Why some screening hits are a PAIN
Posted: 22 June 2021 | Dr Matthew Lloyd (University of Bath) | No comments yet
A major challenge during high-throughput and fragment screening is the potential for identifying ‘frequent hitters’ – compounds that affect unrelated targets. Matthew Lloyd from the University of Bath explains why these hits can arise during drug discovery and how machine learning could be the answer to identifying these compounds.
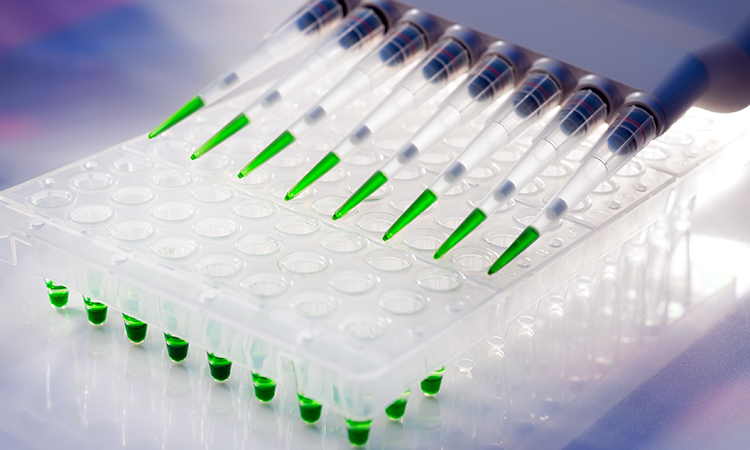
High-throughput screening (HTS) is a common method used in drug discovery (Figure 1). In a typical campaign, an isolated protein is incubated with a diverse library of compounds, drug-like molecules, natural products or other potential drugs and their ability to modify a protein function (such as enzymatic activity) is measured. Screening libraries typically contain many thousands or even millions of compounds. An advantage of utilising HTS is that it can be used to identify novel chemical scaffolds that mediate their effects by several different pharmacological mechanisms.1 A related approach is fragment screening, in which a smaller library of lower molecular weight compounds (typically <300 Da.) are screened by biophysical methods such as surface plasmon resonance (SPR), isothermal calorimetry (ITC) and X-ray crystallography to identify fragments which bind to the target.2 Such fragments typically bind very weakly, but they can be optimised into highly potent and selective compounds.2 These screening approaches are widely recognised as valuable drug discovery strategies.
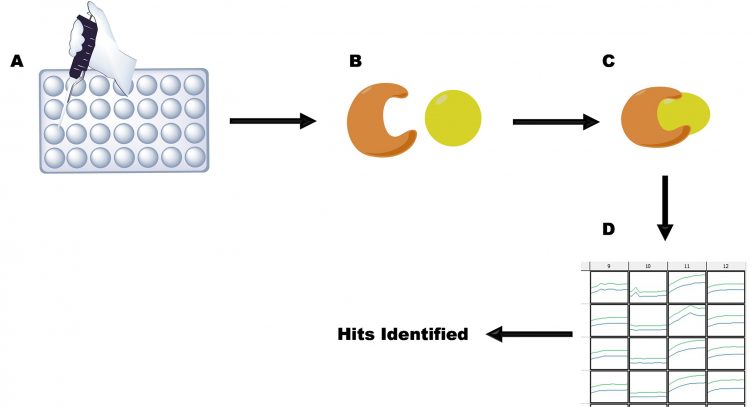
Figure 1: The principles of HTS, as illustrated by an enzyme target using a continuous assay. A. Reagents are pipetted into a microtitre plate; B. Enzyme and drug are present in solution; C. Drug binds to enzyme; D. Substrate is added and activity is measured (columns 9, 11 and 12). Binding of drug is measured by loss of activity (column 10), allowing hits to be identified.
However, a significant problem in HTS and fragment screening is that many ‘hits’ (compounds which appear to have the desired effect) turn out to be false positives or ‘frequent hitters’ – compounds that exert their effects on many unrelated protein (enzyme) drug targets.3 There are several mechanisms by which frequent hitting can arise.3,4 Firstly, some inhibitors are just promiscuous and can bind to many different targets;4,5 indeed, this is very typical of hits identified in fragment screens.2 Similarly, many electrophilic molecules can covalently modify many unrelated targets6 and this is generally seen as undesirable due to potential toxic side effects. In many cases, selectivity and potency can be increased by modification of the drug during the hit-to-lead development phase2 or the tuning of electrophilicity7 in the case of covalent inhibitors. Secondly, potential drug molecules can inhibit their targets by mechanisms that are not ‘pharmacologically tractable’. There are several different mechanisms by which this behaviour can arise, including interference with the assay,3,4 generation of reactive oxygen species8,9 or formation of aggregates.10-12 Such compounds are often referred to as Pan‑Assay Inhibitors (PAINs),2,10,11 Bad Actors or nuisance compounds13 and are a significant problem. In addition, inhibition can also arise due to compound contamination with transition metals.1 Frequent hitters have been shown to be markedly heavier, with more hydrogen‑bonding donors and acceptors, rotatable bonds and a higher number of ‘violations’ of drug‑like properties than ‘well‑behaved compounds’, but only to have marginal differences in LogP.3 Another study also showed no correlation between frequent hitter status and LogP.6
A significant problem in HTS and fragment screening is that many ‘hits’ (compounds which appear to have the desired effect) turn out to be false positives or ‘frequent hitters’”
Many PAINs can be eliminated using confirmatory assays immediately following HTS. These will typically involve repeating assays under the same conditions with positive and negative controls, ideally using freshly-acquired compounds and proteins/enzymes. These assays also eliminate compounds that have been mislabelled, have undergone chemical or biological degradation or where activity arises due to the presence of contaminating metal ions. Assays based on fluorescence are commonly used in HTS1 but are prone to false positive results due to quenching of the product fluorophore. These effects can be minimised by using a fluorophore with a long excitation wavelength14 and a confirmatory assay based on a different technology – eg, high‑performance liquid chromatography (HPLC). Generation of reactive oxygen species can be determined using fluorometric or nuclear magnetic resonance (NMR)-based assays,8,9 of which NMR assays9 appear to provide the most reliable results.
A particularly common and well-investigated mechanism by which PAIN activity can arise is through the formation of compound aggregates (Figure 2).1,2,10-12 Many compounds have limited solubility in assay conditions, even in the presence of cosolvents such as dimethyl sulfoxide (DMSO), which presents a significant problem in HTS1,10-12 and fragment screening.2 The exact mechanism by which aggregates cause inhibition has not been determined, but it appears to arise from enzyme adherence to the aggregate, limiting interaction with the substrate.1 HTS is routinely conducted in the presence of detergents, eg, 0.01 percent Triton X-100,1,11,12 as this will reduce the tendency of compounds to aggregate – several other detergents have also been successfully used.1 Other indicators that inhibition is due to compound aggregation include direct observation of aggregates – for example, by dynamic laser light scattering; diminished inhibition in the presence of higher detergent concentrations, eg, 0.1 percent Triton X-100; or non-competitive inhibition of enzyme activity (which is uncommon and can only arise under very specific circumstances).1
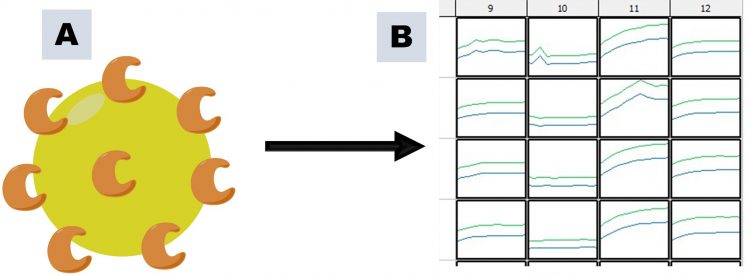
Figure 2: PAINs activity arising through compound aggregation, as illustrated using an enzyme target and a continuous assay. A. Large aggregates are formed, to which enzyme adheres; B. Diffusion of enzyme becomes rate-limiting, resulting in apparent inhibition of enzymatic activity (column 10) compared to other columns.
In view of the problematic nature of PAINs that aggregate, there have been several attempts to remove these compounds from HTS libraries. Many of these attempts necessitate the identification of sub-structure elements that predispose compounds to aggregate.10,11 However, PAIN behaviour such as aggregation is actually observed relatively infrequently1,4,6 and is highly dependent on assay conditions (buffer, pH, ionic strength, compound concentration, etc).1 A consequence of this is that viable hits may be unnecessarily eliminated from compound collections.11
Many PAINs can be eliminated using confirmatory assays immediately following HTS”
In view of this, several groups have attempted to use machine learning or similar techniques to identify problematic compounds.4-6 David et al. used machine learning to identify compounds that interfere with the assay,4 while Matlock et al.6 used a similar approach to identify promiscuous electrophilic compounds. However, there appears to be only one general screen13 for different types of frequent hitters, including aggregating compounds that are a common reason for this behaviour.1,12 Machine learning and similar approaches appear to provide better predictions of frequent hitter behaviour, although it is still early days. Nevertheless, these approaches offer promise as they appear to be able to predict frequent hitter behaviour more accurately,13 especially when combined with traditional algorithms used to predict PAINs.6
Acknowledgement
The author has been funded by Prostate Cancer UK (grants S10-03 and PG14-009), The University of Bath Overseas Research Studentship, The Biochemical Society Summer Vacation Studentship Scheme, the Bath-Shandong University exchange programme and LifeArc. The author is a member of the Cancer Research @ Bath network.
About the author
Dr Matthew Lloyd read Biological Chemistry at the University of Leicester, followed by a DPhil (PhD) degree at the University of Oxford, working on antibiotics and finishing in 1992. After several years of working as a post-doctoral researcher at Brown University, US and Oxford, Matthew joined the Department of Pharmacy & Pharmacology at the University of Bath in 2002. He is currently a Senior Lecturer (Associate Professor) in the department. His research focuses on enzymes using techniques from Chemistry, Biochemistry and Molecular Biology to understand disease states and develop new treatments, especially for cancer.
References
- Lloyd M. J. Med. Chem., 2020, 63, 10742-10772.
- Keserű G, Erlanson D, Ferenczy G, et al. J. Med. Chem., 2016, 59, 8189-8206.
- Böcker A, Bonneau P, Edwards P. J. Biomol. Screening, 2011, 16, 765-774.
- David L, Walsh J, Sturm N, et al. ChemMedChem, 2019, 14, 1795-1802.
- Goodwin S, Shahtahmassebi G, Hanley Q. Sci. Rep., 2020, 10.
- Matlock M, Hughes T, Dahlin J, Swamidass S. J. Chem. Inform. Model., 2018, 58, 1483-1500.
- Hermann M, Pautsch A, Grundl M, et al. J. Comput.-Aided Mol. Des., 2021, 35, 531–539.
- Johnston P, Soares K, Shinde S, et al. Assay Drug Dev. Technol., 2008, 6, 505-518.
- Tarnowski M, Barozet B, Johansson C, et al. Assay Drug Dev. Technol., 2018, 16, 177-191.
- Baell J, Holloway G. J. Med. Chem., 2010, 53, 2719-2740.
- Baell J, Nissink J. ACS Chem. Biol., 2018, 13, 36-44.
- Jadhav A, Ferreira R, Klumpp C, et al. J. Med. Chem., 2010, 53, 37-51.
- Stork C, Chen Y, Sicho M, Kirchmair J. J. Chem. Inform. Model., 2019, 59, 1030-1043.
- Simeonov A, Jadhav A, Thomas C, et al. J. Med. Chem., 2008, 51, 2363-2371.
Related topics
Assays, Drug Discovery, High-Throughput Screening (HTS), Nuclear Magnetic Resonance (NMR), Screening, Small Molecules, X-ray Crystallography
Related organisations
Beckman Coulter, Horizon Discovery, Merck, X-Chem
Related people
Laurianne David, Matthew Matlock