New ‘smart’ cell therapies show promise in solid tumours
Posted: 27 November 2020 | Hannah Balfour (Drug Target Review) | No comments yet
By combining machine learning and T-cell engineering researchers were able to develop cell therapies that can selectively and effectively target and destroy solid tumours.
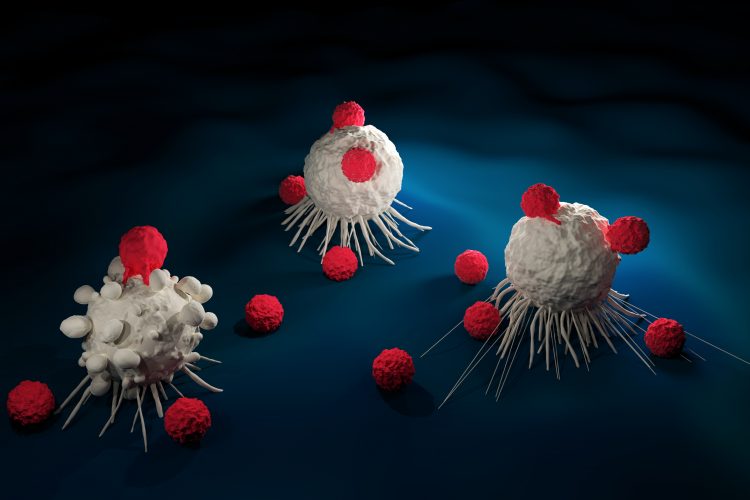
Researchers working to identify anti-cancer therapeutics that can selectively target tumour cells without damaging normal cells have used machine learning and therapeutic cell engineering to develop ‘smart’ cell therapies for solid tumours.
Gathering and validating the data
The research is split across two papers, in the first – published in Cell Systems – researchers in the lab of Dr Wendell Lim at the University Of California – San Francisco (UCSF) Cell Design Initiative and Center for Synthetic Immunology, both US, teamed up with a group of computer scientists led by Dr Olga Troyanskaya at Princeton University’s Lewis-Sigler Institute for Integrative Genomics and the Simons Foundation’s Flatiron Institute.
The scientists used computational approaches to examine the gene expression profile of more than 2,300 genes in normal and tumour cells to see what antigens could help discriminate between diseased and healthy cells. They then used machine learning techniques to come up with antigen combinations and determined if they could significantly improve how T cells recognise tumours while ignoring normal tissue.
Following the identification of antigen combinations on the computer, they engineered T cells to target them using a system known as synNotch. synNotch is a customisable molecular sensor that allows synthetic biologists to fine-tune the programming of cells and was developed in the Lim lab in 2016. According to the team it is a receptor that can be engineered to recognise a myriad of target antigens; additionally the response of synNotch to a target antigen can also be programmed, meaning the therapeutic T cell can execute any of a range of responses once an antigen is recognised.
They specifically used synNotch to programme T cells to destroy kidney cancer cells that express a unique combination of antigens called CD70 and AXL. Although CD70 is also found in healthy immune cells, and AXL in healthy lung cells, the engineered T cells killed only the cancer cells and spared the healthy cells.
“The field of big data analysis of cancer and the field of cell engineering have both exploded in the last few years, but these advances have not been brought together,” said Troyanskaya. “The computing capabilities of therapeutic cells combined with machine learning approaches enable actionable use of the increasingly available rich genomic and proteomic data on cancers.”
Developing further therapies
In the second paper, published in Science, Lim and colleagues used their computationally derived protein data to design effective and highly selective cell therapies for cancer.
“Currently, most cancer treatments, including cell therapies, are told ‘block this’ or ‘kill this,'” said Lim, also professor and chair of cellular and molecular pharmacology and a member of the UCSF Helen Diller Family Comprehensive Cancer Center. “We want to increase the nuance and sophistication of the decisions that a therapeutic cell makes.”
The researchers highlighted the example of chimeric antigen receptor (CAR) T cells. In CAR T-cell therapy, T cells are collected from a patient and manipulated in vitro to express a receptor that will recognise a specific antigen on cancer cells. However, despite their success in haematological malignancies like leukaemia and lymphoma, so far, the method has not been as effective in solid tumours. Cells in these cancers often share antigens with normal cells found in other tissues and they also create an immunosuppressive microenvironment; both limit the efficacy of CAR T cells.
Lim said that since solid tumours are more complex than blood cancers, a more complicated product is required to combat them.
In their paper, researchers daisy-chained multiple synNotch receptors together to create a host of complex cancer recognition circuits. The team said that since synNotch can activate the expression of selected genes in a “plug and play” manner, these components can be linked in different ways to create circuits with diverse functions, allowing for precise recognition of diseased cells and a range of responses when those cells are identified.
“This work is essentially a cell engineering manual that provides us with blueprints for how to build different classes of therapeutic T cells that could recognise almost any possible type of combinatorial antigen pattern that could exist on a cancer cell,” noted Lim.
The team gives two examples of how this can function:
- Engineer the T cells so that when a synNotch receptor recognises antigen A, the cell makes a second synNotch receptor that recognises antigen B. Interaction with antigen B could then induce the expression of a CAR that recognises antigen C. The result is a T cell that requires the presence of all three antigens to trigger killing.
- Alternatively, if the T cell encounters an antigen present in normal tissues but not in the cancer, a synNotch receptor programmed with a NOT function could be programmed to cause the T cell carrying it to die, sparing the normal cells from attack and preventing toxic effects.
In the paper the team uses these configurations to selectively kill cells carrying different combinatorial markers of melanoma and breast cancer. They also showed in a murine model of two similar tumours with different antigen combinations, that the T cells efficiently and precisely located and killed the tumour they had been engineered to detect.
Lim’s group is now exploring how these circuits could be used in CAR T cells to treat glioblastoma.
Related topics
Bioengineering, Biologics, Disease Research, Drug Development, Drug Targets, Immuno-oncology, Immunotherapy, Protein, Proteomics, Screening, Therapeutics
Related conditions
Breast cancer, Glioblastoma, Kidney Cancer
Related organisations
Princeton University, University of California San Francisco (UCSF)
Related people
Dr Olga Troyanskaya, Dr Wendell Lim