Screening (HTS), Lab Automation, Research & Development, Robotics, Screening, Technology
Related organisations
AstraZeneca