Machine learning models predict antibiotic resistance spread
Posted: 29 October 2021 | Anna Begley (Drug Target Review) | No comments yet
Scientists have used several machine learning models to predict bacterial gene exchange, which could reveal novel antibiotic targets.
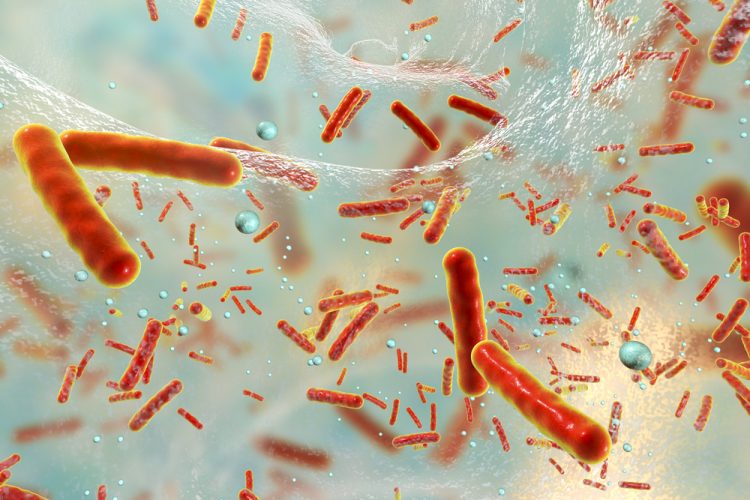
Researchers at Cornell University, US, have used machine learning to sort organisms by their functions and used this information to predict with near-perfect accuracy how genes are transferred between them, an approach that could lead to new targets to stop the spread of antibiotic resistance.
“Organisms basically can acquire resistance genes from other organisms. So, it would help if we knew which organisms bacteria were exchanging with and not only that, but we could figure out what are the driving factors that implicate organisms in this transfer,” said senior author Assistant Professor Ilana Brito. “If we can figure out who is exchanging genes with who, then maybe it would give insight into how this actually happens and possibly even control these processes.”
Many novel traits are shared through gene transfer, however scientists have not been able to determine why some bacteria engage in gene transfer while others do not. Instead of testing individual hypotheses, the team looked to bacteria genomes and their various functions to identify signatures that indicate which were swapping genes and what was driving these networks of exchange.
The team used several machine learning models, each of which teased out different phenomena embedded in the data, enabling them to identify multiple networks of different antibiotic resistance genes and across strains of the same organism.
From their study, published in Science Advances, the team found that the machine learning models were particularly effective when applied to antibiotic resistance genes. “I think one of the big takeaways here is that the network of bacterial gene exchange – specifically for antibiotic resistance – is predictable,” Brito stated. “We can understand it by looking at the data and we can do better if we actually look at each organism’s genome. It is not a random process.”
One of the most surprising findings was that the modelling predicted many possible antibiotic resistance transfers between human-associated bacteria and pathogens that have not yet been observed. These transfer events were almost exclusive to human-associated bacteria in the gut microbiome or oral microbiome.
NEWS: Antibiotic-free hydrogel fights against drug-resistant bacteria – READ HERE
“One can imagine that if we can predict how these genes spread, we might be able to either intervene or choose a specific antibiotic, depending what we see in a patient’s gut,” Brito said. “More broadly, we may see where certain types of organisms are predicted to transfer with others in a certain environment. We think there might be novel antibiotic targets in the data. For example, genes that could cripple these organisms, potentially, in terms of their ability to persist in certain environments or acquire these genes.”
Related topics
Antibiotics, Drug Targets, Genetic Analysis, Genomics, Informatics, Microbiology, Microbiome, Small Molecules, Technology
Related organisations
Cornell University
Related people
Ilana Brito