Translating novel drug success in animals to humans
Posted: 12 August 2020 | Victoria Rees (Drug Target Review) | 2 comments
A team has developed a predictive tool called TransComp-R which could be used to reveal whether new drugs that have been effective in animals will have positive outcomes in humans.
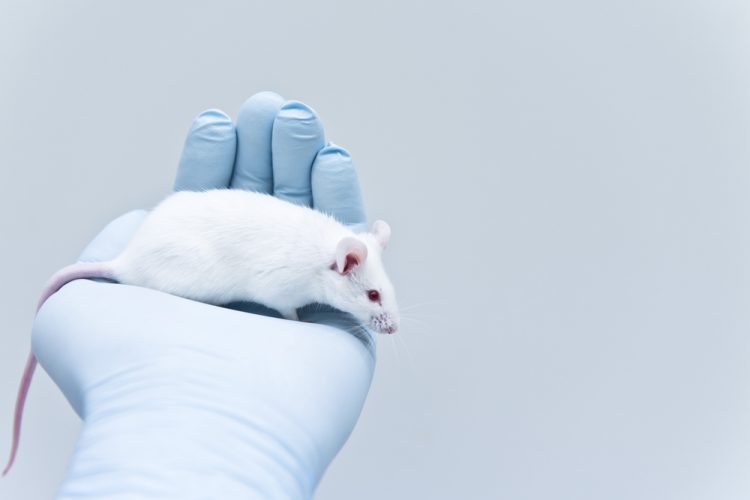
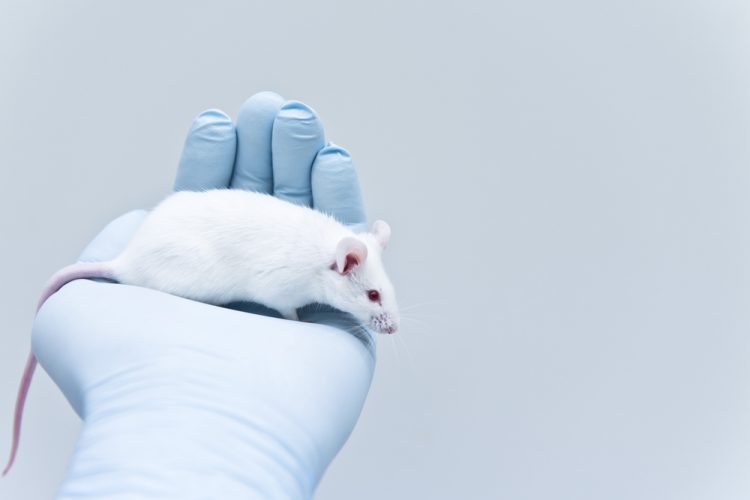
Although many drug compounds show efficacy and are well tolerated in animals, once they reach clinical studies, the situation can change. To prevent drugs that are successful in pre-clinical trials but ineffective in humans reaching the clinical stage, researchers have developed a computational method to identify the drugs that will not work.
The technology was developed by a team from Purdue University and Massachusetts Institute of Technology (MIT), both in the US. The results can be found in Science Signalling.
“This type of cross-species modelling could become a staple of drug development research by giving researchers an additional tool to interpret animal studies for translatable biological signatures. This may inform clinical testing and increase the likelihood of success when transitioning therapies from animal studies to humans,” commented Doug Brubaker, when speaking to Drug Target Review. “If there is a reason why the drug would fail, such as a resistance mechanism that was not obvious from the animal studies, then this model would also potentially detect that and help guide how a clinical trial should be set up.”
Developing the model
The researchers say the mechanisms that make a drug ineffective can be hard to identify during pre-clinical testing because animal models may have different biological processes driving disease or a response to therapy. This can make it difficult to translate observations from animal experiments to human biological contexts.
Calling their newly created model translatable components regression (TransComp-R), the researchers explain it works by consolidating thousands of measurements from an animal model to a few data coordinates for comparison with humans. The compressed data explain the most relevant sources of biological differences between the animal model and humans.
From there, the team suggest scientists can train other sets of models to predict a human’s response to therapy in terms of those data coordinates from an animal model. However, Brubaker commented that the conversion of animal data into human data was not a simple task.
“In terms of methods development, we had to find a way to make cross-species predictions when bimolecular data and phenotypes were discordant between species,” Brubaker said. “The main challenge was representing bimolecular differences in one species relative to the biomolecular differences in another given these discrepancies.”
Putting the model to the test
The researchers demonstrated that their model can successfully pinpoint when a drug may not work by using it to identify an overlooked biological mechanism possibly responsible for a patient’s resistance to infliximab.
The team say that about 50 percent of the patients who take infliximab for inflammatory bowel diseases, such as Crohn’s disease, can end up becoming resistant or unresponsive to it – highlighting a particular need for their new tool.
For infliximab, data from a mouse model and human had not matched because they were different types of biological measurements. The mouse model data came in the form of intestinal proteins, whereas data from patients were only available in the form of expressed genes. Despite this proteomics versus transcriptomics discrepancy, the team say TransComp-R can help to bridge the gap.
…the mechanisms that make a drug ineffective can be hard to identify during pre-clinical testing”
The model helped the researchers find links in the data pointing towards a resistance mechanism in humans. They collaborated with researchers from Vanderbilt University, US, to test the predicted mechanism in intestinal biopsies from a Crohn’s disease patient and then with experiments in human immune cells.
The researchers used single-cell sequencing of a sample from an infliximab-resistant Crohn’s disease patient to identify the cell types expressing the genes related to the resistance mechanism predicted by TransComp-R. They identified that the interactions between the integrin subunit alpha 1 (ITGA1) gene, which was expressed in T cells, and intestinal cell types were associated with resistance to infliximab.
Treating the immune cells with infliximab, the team were then able to show that inhibiting α1 integrin subunit signalling enhanced the ability of the drug to suppress proinflammatory cytokine release from immune cells. This enabled the drug to be more effective as it could better control inflammation.
With additional testing to figure out a way to more precisely measure the markers of this resistance mechanism, the team suggest doctors could use information about the drug response to determine if a patient needs a different course of treatment.
Future uses of the model
Since this study, Brubaker has been working with his former research group at MIT to apply the mathematical framework behind TransComp-R to identify mouse models predictive of a range of other diseases and conditions.
“We are applying the modelling framework in other biological contexts such as Alzheimer’s disease, cancer and COVID-19. Anytime you take a model into a new application area, you learn ways it can be improved such as including additional data types or modelling phenotypes other than binary drug response,” commented Brubaker. “Figuring out when what we see in animals does not track with what is happening in humans could save a lot of time, cost and effort in the drug development process overall.”
Related topics
Drug Development, Drug Leads, Informatics, Proteomics, Research & Development, Technology, Therapeutics
Related conditions
Covid-19, Crohn’s disease, Inflammatory bowel disease (IBD)
Related organisations
Massachusetts Institute of Technology (MIT), Purdue University, Vanderbilt University
Related people
Assistant Professor Doug Brubaker
Hi there, after reading this amazing paragraph i am also cheerful to share my
experience here with friends.
Wow, that’s what I was looking for, what a information! existing here at this weblog, thanks admin of this
website.