How many drug discovery breakthroughs have we missed?
Posted: 14 December 2022 | Dr Aaron Daugherty | No comments yet
Today’s drug screening methods use one or two types of data. However, disease biology is not replicable by simple screening models because diseases are complex and heterogenous. However, advanced screening methods that process dozens of data sources at one time have uncovered novel hits that have been overlooked across the entire history of medicine. In this article, Dr Aaron Daugherty, Vice President of Discovery at Aria Pharmaceuticals, shares evidence of how we might be missing potential breakthrough therapies.
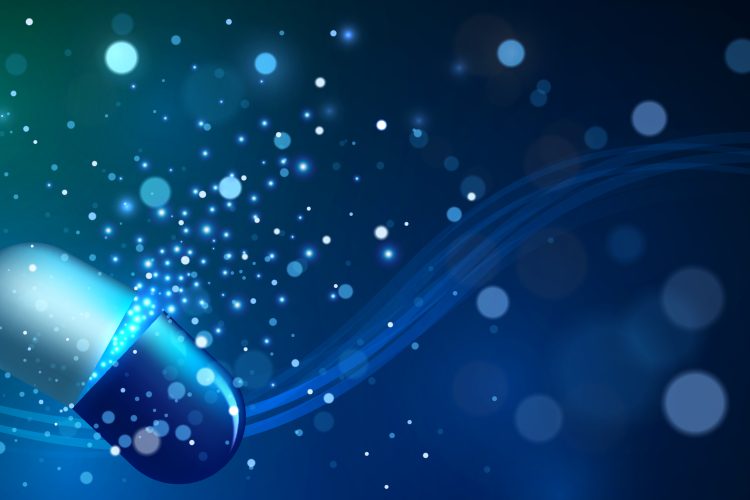
The traditional drug discovery and development process is ripe for innovation at almost every point and drug screening is no exception. Traditionally, screening – the process of identifying hits to treat a disease – is carried out in a lab via a single experiment that represents the key disease-modifying hypothesis. For example, interrogating the activity of a single protein target or the modification of a single phenotype. This is usually a physical and time-consuming task that is limited by human ability to perform experiments and analyse results.
I believe it is safe to say that with these traditional lab-based approaches, we have missed potential breakthroughs. Not because we do not understand the science, but because we are time and resource limited, which can result in a process that is not optimised for scientific robustness, but for speed and efficiency. For years, technology has provided solutions for time and efficiency constraints. Robotic automation is commonplace in high-throughput screening (HTS) and more recently, virtual drug screening, where in silico models simulate a biological process, have been used to screen for effective hits. Utilising technologies such as these to screen for drugs does greatly improve speed and efficiency and the addition of modern advances in technologies like artificial intelligence (AI), carry the potential to increase speed and efficiency even further.
While the use of technology, including AI-assisted screening, is already used to accelerate a traditional screening approach, it has its limits. That is because traditional screening necessarily uses a reductionist approach, wherein a disease hypothesis is reduced to a single dimension (eg, a target or phenotype) to feasibly screen against it. This, by definition, limits insight into the potential of molecules to treat a disease. Using this approach, even with today’s best technology, the result will not substantially change. AI-assisted screening can provide a faster, more efficient process to screen compounds, but we will still only discover a few hits with viable efficacy because we remain siloed within a reduced view of disease.
Are we trying to solve the right problem?
I would argue that inventing solutions to further accelerate a traditional approach is throwing technology towards the wrong solution. Instead, we need to re-examine how technology can allow for a redesign of our approach.
In my view, we need screening methods that allow for the interrogation of the multi-dimensional nature of complex disease pathology. As our knowledge of disease increases, so does the complexity of the problems we need to solve. It therefore makes sense that we also update how we screen for treatments.
Multiple, simultaneous orthogonal screens could offer new insights into the disease-fighting potential of new treatments by interrogating complex diseases in distinct ways. In fact, in an ideal world, we would simultaneously examine dozens of different data modalities to best understand the complex biological interactions of a disease. This is where the true power of technology – especially AI – shines. The human mind is highly capable when analysing data in two or three dimensions, but beyond that, AI can dramatically increase these abilities. The power to simultaneously examine a possible treatment from dozens of different perspectives has the potential to entirely change how we screen for drugs.
Early scientific work has demonstrated that such an approach can lead to new discoveries and by not using it more widely, we are missing potential breakthrough therapies every day. I am fortunate enough to be part of a team that uses technology not only to screen faster, but to rethink how we screen for drug hits in a disease. Rather than using technology to screen compounds against a single disease-relevant target or phenotype, we have created an approach that integrates and simultaneously analyses completely unconnected multimodal data in a single process, breaking the data silos so often faced in traditional drug screening.
The results are not surprising. Using this approach we find that molecules and mechanisms of action (MOA) that don’t show up in a single data modality become evident when analysed against multiple data types simultaneously.
A new approach in action
Our team built an in silico model of systemic lupus erythematosus (SLE) using dozens of different data sources encompassing exclusively human-derived biology, chemistry, molecular, pharmacology and clinical data. We then used that model to screen a purposefully chosen, high-quality library of more than 50,000 molecules representing a wide diversity of chemical structures and mechanisms. This led to the identification of a few thousand molecules predicted to be efficacious for SLE. Within that list of compounds, we rediscovered all the approved and late-stage therapies for SLE, as well as hundreds of molecules with similar mechanisms of action (MOAs). More interestingly, however; we also found a set of 80 unique molecules with novel and safe MOAs. From there, our scientists used our interpretable AI model to conduct a deep dive into these molecules, further narrowing down to nine hits. Along the way, we also verified that all nine hits were supported by multiple unconnected data modalities, underlining the importance of using a multimodal data approach to screening.
To our knowledge, no previous work has led to these nine molecules or their MOAs being clinically tested against or patented for use in SLE. We next took these molecules directly to in vivo pre-clinical studies, which ultimately led to the identification of a lead molecule, as well as a backup, each with unique and novel MOAs. This novel AI-enabled screening approach was completed in a matter of months, versus the years that it would usually take using traditional methods. More importantly, this new approach enabled us to identify and test multiple novel MOAs. This broadened our understanding of SLE disease biology and maximised our chances of finding a disease-modifying hit.
These results showcase that one-dimensional (ie, traditional) screening approaches are, in fact, missing potential breakthroughs in the very early stages of drug discovery and screening. They also illustrate how approaching the problem from a different perspective and applying technology towards the root of the problem, can produce different results. We have seen similar outcomes across other unrelated therapeutic areas, including chronic kidney disease (CKD) and idiopathic pulmonary fibrosis (IPF), to name a few. We of course still have a long way to go with these programmes to demonstrate their potential breakthrough impact for patients. However, we are already fortunate enough to discover, not one, but multiple potential treatments to bring closer to the clinic, each of which would have otherwise been missed using traditional methods. That, to me, represents tangible progress.
In addition to identifying otherwise unappreciated MOAs, a multimodal data approach to drug screening brings other benefits. Firstly, we are not reliant on any single type of data. This is very helpful when working in many unrelated therapeutic areas, but also because when a new data type is developed, it can readily add to and improve an existing approach, instead of trying to replace it. Such iterative improvement leads to massive gains over time. Secondly, because multimodal in silico models are more comprehensive and capture the complex interactions of a disease, it allows us to better understand and predict clinical efficacy and safety. Using an approach like this, we are not only being more efficient, but are also maximising our chances of success.
Screening is an essential part of early drug discovery and it always will be, but it can pose a barrier to progress if we use screening methods with limited scope. It is only natural that as we learn more about diseases and their vast complexities, we must update drug screening. The pharmaceutical industry has been and should be known for its high level of innovation. To maintain that reputation, we need to improve on today’s limits – whether that means utilising the approaches I have outlined or inventing even better ones. By doing so, we will turn from facing a declining return from research and development to an increase in both productivity and return.
As drug discovery researchers, we will continue to miss breakthroughs; that is the nature of the work we do. However, to give us the best chance of finding more breakthroughs, we must be willing to rethink not just what technology we use, but how we use it. By rethinking our approaches in the context of today’s technology we can find more breakthrough medicines for the patients who need them.
Dr Aaron Daugherty
Aaron is Vice President of Discovery at Aria Pharmaceuticals and one of its first employees. He has helped build Aria’s drug discovery platform and leads the Discovery Science team’s efforts to discover potential treatments across a wide range of diseases. Aaron earned his PhD in Genetics from Stanford University. Prior to his time at Stanford, Aaron was a Fulbright Scholar and received his Bachelor of Science in Biology from the University of Richmond.
Related topics
Artificial Intelligence, Drug Development, Drug Discovery, Drug Discovery Processes, Screening, Technology, Therapeutics
Related organisations
aria pharmaceuticals
Related people
Dr Aaron Daughtery (Aria Pharmaceuticals)