Discovering and designing drugs with artificial intelligence
Posted: 4 March 2020 | Victoria Rees (Drug Target Review) | No comments yet
The first drug designed using artificial intelligence (AI) has moved into its Phase I trial. Professor Andrew Hopkins of Exscientia explains how an algorithm was used to achieve this milestone.
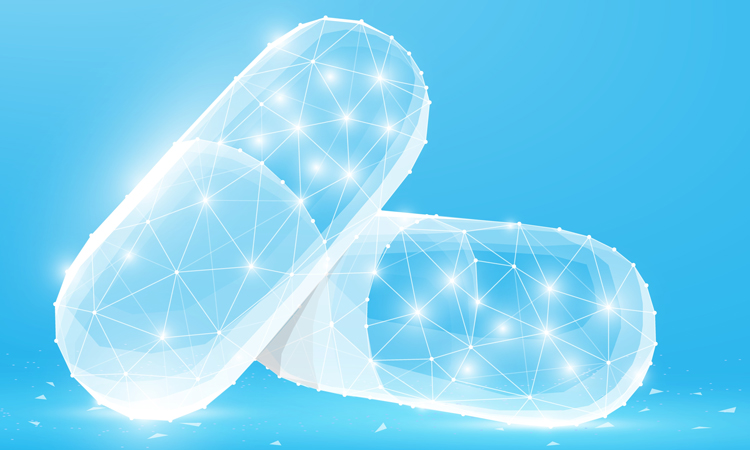
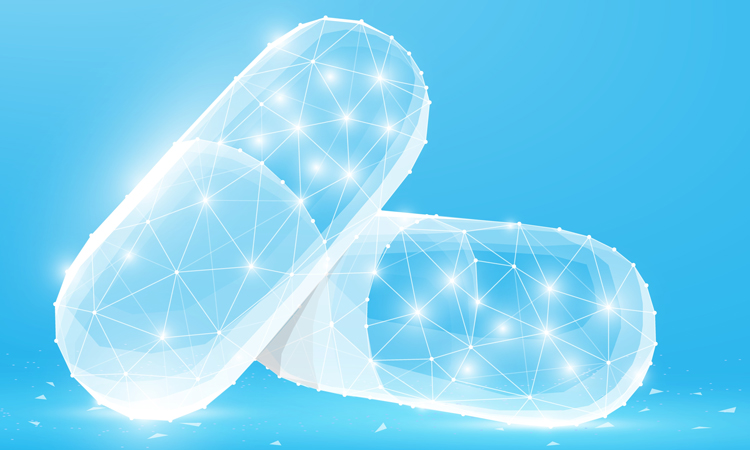
In a landmark development, the first drug created using artificial intelligence (AI) has moved into its Phase I trial. Named DSP-1181, the compound was created in a joint venture between Exscientia and Sumitomo Dainippon Pharma for the treatment of obsessive-compulsive disorder (OCD).
Speaking with Drug Target Review’s Victoria Rees, Professor Andrew Hopkins, Chief Executive Officer of Exscientia, explained how the drug was discovered and optimised in only 12 months.
The drug’s mechanism of action
Hopkins began by explaining that the drug works as a full agonist of potential activity for the serotonin 5-HT1A receptor, unlike other existing serotonin 5-HT1A receptor agonists which only partially block activity. It also has a much longer half-life than other drugs. This has led him and other researchers to believe it will demonstrate a much stronger efficacy and longer duration of action than current therapies.
The agent in the drug manipulates the neural circuits related to OCD, which suggests an onset of efficacy faster than the standard treatments, according to Hopkins.
Using AI in drug design
The researchers defined a strategy and objective for the AI central chemist system utilised in their study, known as Centaur ChemistTM. This allowed optimisation of an algorithm to identify new chemical matter with a desired molecular profile.
Utilising AI to search a chemical space, which includes potentially billions of options in terms of atom configuration, enabled the researchers to reduce the time taken to identify their target. Integrating data-generating hypotheses with machine learning to produce drug design concepts, the steps previously undertaken by humans were replaced with a suite of advanced algorithms.
Firstly, the AI generated millions of potential novel molecules that fit certain specifications. Then, a machine learning platform was used to predict which compounds would be active against hundreds and thousands of proteins. A third tier of algorithms, called active learning, were then applied, which automatically prioritised which compounds the researchers should make and test.
These processes enabled the scientists to precisely engineer the drug, by satisfying a large number of design objectives simultaneously using AI.
“This is why this is such an important breakthrough, because it’s showing how AI can be used to create the molecule and therefore challenges the traditional process,” said Hopkins, “the beauty of the algorithm is that every atom counts.”
Issues in R&D
…the future may see a closer relationship between technologists and drug discovery scientists”
A key problem in drug design identified by Hopkins is that only a small amount of data is readily available. This, he said, is the “opposite of a big data problem,” meaning that different algorithms are required to learn.
He explained that there is a wealth of biological and chemical data sources, which can be integrated and used to build machine learning models. However, for most proteins in the human body, there is often very little information when initiating a drug discovery project. Furthermore, for each individual target there is generally a small data or sparse data problem. Algorithmically, the challenge is therefore how to learn quickly from very little data.
Hopkins said that to solve this, a set of algorithms developed in the field of active learning is essential for designing innovative medicines.
“What we are starting to see by making better design decisions, is that we can synthesise fewer compounds and this leads to a project progressing far more rapidly than is conventionally the case,” he explained.
Significance of using AI
A major benefit of using AI that Hopkins highlighted was the speed at which the pre-clinical phase moved. He explained that it allowed the optimisation and identification of the clinical candidate much faster than traditional methods.
A major problem facing the pharmaceutical industry, he said, is productivity. Poor return rates on research could be solved using AI. “If we can reduce the cost of investment, ultimately we can start to reduce the barriers of translating new insights from academia and the clinic into new medicines.”
However, the “real significance” that Hopkins discussed was that DSP-1181 is a new chemical entity; the molecule is not a repurposed drug. This, he explained, will challenge the way that conventional drug discovery is conducted.
Using a combination of human strategy and creativity in synchronisation with the advantages of algorithms could therefore present a plethora of new tactics and problem solving for drug discovery, which has the potential to increase productivity.
Power through collaboration
Hopkins admitted that an initial complication was to accumulate and integrate the data with the AI, which was a critical step.
This is a broader issue facing the drug discovery industry, Hopkins commented, which all technologists need to be aware of, as “it is not just about designing algorithms with improved efficiency. One of the key things is how technologists interact with drug discoverers and actually understand the data that is coming from the assays.”
Having a successful dialogue between various experts is therefore essential for a deeper understanding of scientific results.
Shaping the future
By the end of the decade, Hopkins expects that all drugs entering into human clinical trials will have been designed with AI. This automation will not only be used in drug design and discovery, but drug development, he said.
AI was used from the beginning of the drug discovery process, including for initial hits”
“We believe the advantages are so powerful that we will start to see a wide adoption amongst the pharmaceutical industry in using these techniques for drug discovery because there will be such a clear economic advantage to do so.”
He predicts that the future may potentially see a closer relationship between technologists and drug discovery scientists that will fuse into a new culture of “pharma-tech.”
As companies undergo constant innovations and the technology for AI and machine learning changes year on year, it is important for experts in technology and drugs discovery to work together, he explained.
“I believe this milestone has demonstrated that AI can directly contribute to the creation of new medicines.”
Related topics
Artificial Intelligence, Drug Development, Drug Discovery, Drug Discovery Processes, Drug Leads, Drug Targets, Informatics, Research & Development, Technology
Related conditions
obsessive-compulsive disorder (OCD)
Related organisations
Exscientia, Sumitomo Dainippon Pharma
Related people
Professor Andrew Hopkins